Name | federleicht JSON |
Version |
0.1.0
JSON |
| download |
home_page | None |
Summary | lightweigth function decorators to cache your `pandas.DataFrame` as feather. |
upload_time | 2024-12-01 17:00:19 |
maintainer | None |
docs_url | None |
author | Christoph Dörrer |
requires_python | <4.0,>=3.9 |
license | MIT |
keywords |
cache
feather
pandas
pyarrow
|
VCS |
 |
bugtrack_url |
|
requirements |
No requirements were recorded.
|
Travis-CI |
No Travis.
|
coveralls test coverage |
No coveralls.
|
# federleicht
[](https://pypi.org/project/federleicht/)
[](https://pypi.org/project/federleicht/)
[](https://pypi.org/project/federleicht/)
[](https://raw.githubusercontent.com/d-chris/federleicht/main/LICENSE)
[](https://github.com/d-chris/federleicht/actions/workflows/pytest.yml)
[](https://d-chris.github.io/federleicht)
[](https://github.com/d-chris/federleicht)
[](https://raw.githubusercontent.com/d-chris/federleicht/main/.pre-commit-config.yaml)
[](https://codecov.io/gh/d-chris/federleicht)
---
`federleicht` is a Python package providing a cache decorator for `pandas.DataFrame`, utilizing the lightweight and efficient `pyarrow` feather file format.
`federleicht.cache_dataframe` is designed to decorate functions that return `pandas.DataFrame` objects. The decorator saves the DataFrame to a feather file on the first call and loads it automatically on subsequent calls if the file exists.
## Key Features
- Feather Integration: Save and load `pandas.DataFrame` effortlessly using the Feather format, known for its speed and simplicity.
- Decorator Simplicity: Add caching functionality to your functions with a single decorator line.
- Efficient Caching: Avoid redundant computations by reusing cached results.
## Cache Expiry
To implement cache expiry, `federleicht` requires all arguments of the decorated function to be serializable. The cache will expire under the following conditions:
- Argument Sensitivity: Cache will expire if the arguments (`args` / `kwargs`) of the decorated function change.
- When a `os.PathLike` object is passed as an argument, the cache will expire if the file size and / or modification time changes.
- Code Change Detection: Cache will expire if the implementation / code of the decorated function changes during development.
- Time-based Expiry: Cache will expire when it is older than a given `timedelta`.
- In addition to the immutable built-in data types, the following types for arguments are supported:
- `os.PathLike`
- `pandas.DataFrame`
- `pandas.Series`
- `numpy.ndarray`
- `datetime.datetime`
- `types.FunctionType`
## Installation
Install federleicht from PyPI:
```cmd
pip install federleicht
```
Normally, `md5` is used for hashing the arguments, but for even faster hashing, you can try `xxhash` as an optional dependency:
```cmd
pip install federleicht[xxhash]
```
## Usage
Here's a quick example:
```python
import pandas as pd
from federleicht import cache_dataframe
@cache_dataframe
def generate_large_dataframe():
# Simulate a heavy computation
return pd.DataFrame({"col1": range(10000), "col2": range(10000)})
df = generate_large_dataframe()
```
## Benchmark
[](https://www.kaggle.com/datasets/alessandrolobello/the-ultimate-earthquake-dataset-from-1990-2023)
- **file**: Eartquakes-1990-2023.csv
- **size**: 494.8 mb
- **lines**: 3,445,752
Functions which are used to benchmark the performance of the `cache_dataframe` decorator.
```python
def read_data(file: str, **kwargs) -> pd.DataFrame:
"""
Read the earthquake dataset from a CSV file to Benchmark cache.
Perform some data type conversions and return the DataFrame.
"""
df = pd.read_csv(
file,
header=0,
dtype={
"status": "category",
"tsunami": "boolean",
"data_type": "category",
"state": "category",
},
**kwargs,
)
df["time"] = pd.to_datetime(df["time"], unit="ms")
df["date"] = pd.to_datetime(df["date"], format="mixed")
return df
```
The `pandas.DataFrame` without the `attrs` dictionary will be cached in the `.pandas_cache` directory and will only expire if the file changes. For more details, see the [Cache Expiry](#cache-expiry) section.
