Name | AutoRAG JSON |
Version |
0.3.13
JSON |
| download |
home_page | None |
Summary | Automatically Evaluate RAG pipelines with your own data. Find optimal structure for new RAG product. |
upload_time | 2025-01-25 05:28:38 |
maintainer | None |
docs_url | None |
author | None |
requires_python | >=3.10 |
license | Apache License
Version 2.0, January 2004
http://www.apache.org/licenses/
TERMS AND CONDITIONS FOR USE, REPRODUCTION, AND DISTRIBUTION
1. Definitions.
"License" shall mean the terms and conditions for use, reproduction,
and distribution as defined by Sections 1 through 9 of this document.
"Licensor" shall mean the copyright owner or entity authorized by
the copyright owner that is granting the License.
"Legal Entity" shall mean the union of the acting entity and all
other entities that control, are controlled by, or are under common
control with that entity. For the purposes of this definition,
"control" means (i) the power, direct or indirect, to cause the
direction or management of such entity, whether by contract or
otherwise, or (ii) ownership of fifty percent (50%) or more of the
outstanding shares, or (iii) beneficial ownership of such entity.
"You" (or "Your") shall mean an individual or Legal Entity
exercising permissions granted by this License.
"Source" form shall mean the preferred form for making modifications,
including but not limited to software source code, documentation
source, and configuration files.
"Object" form shall mean any form resulting from mechanical
transformation or translation of a Source form, including but
not limited to compiled object code, generated documentation,
and conversions to other media types.
"Work" shall mean the work of authorship, whether in Source or
Object form, made available under the License, as indicated by a
copyright notice that is included in or attached to the work
(an example is provided in the Appendix below).
"Derivative Works" shall mean any work, whether in Source or Object
form, that is based on (or derived from) the Work and for which the
editorial revisions, annotations, elaborations, or other modifications
represent, as a whole, an original work of authorship. For the purposes
of this License, Derivative Works shall not include works that remain
separable from, or merely link (or bind by name) to the interfaces of,
the Work and Derivative Works thereof.
"Contribution" shall mean any work of authorship, including
the original version of the Work and any modifications or additions
to that Work or Derivative Works thereof, that is intentionally
submitted to Licensor for inclusion in the Work by the copyright owner
or by an individual or Legal Entity authorized to submit on behalf of
the copyright owner. For the purposes of this definition, "submitted"
means any form of electronic, verbal, or written communication sent
to the Licensor or its representatives, including but not limited to
communication on electronic mailing lists, source code control systems,
and issue tracking systems that are managed by, or on behalf of, the
Licensor for the purpose of discussing and improving the Work, but
excluding communication that is conspicuously marked or otherwise
designated in writing by the copyright owner as "Not a Contribution."
"Contributor" shall mean Licensor and any individual or Legal Entity
on behalf of whom a Contribution has been received by Licensor and
subsequently incorporated within the Work.
2. Grant of Copyright License. Subject to the terms and conditions of
this License, each Contributor hereby grants to You a perpetual,
worldwide, non-exclusive, no-charge, royalty-free, irrevocable
copyright license to reproduce, prepare Derivative Works of,
publicly display, publicly perform, sublicense, and distribute the
Work and such Derivative Works in Source or Object form.
3. Grant of Patent License. Subject to the terms and conditions of
this License, each Contributor hereby grants to You a perpetual,
worldwide, non-exclusive, no-charge, royalty-free, irrevocable
(except as stated in this section) patent license to make, have made,
use, offer to sell, sell, import, and otherwise transfer the Work,
where such license applies only to those patent claims licensable
by such Contributor that are necessarily infringed by their
Contribution(s) alone or by combination of their Contribution(s)
with the Work to which such Contribution(s) was submitted. If You
institute patent litigation against any entity (including a
cross-claim or counterclaim in a lawsuit) alleging that the Work
or a Contribution incorporated within the Work constitutes direct
or contributory patent infringement, then any patent licenses
granted to You under this License for that Work shall terminate
as of the date such litigation is filed.
4. Redistribution. You may reproduce and distribute copies of the
Work or Derivative Works thereof in any medium, with or without
modifications, and in Source or Object form, provided that You
meet the following conditions:
(a) You must give any other recipients of the Work or
Derivative Works a copy of this License; and
(b) You must cause any modified files to carry prominent notices
stating that You changed the files; and
(c) You must retain, in the Source form of any Derivative Works
that You distribute, all copyright, patent, trademark, and
attribution notices from the Source form of the Work,
excluding those notices that do not pertain to any part of
the Derivative Works; and
(d) If the Work includes a "NOTICE" text file as part of its
distribution, then any Derivative Works that You distribute must
include a readable copy of the attribution notices contained
within such NOTICE file, excluding those notices that do not
pertain to any part of the Derivative Works, in at least one
of the following places: within a NOTICE text file distributed
as part of the Derivative Works; within the Source form or
documentation, if provided along with the Derivative Works; or,
within a display generated by the Derivative Works, if and
wherever such third-party notices normally appear. The contents
of the NOTICE file are for informational purposes only and
do not modify the License. You may add Your own attribution
notices within Derivative Works that You distribute, alongside
or as an addendum to the NOTICE text from the Work, provided
that such additional attribution notices cannot be construed
as modifying the License.
You may add Your own copyright statement to Your modifications and
may provide additional or different license terms and conditions
for use, reproduction, or distribution of Your modifications, or
for any such Derivative Works as a whole, provided Your use,
reproduction, and distribution of the Work otherwise complies with
the conditions stated in this License.
5. Submission of Contributions. Unless You explicitly state otherwise,
any Contribution intentionally submitted for inclusion in the Work
by You to the Licensor shall be under the terms and conditions of
this License, without any additional terms or conditions.
Notwithstanding the above, nothing herein shall supersede or modify
the terms of any separate license agreement you may have executed
with Licensor regarding such Contributions.
6. Trademarks. This License does not grant permission to use the trade
names, trademarks, service marks, or product names of the Licensor,
except as required for reasonable and customary use in describing the
origin of the Work and reproducing the content of the NOTICE file.
7. Disclaimer of Warranty. Unless required by applicable law or
agreed to in writing, Licensor provides the Work (and each
Contributor provides its Contributions) on an "AS IS" BASIS,
WITHOUT WARRANTIES OR CONDITIONS OF ANY KIND, either express or
implied, including, without limitation, any warranties or conditions
of TITLE, NON-INFRINGEMENT, MERCHANTABILITY, or FITNESS FOR A
PARTICULAR PURPOSE. You are solely responsible for determining the
appropriateness of using or redistributing the Work and assume any
risks associated with Your exercise of permissions under this License.
8. Limitation of Liability. In no event and under no legal theory,
whether in tort (including negligence), contract, or otherwise,
unless required by applicable law (such as deliberate and grossly
negligent acts) or agreed to in writing, shall any Contributor be
liable to You for damages, including any direct, indirect, special,
incidental, or consequential damages of any character arising as a
result of this License or out of the use or inability to use the
Work (including but not limited to damages for loss of goodwill,
work stoppage, computer failure or malfunction, or any and all
other commercial damages or losses), even if such Contributor
has been advised of the possibility of such damages.
9. Accepting Warranty or Additional Liability. While redistributing
the Work or Derivative Works thereof, You may choose to offer,
and charge a fee for, acceptance of support, warranty, indemnity,
or other liability obligations and/or rights consistent with this
License. However, in accepting such obligations, You may act only
on Your own behalf and on Your sole responsibility, not on behalf
of any other Contributor, and only if You agree to indemnify,
defend, and hold each Contributor harmless for any liability
incurred by, or claims asserted against, such Contributor by reason
of your accepting any such warranty or additional liability.
END OF TERMS AND CONDITIONS
APPENDIX: How to apply the Apache License to your work.
To apply the Apache License to your work, attach the following
boilerplate notice, with the fields enclosed by brackets "[]"
replaced with your own identifying information. (Don't include
the brackets!) The text should be enclosed in the appropriate
comment syntax for the file format. We also recommend that a
file or class name and description of purpose be included on the
same "printed page" as the copyright notice for easier
identification within third-party archives.
Copyright [yyyy] [name of copyright owner]
Licensed under the Apache License, Version 2.0 (the "License");
you may not use this file except in compliance with the License.
You may obtain a copy of the License at
http://www.apache.org/licenses/LICENSE-2.0
Unless required by applicable law or agreed to in writing, software
distributed under the License is distributed on an "AS IS" BASIS,
WITHOUT WARRANTIES OR CONDITIONS OF ANY KIND, either express or implied.
See the License for the specific language governing permissions and
limitations under the License.
|
keywords |
rag
autorag
autorag
rag-evaluation
evaluation
rag-auto
automl
automl-rag
|
VCS |
 |
bugtrack_url |
|
requirements |
pydantic
numpy
pandas
tqdm
tiktoken
openai
rank_bm25
pyyaml
pyarrow
fastparquet
sacrebleu
evaluate
rouge_score
rich
click
cohere
tokenlog
aiohttp
voyageai
mixedbread-ai
llama-index-llms-bedrock
scikit-learn
emoji
pymilvus
chromadb
weaviate-client
pinecone
couchbase
qdrant-client
quart
pyngrok
llama-index
llama-index-core
llama-index-readers-file
llama-index-embeddings-openai
llama-index-embeddings-ollama
llama-index-llms-openai
llama-index-llms-openai-like
llama-index-retrievers-bm25
streamlit
gradio
langchain-core
langchain-unstructured
langchain-upstage
langchain-community
panel
seaborn
ipykernel
ipywidgets
ipywidgets_bokeh
|
Travis-CI |
No Travis.
|
coveralls test coverage |
No coveralls.
|
# AutoRAG
RAG AutoML tool for automatically finding an optimal RAG pipeline for your data.

