Name | graph2mat JSON |
Version |
0.0.6
JSON |
| download |
home_page | None |
Summary | Utility package to work with equivariant matrices and graphs. |
upload_time | 2024-08-18 17:47:49 |
maintainer | None |
docs_url | None |
author | None |
requires_python | >=3.9 |
license | MIT |
keywords |
machine learning
equivariance
e3nn
matrix
|
VCS |
|
bugtrack_url |
|
requirements |
No requirements were recorded.
|
Travis-CI |
No Travis.
|
coveralls test coverage |
No coveralls.
|
graph2mat: Equivariant matrices meet machine learning
----------------------

The aim of `graph2mat` is to pave your way into meaningful science by providing the **tools to interface to common machine learning frameworks** (e3nn, pytorch) **to learn equivariant matrices.**
**[Documentation](https://big-map.github.io/graph2mat/)**
It also provides a **set of tools** to facilitate the training and usage of the models created using the package:
- **Training tools**: It contains custom `pytorch_lightning` modules to train, validate and test the orbital matrix models.
- **Server**: A production ready server (and client) to serve predictions of the trained
models. Implemented using `fastapi`.
- **Siesta**: A set of tools to interface the machine learning models with SIESTA. These include tools for input preparation, analysis of performance...
The package also implements a **command line interface** (CLI): `graph2mat`. The aim of this CLI is
to make the usage of `graph2mat`'s tools as simple as possible. It has two objectives:
- Make life easy for the model developers.
- Facilitate the usage of the models by non machine learning scientists, who just want
good predictions for their systems.
Installation
------------
It can be installed with pip. Adding the tools extra will also install all the dependencies
needed to use the tools provided.
```
pip install graph2mat[tools]
```
If you want to use `graph2mat` with e3nn you can also ask for the `e3nn` extra dependencies:
```
pip install graph2mat[tools,e3nn]
```
You can also ask for
What is an equivariant matrix?
------------------------------
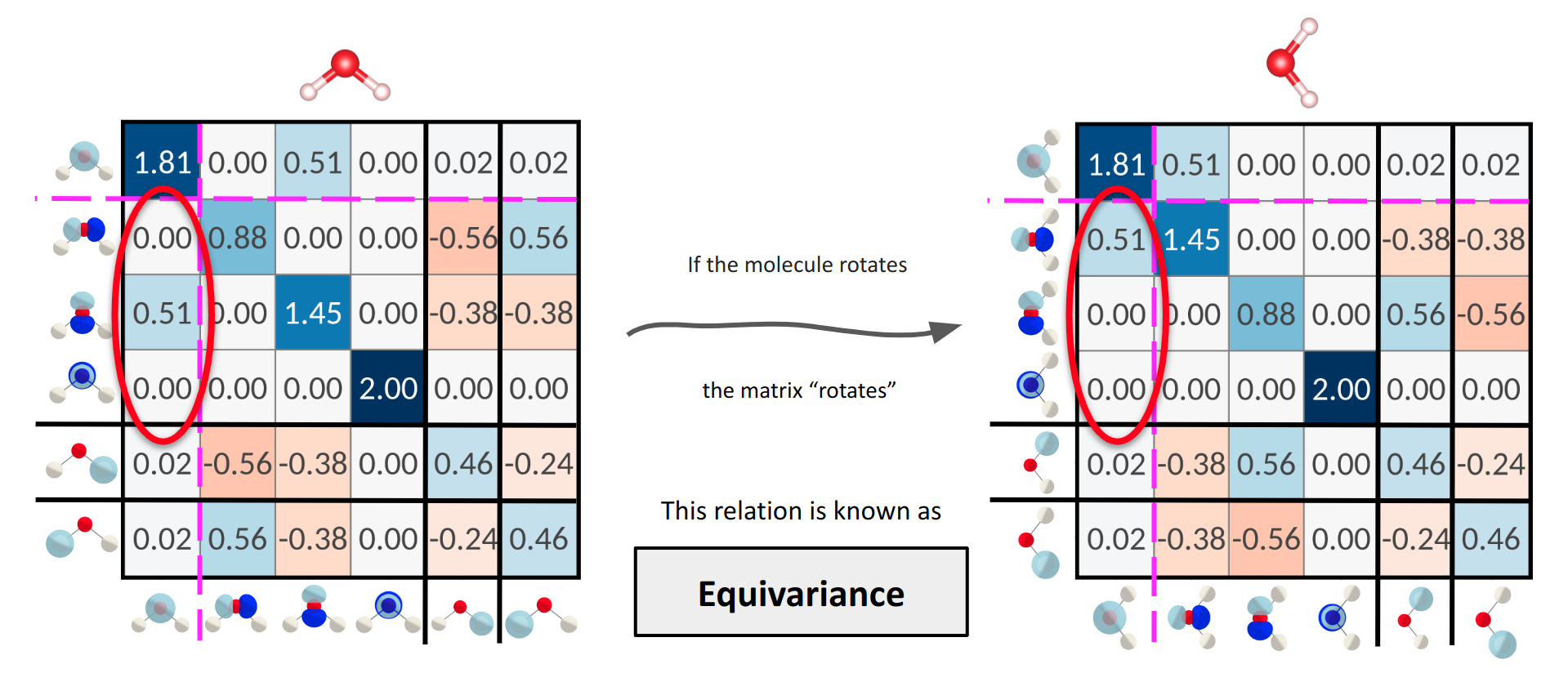
Contributions
--------------
We are very open to suggestions, contributions, discussions...
- If you have questions or want do discuss an idea, please [start a discussion](https://github.com/BIG-MAP/graph2mat/discussions)