```python
@cache_dataframe
def read_cache(file: pathlib.Path, **kwargs) -> pd.DataFrame:
return read_data(file, **kwargs)
```
### Benchmark Results
Results strongly depend on the system configuration and the file system. The following results are obtained on:
- **OS**: Windows
- **OS Version**: 10.0.19044
- **Python**: 3.11.9
- **CPU**: AMD64 Family 23 Model 104 Stepping 1, AuthenticAMD
| nrows | read_data [s] | build_cache [s] | read_cache [s] |
| ------: | ------------: | --------------: | -------------: |
| 10000 | 0.060 | 0.076 | 0.037 |
| 32170 | 0.172 | 0.193 | 0.033 |
| 103493 | 0.536 | 0.569 | 0.067 |
| 332943 | 1.658 | 1.791 | 0.143 |
| 1071093 | 5.383 | 5.465 | 0.366 |
| 3445752 | 16.750 | 17.720 | 1.141 |
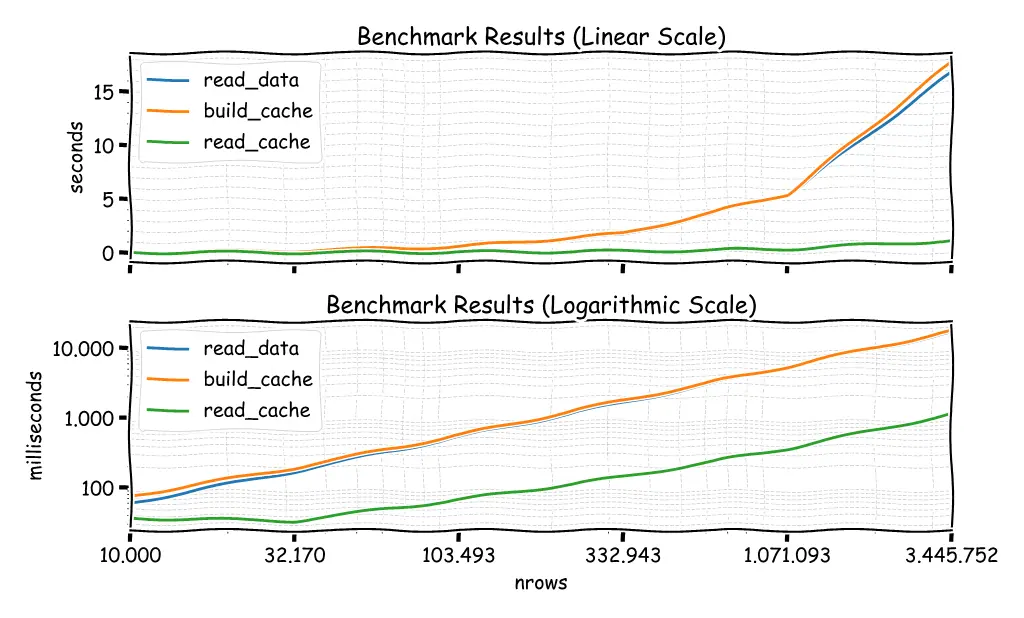
## Dependencies
[](https://pypi.org/project/pandas/)
[](https://pypi.org/project/pyarrow/)
[](https://pypi.org/project/xxhash/)
---
Raw data
{
"_id": null,
"home_page": null,
"name": "federleicht",
"maintainer": null,
"docs_url": null,
"requires_python": "<4.0,>=3.9",
"maintainer_email": null,
"keywords": "cache, feather, pandas, pyarrow",
"author": "Christoph D\u00f6rrer",
"author_email": "d-chris@web.de",
"download_url": "https://files.pythonhosted.org/packages/69/70/57b564168043706288d1d4d77201b427cde369dd171613eaef01c250a988/federleicht-0.1.0.tar.gz",
"platform": null,
"description": "# federleicht\n\n[](https://pypi.org/project/federleicht/)\n[](https://pypi.org/project/federleicht/)\n[](https://pypi.org/project/federleicht/)\n[](https://raw.githubusercontent.com/d-chris/federleicht/main/LICENSE)\n[](https://github.com/d-chris/federleicht/actions/workflows/pytest.yml)\n[](https://d-chris.github.io/federleicht)\n[](https://github.com/d-chris/federleicht)\n[](https://raw.githubusercontent.com/d-chris/federleicht/main/.pre-commit-config.yaml)\n[](https://codecov.io/gh/d-chris/federleicht)\n\n---\n\n`federleicht` is a Python package providing a cache decorator for `pandas.DataFrame`, utilizing the lightweight and efficient `pyarrow` feather file format.\n\n`federleicht.cache_dataframe` is designed to decorate functions that return `pandas.DataFrame` objects. The decorator saves the DataFrame to a feather file on the first call and loads it automatically on subsequent calls if the file exists.\n\n## Key Features\n\n- Feather Integration: Save and load `pandas.DataFrame` effortlessly using the Feather format, known for its speed and simplicity.\n- Decorator Simplicity: Add caching functionality to your functions with a single decorator line.\n- Efficient Caching: Avoid redundant computations by reusing cached results.