 
[](https://www.linkedin.com/company/104375108/admin/dashboard/)

[](https://huggingface.co/AutoRAG)
[](https://github.com/orgs/Auto-RAG/projects/1/views/2)
<img src=https://github.com/user-attachments/assets/9a4d0381-a161-457f-a787-e7eb3593ce00 width="251.5" height="55.2"/>
There are many RAG pipelines and modules out there,
but you don’t know what pipeline is great for “your own data” and "your own use-case."
Making and evaluating all RAG modules is very time-consuming and hard to do.
But without it, you will never know which RAG pipeline is the best for your own use-case.
AutoRAG is a tool for finding the optimal RAG pipeline for “your data.”
You can evaluate various RAG modules automatically with your own evaluation data
and find the best RAG pipeline for your own use-case.
AutoRAG supports a simple way to evaluate many RAG module combinations.
Try now and find the best RAG pipeline for your own use-case.
Explore our 📖 [Document](https://docs.auto-rag.com)!!
Plus, join our 📞 [Discord](https://discord.gg/P4DYXfmSAs) Community.
---
Do you have any difficulties in optimizing your RAG pipeline?
Or is it hard to set up things to use AutoRAG?
Try [**AutoRAG Cloud**](https://tally.so/r/n0jOrZ) beta.
We will help you to run AutoRAG and optimize.
Plus, we can help you to build RAG evaluation dataset.
Starts with 9.99$ per optimization.
---
## YouTube Tutorial
https://github.com/Marker-Inc-Korea/AutoRAG/assets/96727832/c0d23896-40c0-479f-a17b-aa2ec3183a26
_Muted by default, enable sound for voice-over_
You can see on [YouTube](https://youtu.be/2ojK8xjyXAU?feature=shared)
## Use AutoRAG in HuggingFace Space 🚀
- [💬 Naive RAG Chatbot](https://huggingface.co/spaces/AutoRAG/Naive-RAG-chatbot)
- [✏️ AutoRAG Data Creation](https://huggingface.co/spaces/AutoRAG/AutoRAG-data-creation)
- [🚀 AutoRAG RAG Pipeline Optimization](https://huggingface.co/spaces/AutoRAG/AutoRAG-optimization)
## Colab Tutorial
- [Step 1: Basic of AutoRAG | Optimizing your RAG pipeline](https://colab.research.google.com/drive/19OEQXO_pHN6gnn2WdfPd4hjnS-4GurVd?usp=sharing)
- [Step 2: Data Creation | Create your own Data for RAG Optimization](https://colab.research.google.com/drive/1BOdzMndYgMY_iqhwKcCCS7ezHbZ4Oz5X?usp=sharing)
- [Step 3: Use Custom LLM & Embedding Model | Use Custom Model](https://colab.research.google.com/drive/12VpWcSTSOsLSyW0BKb-kPoEzK22ACxvS?usp=sharing)
# Index
- [Quick Install](#quick-install)
- [Data Creation](#data-creation)
- [Parsing](#1-parsing)
- [Chunking](#2-chunking)
- [QA Creation](#3-qa-creation)
- [RAG Optimization](#rag-optimization)
- [How AutoRAG optimizes RAG pipeline?](#how-autorag-optimizes-rag-pipeline)
- [Metrics](#metrics)
- [Quick Start](#quick-start-1)
- [Set YAML File](#1-set-yaml-file)
- [Run AutoRAG](#2-run-autorag)
- [Run Dashboard](#3-run-dashboard)
- [Deploy your optimal RAG pipeline](#4-deploy-your-optimal-rag-pipeline)
- [🐳 AutoRAG Docker Guide](#-autorag-docker-guide)
- [FaQ](#-faq)
# Quick Install
We recommend using Python version 3.10 or higher for AutoRAG.
```bash
pip install AutoRAG
```
If you want to use the local models, you need to install gpu version.
```bash
pip install "AutoRAG[gpu]"
```
Or for parsing, you can use the parsing version.
```bash
pip install "AutoRAG[gpu,parse]"
```
# Data Creation
<a href="https://huggingface.co/spaces/AutoRAG/AutoRAG-data-creation">
<img src="https://github.com/user-attachments/assets/8c6e4b02-3938-4560-b817-c95764965b50" alt="Hugging Face Sticker" style="width:200px;height:auto;">
</a>


RAG Optimization requires two types of data: QA dataset and Corpus dataset.
1. **QA** dataset file (qa.parquet)
2. **Corpus** dataset file (corpus.parquet)
**QA** dataset is important for accurate and reliable evaluation and optimization.
**Corpus** dataset is critical to the performance of RAGs.
This is because RAG uses the corpus to retrieve documents and generate answers using it.
### 📌 Supporting Data Creation Modules

- [Supporting Parsing Modules List](https://edai.notion.site/Supporting-Parsing-Modules-e0b7579c7c0e4fb2963e408eeccddd75?pvs=4)
- [Supporting Chunking Modules List](https://edai.notion.site/Supporting-Chunk-Modules-8db803dba2ec4cd0a8789659106e86a3?pvs=4)
## Quick Start
### 1. Parsing
#### Set YAML File
```yaml
modules:
- module_type: langchain_parse
parse_method: pdfminer
```
You can also use multiple Parse modules at once.
However, in this case, you'll need to return a new process for each parsed result.
#### Start Parsing
You can parse your raw documents with just a few lines of code.
```python
from autorag.parser import Parser
parser = Parser(data_path_glob="your/data/path/*")
parser.start_parsing("your/path/to/parse_config.yaml")
```
### 2. Chunking
#### Set YAML File
```yaml
modules:
- module_type: llama_index_chunk
chunk_method: Token
chunk_size: 1024
chunk_overlap: 24
add_file_name: en
```
You can also use multiple Chunk modules at once.
In this case, you need to use one corpus to create QA and then map the rest of the corpus to QA Data.
If the chunk method is different, the retrieval_gt will be different, so we need to remap it to the QA dataset.
#### Start Chunking
You can chunk your parsed results with just a few lines of code.
```python
from autorag.chunker import Chunker
chunker = Chunker.from_parquet(parsed_data_path="your/parsed/data/path")
chunker.start_chunking("your/path/to/chunk_config.yaml")
```
### 3. QA Creation
You can create QA dataset with just a few lines of code.
```python
import pandas as pd
from llama_index.llms.openai import OpenAI
from autorag.data.qa.filter.dontknow import dontknow_filter_rule_based
from autorag.data.qa.generation_gt.llama_index_gen_gt import (
make_basic_gen_gt,
make_concise_gen_gt,
)
from autorag.data.qa.schema import Raw, Corpus
from autorag.data.qa.query.llama_gen_query import factoid_query_gen
from autorag.data.qa.sample import random_single_hop
llm = OpenAI()
raw_df = pd.read_parquet("your/path/to/parsed.parquet")
raw_instance = Raw(raw_df)
corpus_df = pd.read_parquet("your/path/to/corpus.parquet")
corpus_instance = Corpus(corpus_df, raw_instance)
initial_qa = (
corpus_instance.sample(random_single_hop, n=3)
.map(
lambda df: df.reset_index(drop=True),
)
.make_retrieval_gt_contents()
.batch_apply(
factoid_query_gen, # query generation
llm=llm,
)
.batch_apply(
make_basic_gen_gt, # answer generation (basic)
llm=llm,
)
.batch_apply(
make_concise_gen_gt, # answer generation (concise)
llm=llm,
)
.filter(
dontknow_filter_rule_based, # filter don't know
lang="en",
)
)
initial_qa.to_parquet('./qa.parquet', './corpus.parquet')
```
# RAG Optimization
<a href="https://huggingface.co/spaces/AutoRAG/RAG-Pipeline-Optimization">
<img src="https://github.com/user-attachments/assets/8c6e4b02-3938-4560-b817-c95764965b50" alt="Hugging Face Sticker" style="width:200px;height:auto;">
</a>


## How AutoRAG optimizes RAG pipeline?
Here is the AutoRAG RAG Structure that only show Nodes.

Here is the image showing all the nodes and modules.


### 📌 Supporting RAG Optimization Nodes & modules
- [Supporting RAG Modules list](https://edai.notion.site/Supporting-Nodes-modules-0ebc7810649f4e41aead472a92976be4?pvs=4)
## Metrics
The metrics used by each node in AutoRAG are shown below.


- [Supporting metrics list](https://edai.notion.site/Supporting-metrics-867d71caefd7401c9264dd91ba406043?pvs=4)
Here is the detailed information about the metrics that AutoRAG supports.
- [Retrieval Metrics](https://edai.notion.site/Retrieval-Metrics-dde3d9fa1d9547cdb8b31b94060d21e7?pvs=4)
- [Retrieval Token Metrics](https://edai.notion.site/Retrieval-Token-Metrics-c3e2d83358e04510a34b80429ebb543f?pvs=4)
- [Generation Metrics](https://github.com/user-attachments/assets/7d4a3069-9186-4854-885d-ca0f7bcc17e8)
## Quick Start
### 1. Set YAML File
First, you need to set the config YAML file for your RAG optimization.