\n\n## Cache Expiry\n\nTo implement cache expiry, `federleicht` requires all arguments of the decorated function to be serializable. The cache will expire under the following conditions:\n\n- Argument Sensitivity: Cache will expire if the arguments (`args` / `kwargs`) of the decorated function change.\n- When a `os.PathLike` object is passed as an argument, the cache will expire if the file size and / or modification time changes.\n- Code Change Detection: Cache will expire if the implementation / code of the decorated function changes during development.\n- Time-based Expiry: Cache will expire when it is older than a given `timedelta`.\n- In addition to the immutable built-in data types, the following types for arguments are supported:\n - `os.PathLike`\n - `pandas.DataFrame`\n - `pandas.Series`\n - `numpy.ndarray`\n - `datetime.datetime`\n - `types.FunctionType`\n\n## Installation\n\nInstall federleicht from PyPI:\n\n```cmd\npip install federleicht\n```\n\nNormally, `md5` is used for hashing the arguments, but for even faster hashing, you can try `xxhash` as an optional dependency:\n\n```cmd\npip install federleicht[xxhash]\n```\n\n## Usage\n\nHere's a quick example:\n\n```python\nimport pandas as pd\nfrom federleicht import cache_dataframe\n\n@cache_dataframe\ndef generate_large_dataframe():\n # Simulate a heavy computation\n return pd.DataFrame({\"col1\": range(10000), \"col2\": range(10000)})\n\ndf = generate_large_dataframe()\n```\n\n## Benchmark\n\n[](https://www.kaggle.com/datasets/alessandrolobello/the-ultimate-earthquake-dataset-from-1990-2023)\n\n- **file**: Eartquakes-1990-2023.csv\n- **size**: 494.8 mb\n- **lines**: 3,445,752\n\nFunctions which are used to benchmark the performance of the `cache_dataframe` decorator.\n\n```python\ndef read_data(file: str, **kwargs) -> pd.DataFrame:\n \"\"\"\n Read the earthquake dataset from a CSV file to Benchmark cache.\n\n Perform some data type conversions and return the DataFrame.\n \"\"\"\n df = pd.read_csv(\n file,\n header=0,\n dtype={\n \"status\": \"category\",\n \"tsunami\": \"boolean\",\n \"data_type\": \"category\",\n \"state\": \"category\",\n },\n **kwargs,\n )\n\n df[\"time\"] = pd.to_datetime(df[\"time\"], unit=\"ms\")\n df[\"date\"] = pd.to_datetime(df[\"date\"], format=\"mixed\")\n\n return df\n```\n\nThe `pandas.DataFrame` without the `attrs` dictionary will be cached in the `.pandas_cache` directory and will only expire if the file changes. For more details, see the [Cache Expiry](#cache-expiry) section.