We highly recommend using pre-made config YAML files for starter.
- [Get Sample YAML](./sample_config/rag)
- [Sample YAML Guide](https://docs.auto-rag.com/optimization/sample_config.html)
- [Make Custom YAML Guide](https://docs.auto-rag.com/optimization/custom_config.html)
Here is an example of the config YAML file to use `retrieval`, `prompt_maker`, and `generator` nodes.
```yaml
node_lines:
- node_line_name: retrieve_node_line # Set Node Line (Arbitrary Name)
nodes:
- node_type: retrieval # Set Retrieval Node
strategy:
metrics: [retrieval_f1, retrieval_recall, retrieval_ndcg, retrieval_mrr] # Set Retrieval Metrics
top_k: 3
modules:
- module_type: vectordb
vectordb: default
- module_type: bm25
- module_type: hybrid_rrf
weight_range: (4,80)
- node_line_name: post_retrieve_node_line # Set Node Line (Arbitrary Name)
nodes:
- node_type: prompt_maker # Set Prompt Maker Node
strategy:
metrics: # Set Generation Metrics
- metric_name: meteor
- metric_name: rouge
- metric_name: sem_score
embedding_model: openai
modules:
- module_type: fstring
prompt: "Read the passages and answer the given question. \n Question: {query} \n Passage: {retrieved_contents} \n Answer : "
- node_type: generator # Set Generator Node
strategy:
metrics: # Set Generation Metrics
- metric_name: meteor
- metric_name: rouge
- metric_name: sem_score
embedding_model: openai
modules:
- module_type: openai_llm
llm: gpt-4o-mini
batch: 16
```
### 2. Run AutoRAG
You can evaluate your RAG pipeline with just a few lines of code.
```python
from autorag.evaluator import Evaluator
evaluator = Evaluator(qa_data_path='your/path/to/qa.parquet', corpus_data_path='your/path/to/corpus.parquet')
evaluator.start_trial('your/path/to/config.yaml')
```
or you can use the command line interface
```bash
autorag evaluate --config your/path/to/default_config.yaml --qa_data_path your/path/to/qa.parquet --corpus_data_path your/path/to/corpus.parquet
```
Once it is done, you can see several files and folders created in your current directory.
At the trial folder named to numbers (like 0),
you can check `summary.csv` file that summarizes the evaluation results and the best RAG pipeline for your data.
For more details, you can check out how the folder structure looks like
at [here](https://docs.auto-rag.com/optimization/folder_structure.html).
### 3. Run Dashboard
You can run a dashboard to easily see the result.
```bash
autorag dashboard --trial_dir /your/path/to/trial_dir
```
#### sample dashboard

### 4. Deploy your optimal RAG pipeline
### 4-1. Run as a Code
You can use an optimal RAG pipeline right away from the trial folder.
The trial folder is the directory used in the running dashboard. (like 0, 1, 2, ...)
```python
from autorag.deploy import Runner
runner = Runner.from_trial_folder('/your/path/to/trial_dir')
runner.run('your question')
```
### 4-2. Run as an API server
You can run this pipeline as an API server.
Check out the API endpoint at [here](./docs/source/deploy/api_endpoint.md).
```python
import nest_asyncio
from autorag.deploy import ApiRunner
nest_asyncio.apply()
runner = ApiRunner.from_trial_folder('/your/path/to/trial_dir')
runner.run_api_server()
```
```bash
autorag run_api --trial_dir your/path/to/trial_dir --host 0.0.0.0 --port 8000
```
The cli command uses extracted config YAML file. If you want to know it more, check out [here](https://docs.auto-rag.com/tutorial.html#extract-pipeline-and-evaluate-test-dataset).
### 4-3. Run as a Web Interface
you can run this pipeline as a web interface.
Check out the web interface at [here](deploy/web.md).
```bash
autorag run_web --trial_path your/path/to/trial_path
```
#### sample web interface
<img width="1491" alt="web_interface" src="https://github.com/Marker-Inc-Korea/AutoRAG/assets/96727832/f6b00353-f6bb-4d8f-8740-1c264c0acbb8">
### Use advanced web interface
You can deploy the advanced web interface featured by [Kotaemon](https://github.com/Cinnamon/kotaemon) to the fly.io.
Go [here](https://github.com/vkehfdl1/AutoRAG-web-kotaemon) to use it and deploy to the fly.io.
Example :
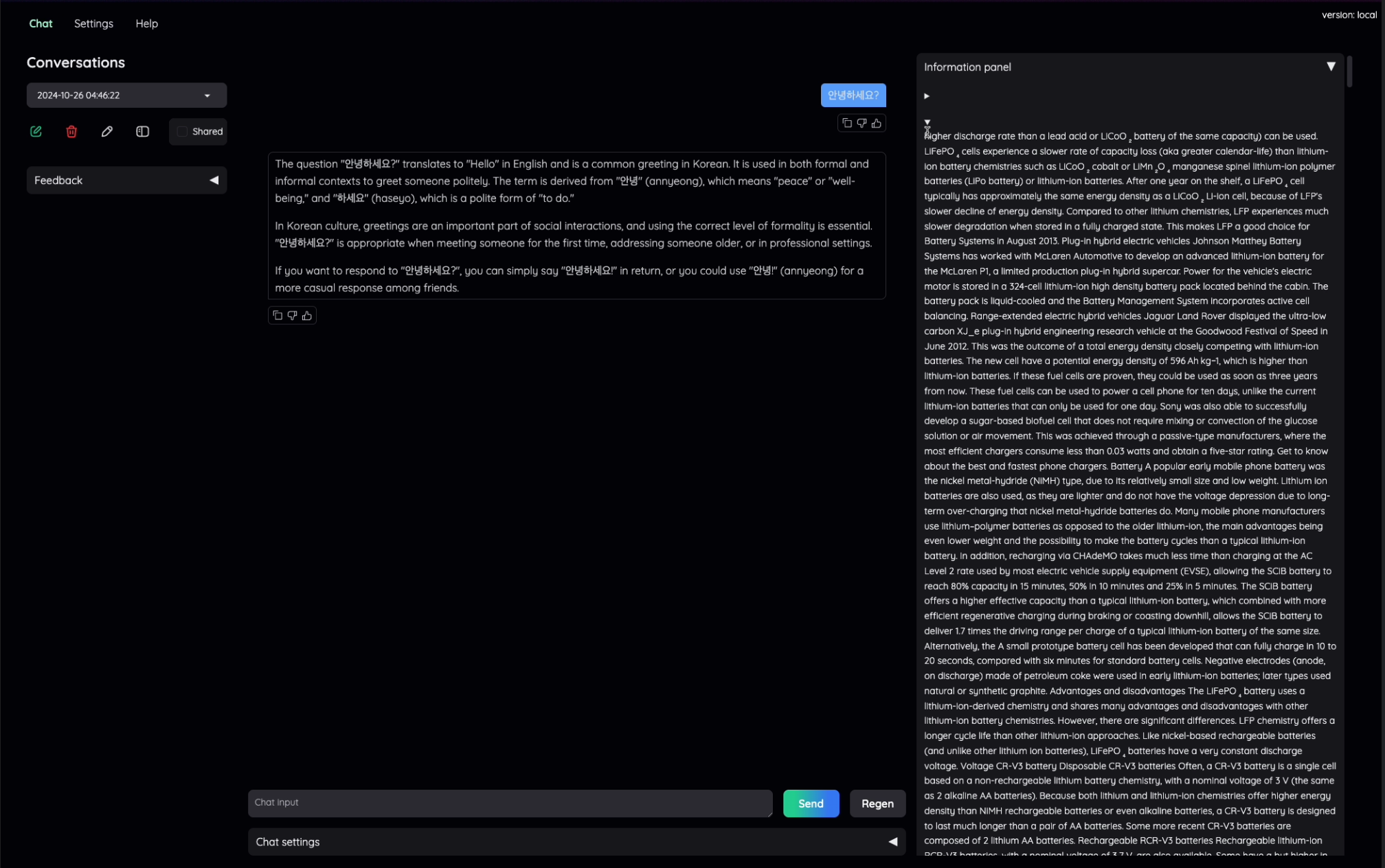
## 🐳 AutoRAG Docker Guide
This guide provides a quick overview of building and running the AutoRAG Docker container for production, with instructions on setting up the environment for evaluation using your configuration and data paths.
### 🚀 Building the Docker Image
Tip: If you want to build an image for a gpu version, you can use `autoraghq/autorag:gpu` or `autoraghq/autorag:gpu-parsing`
#### 1.Download dataset for [Tutorial Step 1](https://colab.research.google.com/drive/19OEQXO_pHN6gnn2WdfPd4hjnS-4GurVd?usp=sharing)
```bash
python sample_dataset/eli5/load_eli5_dataset.py --save_path projects/tutorial_1
```
#### 2. Run `evaluate`
> **Note**: This step may take a long time to complete and involves OpenAI API calls, which may cost approximately $0.30.
```bash
docker run --rm -it \
-v ~/.cache/huggingface:/root/.cache/huggingface \
-v $(pwd)/projects:/usr/src/app/projects \
-e OPENAI_API_KEY=${OPENAI_API_KEY} \
autoraghq/autorag:api-latest evaluate \
--config /usr/src/app/projects/tutorial_1/config.yaml \
--qa_data_path /usr/src/app/projects/tutorial_1/qa_test.parquet \
--corpus_data_path /usr/src/app/projects/tutorial_1/corpus.parquet \
--project_dir /usr/src/app/projects/tutorial_1/
```
#### 3. Run validate
```bash
docker run --rm -it \
-v ~/.cache/huggingface:/root/.cache/huggingface \
-v $(pwd)/projects:/usr/src/app/projects \
-e OPENAI_API_KEY=${OPENAI_API_KEY} \
autoraghq/autorag:api-latest validate \
--config /usr/src/app/projects/tutorial_1/config.yaml \
--qa_data_path /usr/src/app/projects/tutorial_1/qa_test.parquet \
--corpus_data_path /usr/src/app/projects/tutorial_1/corpus.parquet
```
#### 4. Run `dashboard`
```bash
docker run --rm -it \
-v ~/.cache/huggingface:/root/.cache/huggingface \
-v $(pwd)/projects:/usr/src/app/projects \
-e OPENAI_API_KEY=${OPENAI_API_KEY} \
-p 8502:8502 \
autoraghq/autorag:api-latest dashboard \
--trial_dir /usr/src/app/projects/tutorial_1/0
```
#### 4. Run `run_web`
```bash
docker run --rm -it \
-v ~/.cache/huggingface:/root/.cache/huggingface \
-v $(pwd)/projects:/usr/src/app/projects \
-e OPENAI_API_KEY=${OPENAI_API_KEY} \
-p 8501:8501 \
autoraghq/autorag:api-latest run_web --trial_path ./projects/tutorial_1/0
```
#### Key Points :
- **`-v ~/.cache/huggingface:/cache/huggingface`**: Mounts the host machine’s Hugging Face cache to `/cache/huggingface` in the container, enabling access to pre-downloaded models.
- **`-e OPENAI_API_KEY: ${OPENAI_API_KEY}`**: Passes the `OPENAI_API_KEY` from your host environment.
For more detailed instructions, refer to the [Docker Installation Guide](./docs/source/install.md#1-build-the-docker-image).
## ☎️ FaQ
🛣️ [Roadmap](https://github.com/orgs/Auto-RAG/projects/1/views/2)
💻 [Hardware Specs](https://edai.notion.site/Hardware-specs-28cefcf2a26246ffadc91e2f3dc3d61c?pvs=4)
⭐ [Running AutoRAG](https://edai.notion.site/About-running-AutoRAG-44a8058307af42068fc218a073ee480b?pvs=4)
🍯 [Tips/Tricks](https://edai.notion.site/Tips-Tricks-10708a0e36ff461cb8a5d4fb3279ff15?pvs=4)
☎️ [TroubleShooting](https://medium.com/@autorag/autorag-troubleshooting-5cf872b100e3)
## Thanks for shoutout
### Company
<a href="https://www.linkedin.com/posts/llamaindex_rag-pipelines-have-a-lot-of-hyperparameters-activity-7182053546593247232-HFMN/">
<img src="https://github.com/user-attachments/assets/b8fdaaf6-543a-4019-8dbe-44191a5269b9" alt="llama index" style="width:200px;height:auto;">
</a>
### Individual
- [Shubham Saboo](https://www.linkedin.com/posts/shubhamsaboo_just-found-the-solution-to-the-biggest-rag-activity-7255404464054939648-ISQ8/)
- [Kalyan KS](https://www.linkedin.com/posts/kalyanksnlp_rag-autorag-llms-activity-7258677155574788097-NgS0/)
## 💬 Talk with Founders
Talk with us! We are always open to talk with you.
- 🎤 [Talk with Jeffrey](https://zcal.co/autorag-jeffrey/autorag-demo-15min)
- 🦜 [Talk with Bwook](https://zcal.co/i/tcuLtmq5)
---
# ✨ Contributors ✨
Thanks go to these wonderful people:
<a href="https://github.com/Marker-Inc-Korea/AutoRAG/graphs/contributors">
<img src="https://contrib.rocks/image?repo=Marker-Inc-Korea/AutoRAG" />
</a>
# Contribution
We are developing AutoRAG as open-source.
So this project welcomes contributions and suggestions. Feel free to contribute to this project.
Plus, check out our detailed documentation at [here](https://docs.auto-rag.com/index.html).
## Citation
```bibtex
@misc{kim2024autoragautomatedframeworkoptimization,
title={AutoRAG: Automated Framework for optimization of Retrieval Augmented Generation Pipeline},
author={Dongkyu Kim and Byoungwook Kim and Donggeon Han and Matouš Eibich},
year={2024},
eprint={2410.20878},
archivePrefix={arXiv},
primaryClass={cs.CL},
url={https://arxiv.org/abs/2410.20878},
}
```
Raw data
{
"_id": null,
"home_page": null,
"name": "AutoRAG",
"maintainer": null,
"docs_url": null,
"requires_python": ">=3.10",
"maintainer_email": null,
"keywords": "RAG, AutoRAG, autorag, rag-evaluation, evaluation, rag-auto, AutoML, AutoML-RAG",
"author": null,
"author_email": "Marker-Inc <vkehfdl1@gmail.com>",
"download_url": "https://files.pythonhosted.org/packages/69/d0/bf645c26c194e8abc5d331f816b63f72cf784a3329c0ff4080ce51ed0979/autorag-0.3.13.tar.gz",
"platform": null,
"description": "# AutoRAG\n\nRAG AutoML tool for automatically finding an optimal RAG pipeline for your data.\n\n\n\n \n[](https://www.linkedin.com/company/104375108/admin/dashboard/)\n\n[](https://huggingface.co/AutoRAG)\n[](https://github.com/orgs/Auto-RAG/projects/1/views/2)\n\n<img src=https://github.com/user-attachments/assets/9a4d0381-a161-457f-a787-e7eb3593ce00 width=\"251.5\" height=\"55.2\"/>\n\nThere are many RAG pipelines and modules out there,\nbut you don\u2019t know what pipeline is great for \u201cyour own data\u201d and \"your own use-case.\"\nMaking and evaluating all RAG modules is very time-consuming and hard to do.\nBut without it, you will never know which RAG pipeline is the best for your own use-case.\n\nAutoRAG is a tool for finding the optimal RAG pipeline for \u201cyour data.\u201d\nYou can evaluate various RAG modules automatically with your own evaluation data\nand find the best RAG pipeline for your own use-case.\n\nAutoRAG supports a simple way to evaluate many RAG module combinations.\nTry now and find the best RAG pipeline for your own use-case.\n\nExplore our \ud83d\udcd6 [Document](https://docs.auto-rag.com)!!\n\nPlus, join our \ud83d\udcde [Discord](https://discord.gg/P4DYXfmSAs) Community.\n\n---\n\nDo you have any difficulties in optimizing your RAG pipeline?\nOr is it hard to set up things to use AutoRAG?\nTry [**AutoRAG Cloud**](https://tally.so/r/n0jOrZ) beta.\nWe will help you to run AutoRAG and optimize.\nPlus, we can help you to build RAG evaluation dataset.\n\nStarts with 9.99$ per optimization.\n\n---\n\n## YouTube Tutorial\n\nhttps://github.com/Marker-Inc-Korea/AutoRAG/assets/96727832/c0d23896-40c0-479f-a17b-aa2ec3183a26\n\n_Muted by default, enable sound for voice-over_\n\nYou can see on [YouTube](https://youtu.be/2ojK8xjyXAU?feature=shared)\n\n## Use AutoRAG in HuggingFace Space \ud83d\ude80\n\n- [\ud83d\udcac Naive RAG Chatbot](https://huggingface.co/spaces/AutoRAG/Naive-RAG-chatbot)\n- [\u270f\ufe0f AutoRAG Data Creation](https://huggingface.co/spaces/AutoRAG/AutoRAG-data-creation)\n- [\ud83d\ude80 AutoRAG RAG Pipeline Optimization](https://huggingface.co/spaces/AutoRAG/AutoRAG-optimization)\n\n## Colab Tutorial\n\n- [Step 1: Basic of AutoRAG | Optimizing your RAG pipeline](https://colab.research.google.com/drive/19OEQXO_pHN6gnn2WdfPd4hjnS-4GurVd?usp=sharing)\n- [Step 2: Data Creation | Create your own Data for RAG Optimization](https://colab.research.google.com/drive/1BOdzMndYgMY_iqhwKcCCS7ezHbZ4Oz5X?usp=sharing)\n- [Step 3: Use Custom LLM & Embedding Model | Use Custom Model](https://colab.research.google.com/drive/12VpWcSTSOsLSyW0BKb-kPoEzK22ACxvS?usp=sharing)\n\n# Index\n\n- [Quick Install](#quick-install)\n- [Data Creation](#data-creation)\n - [Parsing](#1-parsing)\n - [Chunking](#2-chunking)\n - [QA Creation](#3-qa-creation)\n- [RAG Optimization](#rag-optimization)\n - [How AutoRAG optimizes RAG pipeline?](#how-autorag-optimizes-rag-pipeline)\n - [Metrics](#metrics)\n - [Quick Start](#quick-start-1)\n - [Set YAML File](#1-set-yaml-file)\n - [Run AutoRAG](#2-run-autorag)\n - [Run Dashboard](#3-run-dashboard)\n - [Deploy your optimal RAG pipeline](#4-deploy-your-optimal-rag-pipeline)\n- [\ud83d\udc33 AutoRAG Docker Guide](#-autorag-docker-guide)\n- [FaQ](#-faq)\n\n# Quick Install\n\nWe recommend using Python version 3.10 or higher for AutoRAG.