\n\n```python\n@cache_dataframe\ndef read_cache(file: pathlib.Path, **kwargs) -> pd.DataFrame:\n return read_data(file, **kwargs)\n```\n\n### Benchmark Results\n\nResults strongly depend on the system configuration and the file system. The following results are obtained on:\n\n- **OS**: Windows\n- **OS Version**: 10.0.19044\n- **Python**: 3.11.9\n- **CPU**: AMD64 Family 23 Model 104 Stepping 1, AuthenticAMD\n\n| nrows | read_data [s] | build_cache [s] | read_cache [s] |\n| ------: | ------------: | --------------: | -------------: |\n| 10000 | 0.060 | 0.076 | 0.037 |\n| 32170 | 0.172 | 0.193 | 0.033 |\n| 103493 | 0.536 | 0.569 | 0.067 |\n| 332943 | 1.658 | 1.791 | 0.143 |\n| 1071093 | 5.383 | 5.465 | 0.366 |\n| 3445752 | 16.750 | 17.720 | 1.141 |\n\n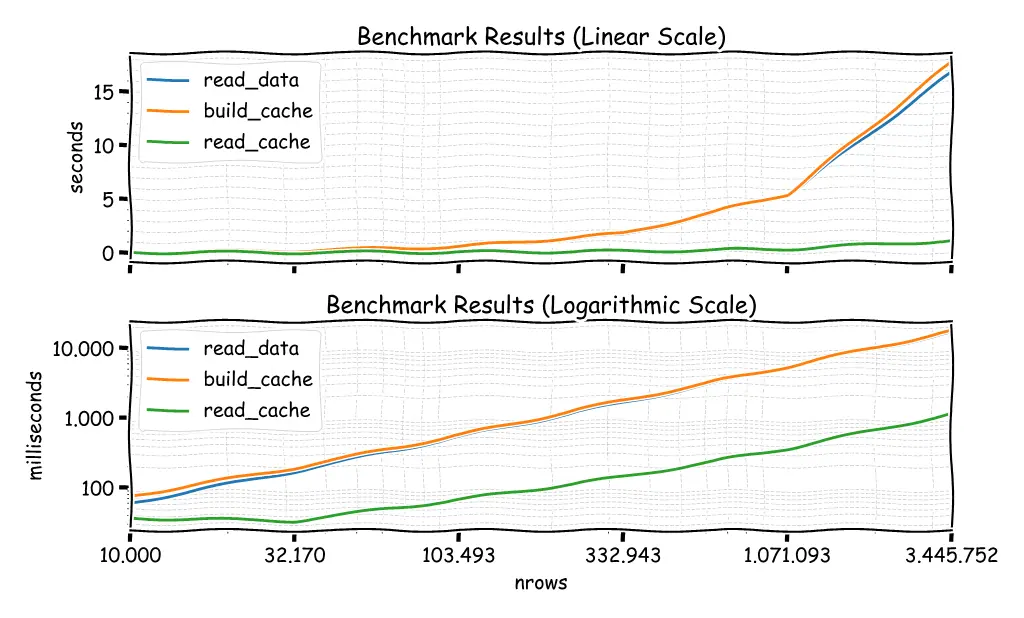\n\n## Dependencies\n\n[](https://pypi.org/project/pandas/)\n[](https://pypi.org/project/pyarrow/)\n[](https://pypi.org/project/xxhash/)\n\n---\n",
"bugtrack_url": null,
"license": "MIT",
"summary": "lightweigth function decorators to cache your `pandas.DataFrame` as feather.",
"version": "0.1.0",
"project_urls": {
"Documentation": "https://d-chris.github.io/federleicht",
"Repository": "https://github.com/d-chris/federleicht"
},
"split_keywords": [
"cache",
" feather",
" pandas",
" pyarrow"
],
"urls": [
{
"comment_text": "",
"digests": {
"blake2b_256": "bf5523bb0bc9f70aed130442b29c68eed24fc9bb51f2ee9e3f84c92157ffbfbb",
"md5": "1b9526441622a3a78f765baba1278b67",
"sha256": "ce8bb9c83444d104e3f9e2ee04010ffb87885efbb8cbc7be249efd0e405e3d1c"
},
"downloads": -1,
"filename": "federleicht-0.1.0-py3-none-any.whl",
"has_sig": false,
"md5_digest": "1b9526441622a3a78f765baba1278b67",
"packagetype": "bdist_wheel",
"python_version": "py3",
"requires_python": "<4.0,>=3.9",
"size": 11288,
"upload_time": "2024-12-01T17:00:18",
"upload_time_iso_8601": "2024-12-01T17:00:18.349398Z",
"url": "https://files.pythonhosted.org/packages/bf/55/23bb0bc9f70aed130442b29c68eed24fc9bb51f2ee9e3f84c92157ffbfbb/federleicht-0.1.0-py3-none-any.whl",
"yanked": false,
"yanked_reason": null
},
{
"comment_text": "",
"digests": {
"blake2b_256": "697057b564168043706288d1d4d77201b427cde369dd171613eaef01c250a988",
"md5": "837f1ad8a539edd7ed415939b3da2cda",
"sha256": "ebb4ede1f9f13a210a85711f20df7001e0f0d3531bf4ff84c71c34851cb68995"
},
"downloads": -1,
"filename": "federleicht-0.1.0.tar.gz",
"has_sig": false,
"md5_digest": "837f1ad8a539edd7ed415939b3da2cda",
"packagetype": "sdist",
"python_version": "source",
"requires_python": "<4.0,>=3.9",
"size": 11059,
"upload_time": "2024-12-01T17:00:19",
"upload_time_iso_8601": "2024-12-01T17:00:19.974339Z",
"url": "https://files.pythonhosted.org/packages/69/70/57b564168043706288d1d4d77201b427cde369dd171613eaef01c250a988/federleicht-0.1.0.tar.gz",
"yanked": false,
"yanked_reason": null
}
],
"upload_time": "2024-12-01 17:00:19",
"github": true,
"gitlab": false,
"bitbucket": false,
"codeberg": false,
"github_user": "d-chris",
"github_project": "federleicht",
"travis_ci": false,
"coveralls": false,
"github_actions": true,
"tox": true,
"lcname": "federleicht"
}