\n\n```bash\npip install AutoRAG\n```\n\nIf you want to use the local models, you need to install gpu version.\n\n```bash\npip install \"AutoRAG[gpu]\"\n```\n\nOr for parsing, you can use the parsing version.\n```bash\npip install \"AutoRAG[gpu,parse]\"\n```\n\n# Data Creation\n\n<a href=\"https://huggingface.co/spaces/AutoRAG/AutoRAG-data-creation\">\n<img src=\"https://github.com/user-attachments/assets/8c6e4b02-3938-4560-b817-c95764965b50\" alt=\"Hugging Face Sticker\" style=\"width:200px;height:auto;\">\n</a>\n\n\n\n\n\nRAG Optimization requires two types of data: QA dataset and Corpus dataset.\n\n1. **QA** dataset file (qa.parquet)\n2. **Corpus** dataset file (corpus.parquet)\n\n**QA** dataset is important for accurate and reliable evaluation and optimization.\n\n**Corpus** dataset is critical to the performance of RAGs.\nThis is because RAG uses the corpus to retrieve documents and generate answers using it.\n\n### \ud83d\udccc Supporting Data Creation Modules\n\n\n\n- [Supporting Parsing Modules List](https://edai.notion.site/Supporting-Parsing-Modules-e0b7579c7c0e4fb2963e408eeccddd75?pvs=4)\n- [Supporting Chunking Modules List](https://edai.notion.site/Supporting-Chunk-Modules-8db803dba2ec4cd0a8789659106e86a3?pvs=4)\n\n\n## Quick Start\n\n### 1. Parsing\n\n#### Set YAML File\n\n```yaml\nmodules:\n - module_type: langchain_parse\n parse_method: pdfminer\n```\n\nYou can also use multiple Parse modules at once.\nHowever, in this case, you'll need to return a new process for each parsed result.\n\n#### Start Parsing\n\nYou can parse your raw documents with just a few lines of code.\n\n```python\nfrom autorag.parser import Parser\n\nparser = Parser(data_path_glob=\"your/data/path/*\")\nparser.start_parsing(\"your/path/to/parse_config.yaml\")\n```\n\n### 2. Chunking\n\n#### Set YAML File\n\n```yaml\nmodules:\n - module_type: llama_index_chunk\n chunk_method: Token\n chunk_size: 1024\n chunk_overlap: 24\n add_file_name: en\n```\n\nYou can also use multiple Chunk modules at once.\nIn this case, you need to use one corpus to create QA and then map the rest of the corpus to QA Data.\nIf the chunk method is different, the retrieval_gt will be different, so we need to remap it to the QA dataset.\n\n#### Start Chunking\n\nYou can chunk your parsed results with just a few lines of code.\n\n```python\nfrom autorag.chunker import Chunker\n\nchunker = Chunker.from_parquet(parsed_data_path=\"your/parsed/data/path\")\nchunker.start_chunking(\"your/path/to/chunk_config.yaml\")\n```\n\n### 3. QA Creation\n\nYou can create QA dataset with just a few lines of code.\n\n```python\nimport pandas as pd\nfrom llama_index.llms.openai import OpenAI\n\nfrom autorag.data.qa.filter.dontknow import dontknow_filter_rule_based\nfrom autorag.data.qa.generation_gt.llama_index_gen_gt import (\n make_basic_gen_gt,\n make_concise_gen_gt,\n)\nfrom autorag.data.qa.schema import Raw, Corpus\nfrom autorag.data.qa.query.llama_gen_query import factoid_query_gen\nfrom autorag.data.qa.sample import random_single_hop\n\nllm = OpenAI()\nraw_df = pd.read_parquet(\"your/path/to/parsed.parquet\")\nraw_instance = Raw(raw_df)\n\ncorpus_df = pd.read_parquet(\"your/path/to/corpus.parquet\")\ncorpus_instance = Corpus(corpus_df, raw_instance)\n\ninitial_qa = (\n corpus_instance.sample(random_single_hop, n=3)\n .map(\n lambda df: df.reset_index(drop=True),\n )\n .make_retrieval_gt_contents()\n .batch_apply(\n factoid_query_gen, # query generation\n llm=llm,\n )\n .batch_apply(\n make_basic_gen_gt, # answer generation (basic)\n llm=llm,\n )\n .batch_apply(\n make_concise_gen_gt, # answer generation (concise)\n llm=llm,\n )\n .filter(\n dontknow_filter_rule_based, # filter don't know\n lang=\"en\",\n )\n)\n\ninitial_qa.to_parquet('./qa.parquet', './corpus.parquet')\n```\n\n# RAG Optimization\n\n<a href=\"https://huggingface.co/spaces/AutoRAG/RAG-Pipeline-Optimization\">\n<img src=\"https://github.com/user-attachments/assets/8c6e4b02-3938-4560-b817-c95764965b50\" alt=\"Hugging Face Sticker\" style=\"width:200px;height:auto;\">\n</a>\n\n\n\n\n\n## How AutoRAG optimizes RAG pipeline?\n\nHere is the AutoRAG RAG Structure that only show Nodes.\n\n\n\nHere is the image showing all the nodes and modules.\n\n\n\n\n\n### \ud83d\udccc Supporting RAG Optimization Nodes & modules\n\n- [Supporting RAG Modules list](https://edai.notion.site/Supporting-Nodes-modules-0ebc7810649f4e41aead472a92976be4?pvs=4)\n\n## Metrics\n\nThe metrics used by each node in AutoRAG are shown below.\n\n\n\n\n\n- [Supporting metrics list](https://edai.notion.site/Supporting-metrics-867d71caefd7401c9264dd91ba406043?pvs=4)\n\nHere is the detailed information about the metrics that AutoRAG supports.\n- [Retrieval Metrics](https://edai.notion.site/Retrieval-Metrics-dde3d9fa1d9547cdb8b31b94060d21e7?pvs=4)\n- [Retrieval Token Metrics](https://edai.notion.site/Retrieval-Token-Metrics-c3e2d83358e04510a34b80429ebb543f?pvs=4)\n- [Generation Metrics](https://github.com/user-attachments/assets/7d4a3069-9186-4854-885d-ca0f7bcc17e8)\n\n## Quick Start\n\n### 1. Set YAML File\n\nFirst, you need to set the config YAML file for your RAG optimization.\n\nWe highly recommend using pre-made config YAML files for starter.\n\n- [Get Sample YAML](./sample_config/rag)\n - [Sample YAML Guide](https://docs.auto-rag.com/optimization/sample_config.html)\n- [Make Custom YAML Guide](https://docs.auto-rag.com/optimization/custom_config.html)\n\n\nHere is an example of the config YAML file to use `retrieval`, `prompt_maker`, and `generator` nodes.\n\n```yaml\nnode_lines:\n- node_line_name: retrieve_node_line # Set Node Line (Arbitrary Name)\n nodes:\n - node_type: retrieval # Set Retrieval Node\n strategy:\n metrics: [retrieval_f1, retrieval_recall, retrieval_ndcg, retrieval_mrr] # Set Retrieval Metrics\n top_k: 3\n modules:\n - module_type: vectordb\n vectordb: default\n - module_type: bm25\n - module_type: hybrid_rrf\n weight_range: (4,80)\n- node_line_name: post_retrieve_node_line # Set Node Line (Arbitrary Name)\n nodes:\n - node_type: prompt_maker # Set Prompt Maker Node\n strategy:\n metrics: # Set Generation Metrics\n - metric_name: meteor\n - metric_name: rouge\n - metric_name: sem_score\n embedding_model: openai\n modules:\n - module_type: fstring\n prompt: \"Read the passages and answer the given question. \\n Question: {query} \\n Passage: {retrieved_contents} \\n Answer : \"\n - node_type: generator # Set Generator Node\n strategy:\n metrics: # Set Generation Metrics\n - metric_name: meteor\n - metric_name: rouge\n - metric_name: sem_score\n embedding_model: openai\n modules:\n - module_type: openai_llm\n llm: gpt-4o-mini\n batch: 16\n```\n\n### 2. Run AutoRAG\n\nYou can evaluate your RAG pipeline with just a few lines of code.\n\n```python\nfrom autorag.evaluator import Evaluator\n\nevaluator = Evaluator(qa_data_path='your/path/to/qa.parquet', corpus_data_path='your/path/to/corpus.parquet')\nevaluator.start_trial('your/path/to/config.yaml')\n```\n\nor you can use the command line interface\n\n```bash\nautorag evaluate --config your/path/to/default_config.yaml --qa_data_path your/path/to/qa.parquet --corpus_data_path your/path/to/corpus.parquet\n```\n\nOnce it is done, you can see several files and folders created in your current directory.\nAt the trial folder named to numbers (like 0),\nyou can check `summary.csv` file that summarizes the evaluation results and the best RAG pipeline for your data.\n\nFor more details, you can check out how the folder structure looks like\nat [here](https://docs.auto-rag.com/optimization/folder_structure.html).\n\n### 3. Run Dashboard\n\nYou can run a dashboard to easily see the result.\n\n```bash\nautorag dashboard --trial_dir /your/path/to/trial_dir\n```\n\n#### sample dashboard\n\n\n\n### 4. Deploy your optimal RAG pipeline\n\n### 4-1. Run as a Code\n\nYou can use an optimal RAG pipeline right away from the trial folder.\nThe trial folder is the directory used in the running dashboard. (like 0, 1, 2, ...)\n\n```python\nfrom autorag.deploy import Runner\n\nrunner = Runner.from_trial_folder('/your/path/to/trial_dir')\nrunner.run('your question')\n```\n\n### 4-2. Run as an API server\n\nYou can run this pipeline as an API server.\n\nCheck out the API endpoint at [here](./docs/source/deploy/api_endpoint.md).\n\n```python\nimport nest_asyncio\nfrom autorag.deploy import ApiRunner\n\nnest_asyncio.apply()\n\nrunner = ApiRunner.from_trial_folder('/your/path/to/trial_dir')\nrunner.run_api_server()\n```\n\n```bash\nautorag run_api --trial_dir your/path/to/trial_dir --host 0.0.0.0 --port 8000\n```\n\nThe cli command uses extracted config YAML file. If you want to know it more, check out [here](https://docs.auto-rag.com/tutorial.html#extract-pipeline-and-evaluate-test-dataset).\n\n### 4-3. Run as a Web Interface\n\nyou can run this pipeline as a web interface.\n\nCheck out the web interface at [here](deploy/web.md).\n\n```bash\nautorag run_web --trial_path your/path/to/trial_path\n```\n\n#### sample web interface\n\n<img width=\"1491\" alt=\"web_interface\" src=\"https://github.com/Marker-Inc-Korea/AutoRAG/assets/96727832/f6b00353-f6bb-4d8f-8740-1c264c0acbb8\">\n\n### Use advanced web interface\n\nYou can deploy the advanced web interface featured by [Kotaemon](https://github.com/Cinnamon/kotaemon) to the fly.io.\nGo [here](https://github.com/vkehfdl1/AutoRAG-web-kotaemon) to use it and deploy to the fly.io.\n\nExample :\n\n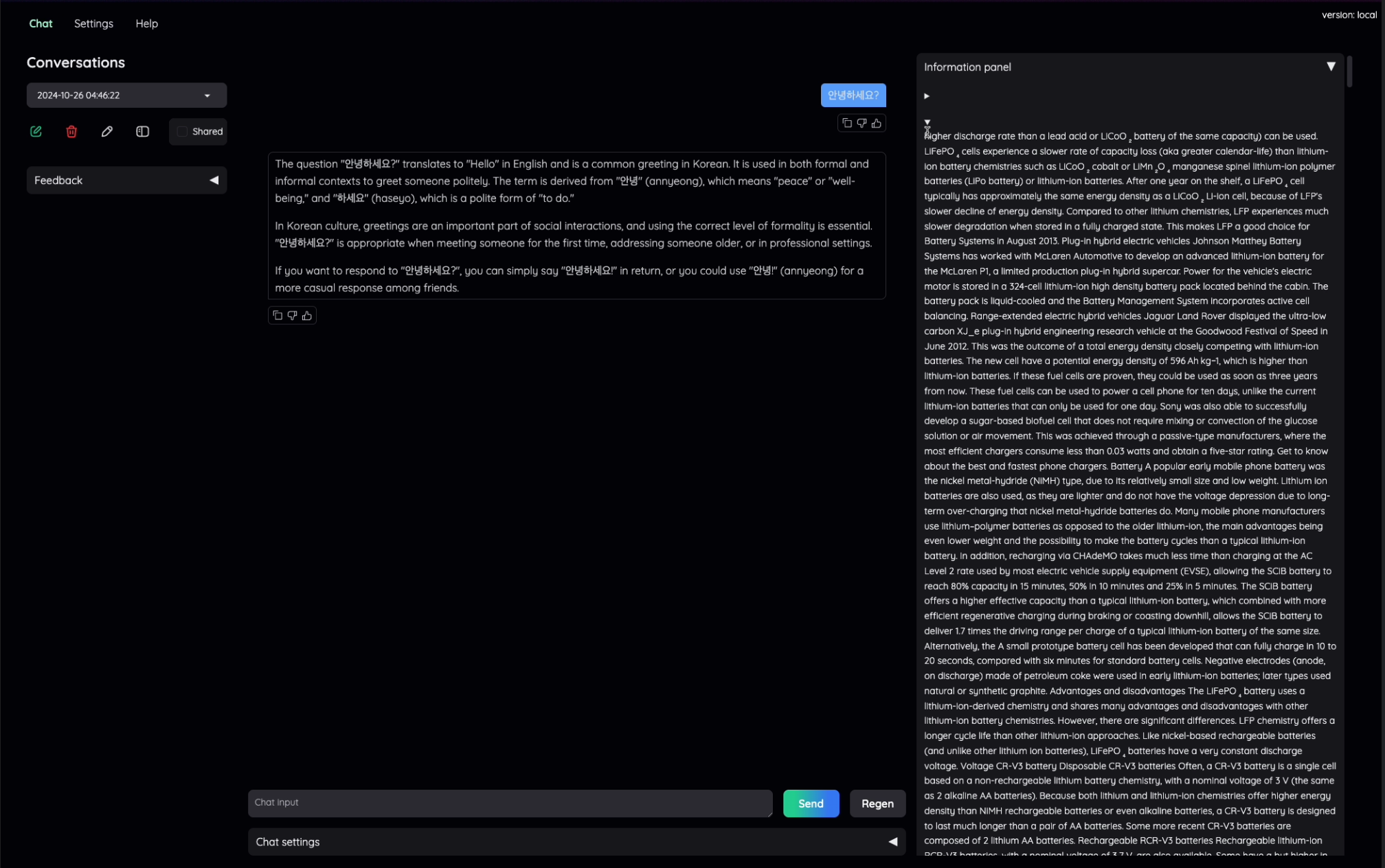\n\n## \ud83d\udc33 AutoRAG Docker Guide\n\nThis guide provides a quick overview of building and running the AutoRAG Docker container for production, with instructions on setting up the environment for evaluation using your configuration and data paths.\n\n### \ud83d\ude80 Building the Docker Image\n\nTip: If you want to build an image for a gpu version, you can use `autoraghq/autorag:gpu` or `autoraghq/autorag:gpu-parsing`\n\n#### 1.Download dataset for [Tutorial Step 1](https://colab.research.google.com/drive/19OEQXO_pHN6gnn2WdfPd4hjnS-4GurVd?usp=sharing)\n```bash\npython sample_dataset/eli5/load_eli5_dataset.py --save_path projects/tutorial_1\n```\n\n#### 2. Run `evaluate`\n> **Note**: This step may take a long time to complete and involves OpenAI API calls, which may cost approximately $0.30.\n\n```bash\ndocker run --rm -it \\\n -v ~/.cache/huggingface:/root/.cache/huggingface \\\n -v $(pwd)/projects:/usr/src/app/projects \\\n -e OPENAI_API_KEY=${OPENAI_API_KEY} \\\n autoraghq/autorag:api-latest evaluate \\\n --config /usr/src/app/projects/tutorial_1/config.yaml \\\n --qa_data_path /usr/src/app/projects/tutorial_1/qa_test.parquet \\\n --corpus_data_path /usr/src/app/projects/tutorial_1/corpus.parquet \\\n --project_dir /usr/src/app/projects/tutorial_1/\n```\n\n\n#### 3. Run validate\n```bash\ndocker run --rm -it \\\n -v ~/.cache/huggingface:/root/.cache/huggingface \\\n -v $(pwd)/projects:/usr/src/app/projects \\\n -e OPENAI_API_KEY=${OPENAI_API_KEY} \\\n autoraghq/autorag:api-latest validate \\\n --config /usr/src/app/projects/tutorial_1/config.yaml \\\n --qa_data_path /usr/src/app/projects/tutorial_1/qa_test.parquet \\\n --corpus_data_path /usr/src/app/projects/tutorial_1/corpus.parquet\n```\n\n\n#### 4. Run `dashboard`\n```bash\ndocker run --rm -it \\\n -v ~/.cache/huggingface:/root/.cache/huggingface \\\n -v $(pwd)/projects:/usr/src/app/projects \\\n -e OPENAI_API_KEY=${OPENAI_API_KEY} \\\n -p 8502:8502 \\\n autoraghq/autorag:api-latest dashboard \\\n --trial_dir /usr/src/app/projects/tutorial_1/0\n```\n\n\n#### 4. Run `run_web`\n```bash\ndocker run --rm -it \\\n -v ~/.cache/huggingface:/root/.cache/huggingface \\\n -v $(pwd)/projects:/usr/src/app/projects \\\n -e OPENAI_API_KEY=${OPENAI_API_KEY} \\\n -p 8501:8501 \\\n autoraghq/autorag:api-latest run_web --trial_path ./projects/tutorial_1/0\n```\n\n#### Key Points :\n- **`-v ~/.cache/huggingface:/cache/huggingface`**: Mounts the host machine\u2019s Hugging Face cache to `/cache/huggingface` in the container, enabling access to pre-downloaded models.\n- **`-e OPENAI_API_KEY: ${OPENAI_API_KEY}`**: Passes the `OPENAI_API_KEY` from your host environment.\n\nFor more detailed instructions, refer to the [Docker Installation Guide](./docs/source/install.md#1-build-the-docker-image).\n\n## \u260e\ufe0f FaQ\n\n\ud83d\udee3\ufe0f [Roadmap](https://github.com/orgs/Auto-RAG/projects/1/views/2)\n\n\ud83d\udcbb [Hardware Specs](https://edai.notion.site/Hardware-specs-28cefcf2a26246ffadc91e2f3dc3d61c?pvs=4)\n\n\u2b50 [Running AutoRAG](https://edai.notion.site/About-running-AutoRAG-44a8058307af42068fc218a073ee480b?pvs=4)\n\n\ud83c\udf6f [Tips/Tricks](https://edai.notion.site/Tips-Tricks-10708a0e36ff461cb8a5d4fb3279ff15?pvs=4)\n\n\u260e\ufe0f [TroubleShooting](https://medium.com/@autorag/autorag-troubleshooting-5cf872b100e3)\n\n## Thanks for shoutout\n\n### Company\n\n<a href=\"https://www.linkedin.com/posts/llamaindex_rag-pipelines-have-a-lot-of-hyperparameters-activity-7182053546593247232-HFMN/\">\n<img src=\"https://github.com/user-attachments/assets/b8fdaaf6-543a-4019-8dbe-44191a5269b9\" alt=\"llama index\" style=\"width:200px;height:auto;\">\n</a>\n\n### Individual\n- [Shubham Saboo](https://www.linkedin.com/posts/shubhamsaboo_just-found-the-solution-to-the-biggest-rag-activity-7255404464054939648-ISQ8/)\n- [Kalyan KS](https://www.linkedin.com/posts/kalyanksnlp_rag-autorag-llms-activity-7258677155574788097-NgS0/)\n\n## \ud83d\udcac Talk with Founders\n\nTalk with us! We are always open to talk with you.\n\n- \ud83c\udfa4 [Talk with Jeffrey](https://zcal.co/autorag-jeffrey/autorag-demo-15min)\n\n- \ud83e\udd9c [Talk with Bwook](https://zcal.co/i/tcuLtmq5)\n\n---\n\n# \u2728 Contributors \u2728\n\nThanks go to these wonderful people:\n\n<a href=\"https://github.com/Marker-Inc-Korea/AutoRAG/graphs/contributors\">\n <img src=\"https://contrib.rocks/image?repo=Marker-Inc-Korea/AutoRAG\" />\n</a>\n\n# Contribution\n\nWe are developing AutoRAG as open-source.\n\nSo this project welcomes contributions and suggestions. Feel free to contribute to this project.\n\nPlus, check out our detailed documentation at [here](https://docs.auto-rag.com/index.html).\n\n\n## Citation\n\n```bibtex\n@misc{kim2024autoragautomatedframeworkoptimization,\n title={AutoRAG: Automated Framework for optimization of Retrieval Augmented Generation Pipeline},\n author={Dongkyu Kim and Byoungwook Kim and Donggeon Han and Matou\u0161 Eibich},\n year={2024},\n eprint={2410.20878},\n archivePrefix={arXiv},\n primaryClass={cs.CL},\n url={https://arxiv.org/abs/2410.20878},\n}\n```\n",
"bugtrack_url": null,
"license": "Apache License\n Version 2.0, January 2004\n http://www.apache.org/licenses/\n \n TERMS AND CONDITIONS FOR USE, REPRODUCTION, AND DISTRIBUTION\n \n 1. Definitions.\n \n \"License\" shall mean the terms and conditions for use, reproduction,\n and distribution as defined by Sections 1 through 9 of this document.\n \n \"Licensor\" shall mean the copyright owner or entity authorized by\n the copyright owner that is granting the License.\n \n \"Legal Entity\" shall mean the union of the acting entity and all\n other entities that control, are controlled by, or are under common\n control with that entity. For the purposes of this definition,\n \"control\" means (i) the power, direct or indirect, to cause the\n direction or management of such entity, whether by contract or\n otherwise, or (ii) ownership of fifty percent (50%) or more of the\n outstanding shares, or (iii) beneficial ownership of such entity.\n \n \"You\" (or \"Your\") shall mean an individual or Legal Entity\n exercising permissions granted by this License.\n \n \"Source\" form shall mean the preferred form for making modifications,\n including but not limited to software source code, documentation\n source, and configuration files.\n \n \"Object\" form shall mean any form resulting from mechanical\n transformation or translation of a Source form, including but\n not limited to compiled object code, generated documentation,\n and conversions to other media types.\n \n \"Work\" shall mean the work of authorship, whether in Source or\n Object form, made available under the License, as indicated by a\n copyright notice that is included in or attached to the work\n (an example is provided in the Appendix below).\n \n \"Derivative Works\" shall mean any work, whether in Source or Object\n form, that is based on (or derived from) the Work and for which the\n editorial revisions, annotations, elaborations, or other modifications\n represent, as a whole, an original work of authorship. For the purposes\n of this License, Derivative Works shall not include works that remain\n separable from, or merely link (or bind by name) to the interfaces of,\n the Work and Derivative Works thereof.\n \n \"Contribution\" shall mean any work of authorship, including\n the original version of the Work and any modifications or additions\n to that Work or Derivative Works thereof, that is intentionally\n submitted to Licensor for inclusion in the Work by the copyright owner\n or by an individual or Legal Entity authorized to submit on behalf of\n the copyright owner. For the purposes of this definition, \"submitted\"\n means any form of electronic, verbal, or written communication sent\n to the Licensor or its representatives, including but not limited to\n communication on electronic mailing lists, source code control systems,\n and issue tracking systems that are managed by, or on behalf of, the\n Licensor for the purpose of discussing and improving the Work, but\n excluding communication that is conspicuously marked or otherwise\n designated in writing by the copyright owner as \"Not a Contribution.\"\n \n \"Contributor\" shall mean Licensor and any individual or Legal Entity\n on behalf of whom a Contribution has been received by Licensor and\n subsequently incorporated within the Work.\n \n 2. Grant of Copyright License. Subject to the terms and conditions of\n this License, each Contributor hereby grants to You a perpetual,\n worldwide, non-exclusive, no-charge, royalty-free, irrevocable\n copyright license to reproduce, prepare Derivative Works of,\n publicly display, publicly perform, sublicense, and distribute the\n Work and such Derivative Works in Source or Object form.\n \n 3. Grant of Patent License. Subject to the terms and conditions of\n this License, each Contributor hereby grants to You a perpetual,\n worldwide, non-exclusive, no-charge, royalty-free, irrevocable\n (except as stated in this section) patent license to make, have made,\n use, offer to sell, sell, import, and otherwise transfer the Work,\n where such license applies only to those patent claims licensable\n by such Contributor that are necessarily infringed by their\n Contribution(s) alone or by combination of their Contribution(s)\n with the Work to which such Contribution(s) was submitted. If You\n institute patent litigation against any entity (including a\n cross-claim or counterclaim in a lawsuit) alleging that the Work\n or a Contribution incorporated within the Work constitutes direct\n or contributory patent infringement, then any patent licenses\n granted to You under this License for that Work shall terminate\n as of the date such litigation is filed.\n \n 4. Redistribution. You may reproduce and distribute copies of the\n Work or Derivative Works thereof in any medium, with or without\n modifications, and in Source or Object form, provided that You\n meet the following conditions:\n \n (a) You must give any other recipients of the Work or\n Derivative Works a copy of this License; and\n \n (b) You must cause any modified files to carry prominent notices\n stating that You changed the files; and\n \n (c) You must retain, in the Source form of any Derivative Works\n that You distribute, all copyright, patent, trademark, and\n attribution notices from the Source form of the Work,\n excluding those notices that do not pertain to any part of\n the Derivative Works; and\n \n (d) If the Work includes a \"NOTICE\" text file as part of its\n distribution, then any Derivative Works that You distribute must\n include a readable copy of the attribution notices contained\n within such NOTICE file, excluding those notices that do not\n pertain to any part of the Derivative Works, in at least one\n of the following places: within a NOTICE text file distributed\n as part of the Derivative Works; within the Source form or\n documentation, if provided along with the Derivative Works; or,\n within a display generated by the Derivative Works, if and\n wherever such third-party notices normally appear. The contents\n of the NOTICE file are for informational purposes only and\n do not modify the License. You may add Your own attribution\n notices within Derivative Works that You distribute, alongside\n or as an addendum to the NOTICE text from the Work, provided\n that such additional attribution notices cannot be construed\n as modifying the License.\n \n You may add Your own copyright statement to Your modifications and\n may provide additional or different license terms and conditions\n for use, reproduction, or distribution of Your modifications, or\n for any such Derivative Works as a whole, provided Your use,\n reproduction, and distribution of the Work otherwise complies with\n the conditions stated in this License.\n \n 5. Submission of Contributions. Unless You explicitly state otherwise,\n any Contribution intentionally submitted for inclusion in the Work\n by You to the Licensor shall be under the terms and conditions of\n this License, without any additional terms or conditions.\n Notwithstanding the above, nothing herein shall supersede or modify\n the terms of any separate license agreement you may have executed\n with Licensor regarding such Contributions.\n \n 6. Trademarks. This License does not grant permission to use the trade\n names, trademarks, service marks, or product names of the Licensor,\n except as required for reasonable and customary use in describing the\n origin of the Work and reproducing the content of the NOTICE file.\n \n 7. Disclaimer of Warranty. Unless required by applicable law or\n agreed to in writing, Licensor provides the Work (and each\n Contributor provides its Contributions) on an \"AS IS\" BASIS,\n WITHOUT WARRANTIES OR CONDITIONS OF ANY KIND, either express or\n implied, including, without limitation, any warranties or conditions\n of TITLE, NON-INFRINGEMENT, MERCHANTABILITY, or FITNESS FOR A\n PARTICULAR PURPOSE. You are solely responsible for determining the\n appropriateness of using or redistributing the Work and assume any\n risks associated with Your exercise of permissions under this License.\n \n 8. Limitation of Liability. In no event and under no legal theory,\n whether in tort (including negligence), contract, or otherwise,\n unless required by applicable law (such as deliberate and grossly\n negligent acts) or agreed to in writing, shall any Contributor be\n liable to You for damages, including any direct, indirect, special,\n incidental, or consequential damages of any character arising as a\n result of this License or out of the use or inability to use the\n Work (including but not limited to damages for loss of goodwill,\n work stoppage, computer failure or malfunction, or any and all\n other commercial damages or losses), even if such Contributor\n has been advised of the possibility of such damages.\n \n 9. Accepting Warranty or Additional Liability. While redistributing\n the Work or Derivative Works thereof, You may choose to offer,\n and charge a fee for, acceptance of support, warranty, indemnity,\n or other liability obligations and/or rights consistent with this\n License. However, in accepting such obligations, You may act only\n on Your own behalf and on Your sole responsibility, not on behalf\n of any other Contributor, and only if You agree to indemnify,\n defend, and hold each Contributor harmless for any liability\n incurred by, or claims asserted against, such Contributor by reason\n of your accepting any such warranty or additional liability.\n \n END OF TERMS AND CONDITIONS\n \n APPENDIX: How to apply the Apache License to your work.\n \n To apply the Apache License to your work, attach the following\n boilerplate notice, with the fields enclosed by brackets \"[]\"\n replaced with your own identifying information. (Don't include\n the brackets!) The text should be enclosed in the appropriate\n comment syntax for the file format. We also recommend that a\n file or class name and description of purpose be included on the\n same \"printed page\" as the copyright notice for easier\n identification within third-party archives.\n \n Copyright [yyyy] [name of copyright owner]\n \n Licensed under the Apache License, Version 2.0 (the \"License\");\n you may not use this file except in compliance with the License.\n You may obtain a copy of the License at\n \n http://www.apache.org/licenses/LICENSE-2.0\n \n Unless required by applicable law or agreed to in writing, software\n distributed under the License is distributed on an \"AS IS\" BASIS,\n WITHOUT WARRANTIES OR CONDITIONS OF ANY KIND, either express or implied.\n See the License for the specific language governing permissions and\n limitations under the License.\n ",
"summary": "Automatically Evaluate RAG pipelines with your own data. Find optimal structure for new RAG product.",
"version": "0.3.13",
"project_urls": {
"Homepage": "https://github.com/Marker-Inc-Korea/AutoRAG"
},
"split_keywords": [
"rag",
" autorag",
" autorag",
" rag-evaluation",
" evaluation",
" rag-auto",
" automl",
" automl-rag"
],
"urls": [
{
"comment_text": null,
"digests": {
"blake2b_256": "e61032f8d2772e0fe784263221b05014b597dce9f1eb472b23560fda675953e9",
"md5": "54746ee97090a71641e46128702a9f55",
"sha256": "28fa95e3a8aedb36f3989135ee251bcc7f9489f41a7da4cbdbedc7fd1f5f337c"
},
"downloads": -1,
"filename": "AutoRAG-0.3.13-py3-none-any.whl",
"has_sig": false,
"md5_digest": "54746ee97090a71641e46128702a9f55",
"packagetype": "bdist_wheel",
"python_version": "py3",
"requires_python": ">=3.10",
"size": 247686,
"upload_time": "2025-01-25T05:28:34",
"upload_time_iso_8601": "2025-01-25T05:28:34.568107Z",
"url": "https://files.pythonhosted.org/packages/e6/10/32f8d2772e0fe784263221b05014b597dce9f1eb472b23560fda675953e9/AutoRAG-0.3.13-py3-none-any.whl",
"yanked": false,
"yanked_reason": null
},
{
"comment_text": null,
"digests": {
"blake2b_256": "69d0bf645c26c194e8abc5d331f816b63f72cf784a3329c0ff4080ce51ed0979",
"md5": "523009fd62c37ec3c3a8977e59e414c7",
"sha256": "f46aa35139b99ef8fe8842c08a59b871881a563cb3cf7ccc8cfd61725287bfbb"
},
"downloads": -1,
"filename": "autorag-0.3.13.tar.gz",
"has_sig": false,
"md5_digest": "523009fd62c37ec3c3a8977e59e414c7",
"packagetype": "sdist",
"python_version": "source",
"requires_python": ">=3.10",
"size": 8464326,
"upload_time": "2025-01-25T05:28:38",
"upload_time_iso_8601": "2025-01-25T05:28:38.068303Z",
"url": "https://files.pythonhosted.org/packages/69/d0/bf645c26c194e8abc5d331f816b63f72cf784a3329c0ff4080ce51ed0979/autorag-0.3.13.tar.gz",
"yanked": false,
"yanked_reason": null
}
],
"upload_time": "2025-01-25 05:28:38",
"github": true,
"gitlab": false,
"bitbucket": false,
"codeberg": false,
"github_user": "Marker-Inc-Korea",
"github_project": "AutoRAG",
"travis_ci": false,
"coveralls": false,
"github_actions": true,
"requirements": [
{
"name": "pydantic",
"specs": [
[
"<",
"2.10.0"
]
]
},
{
"name": "numpy",
"specs": [
[
"<",
"2.0.0"
]
]
},
{
"name": "pandas",
"specs": [
[
">=",
"2.1.0"
]
]
},
{
"name": "tqdm",
"specs": []
},
{
"name": "tiktoken",
"specs": [
[
">=",
"0.7.0"
]
]
},
{
"name": "openai",
"specs": [
[
">=",
"1.0.0"
]
]
},
{
"name": "rank_bm25",
"specs": []
},
{
"name": "pyyaml",
"specs": []
},
{
"name": "pyarrow",
"specs": []
},
{
"name": "fastparquet",
"specs": []
},
{
"name": "sacrebleu",
"specs": []
},
{
"name": "evaluate",
"specs": []
},
{
"name": "rouge_score",
"specs": []
},
{
"name": "rich",
"specs": []
},
{
"name": "click",
"specs": []
},
{
"name": "cohere",
"specs": [
[
">=",
"5.8.0"
]
]
},
{
"name": "tokenlog",
"specs": [
[
">=",
"0.0.2"
]
]
},
{
"name": "aiohttp",
"specs": []
},
{
"name": "voyageai",
"specs": []
},
{
"name": "mixedbread-ai",
"specs": []
},
{
"name": "llama-index-llms-bedrock",
"specs": []
},
{
"name": "scikit-learn",
"specs": []
},
{
"name": "emoji",
"specs": []
},
{
"name": "pymilvus",
"specs": [
[
">=",
"2.3.0"
]
]
},
{
"name": "chromadb",
"specs": [
[
">=",
"0.5.0"
]
]
},
{
"name": "weaviate-client",
"specs": []
},
{
"name": "pinecone",
"specs": []
},
{
"name": "couchbase",
"specs": []
},
{
"name": "qdrant-client",
"specs": []
},
{
"name": "quart",
"specs": []
},
{
"name": "pyngrok",
"specs": []
},
{
"name": "llama-index",
"specs": [
[
">=",
"0.11.0"
]
]
},
{
"name": "llama-index-core",
"specs": [
[
">=",
"0.11.0"
]
]
},
{
"name": "llama-index-readers-file",
"specs": []
},
{
"name": "llama-index-embeddings-openai",
"specs": []
},
{
"name": "llama-index-embeddings-ollama",
"specs": []
},
{
"name": "llama-index-llms-openai",
"specs": [
[
">=",
"0.2.7"
]
]
},
{
"name": "llama-index-llms-openai-like",
"specs": []
},
{
"name": "llama-index-retrievers-bm25",
"specs": []
},
{
"name": "streamlit",
"specs": []
},
{
"name": "gradio",
"specs": []
},
{
"name": "langchain-core",
"specs": [
[
">=",
"0.3.0"
]
]
},
{
"name": "langchain-unstructured",
"specs": [
[
">=",
"0.1.5"
]
]
},
{
"name": "langchain-upstage",
"specs": []
},
{
"name": "langchain-community",
"specs": [
[
">=",
"0.3.0"
]
]
},
{
"name": "panel",
"specs": []
},
{
"name": "seaborn",
"specs": []
},
{
"name": "ipykernel",
"specs": []
},
{
"name": "ipywidgets",
"specs": []
},
{
"name": "ipywidgets_bokeh",
"specs": []
}
],
"lcname": "autorag"
}