- If you have a feature suggestion or bug report, please [open an issue](https://github.com/BIG-MAP/graph2mat/issues)
We look forward to your contributions!
Raw data
{
"_id": null,
"home_page": null,
"name": "graph2mat",
"maintainer": null,
"docs_url": null,
"requires_python": ">=3.9",
"maintainer_email": null,
"keywords": "machine learning, equivariance, e3nn, matrix",
"author": null,
"author_email": "Pol Febrer <pfebrer96@gmail.com>, =?utf-8?q?Peter_B=2E_J=C3=B8rgensen?= <peterbjorgensen@gmail.com>",
"download_url": "https://files.pythonhosted.org/packages/29/d2/12b5ebab58849bb0af6dcbcdae5e1e70b67e2d3d686ef11953abc3f14d3b/graph2mat-0.0.6.tar.gz",
"platform": null,
"description": "graph2mat: Equivariant matrices meet machine learning\n----------------------\n\n\n\nThe aim of `graph2mat` is to pave your way into meaningful science by providing the **tools to interface to common machine learning frameworks** (e3nn, pytorch) **to learn equivariant matrices.**\n\n**[Documentation](https://big-map.github.io/graph2mat/)**\n\nIt also provides a **set of tools** to facilitate the training and usage of the models created using the package:\n\n- **Training tools**: It contains custom `pytorch_lightning` modules to train, validate and test the orbital matrix models.\n- **Server**: A production ready server (and client) to serve predictions of the trained\n models. Implemented using `fastapi`.\n- **Siesta**: A set of tools to interface the machine learning models with SIESTA. These include tools for input preparation, analysis of performance...\n\nThe package also implements a **command line interface** (CLI): `graph2mat`. The aim of this CLI is\nto make the usage of `graph2mat`'s tools as simple as possible. It has two objectives:\n\n- Make life easy for the model developers.\n- Facilitate the usage of the models by non machine learning scientists, who just want\n good predictions for their systems.\n\nInstallation\n------------\n\nIt can be installed with pip. Adding the tools extra will also install all the dependencies\nneeded to use the tools provided.\n\n```\npip install graph2mat[tools]\n```\n\nIf you want to use `graph2mat` with e3nn you can also ask for the `e3nn` extra dependencies:\n\n```\npip install graph2mat[tools,e3nn]\n```\n\nYou can also ask for\n\nWhat is an equivariant matrix?\n------------------------------\n\n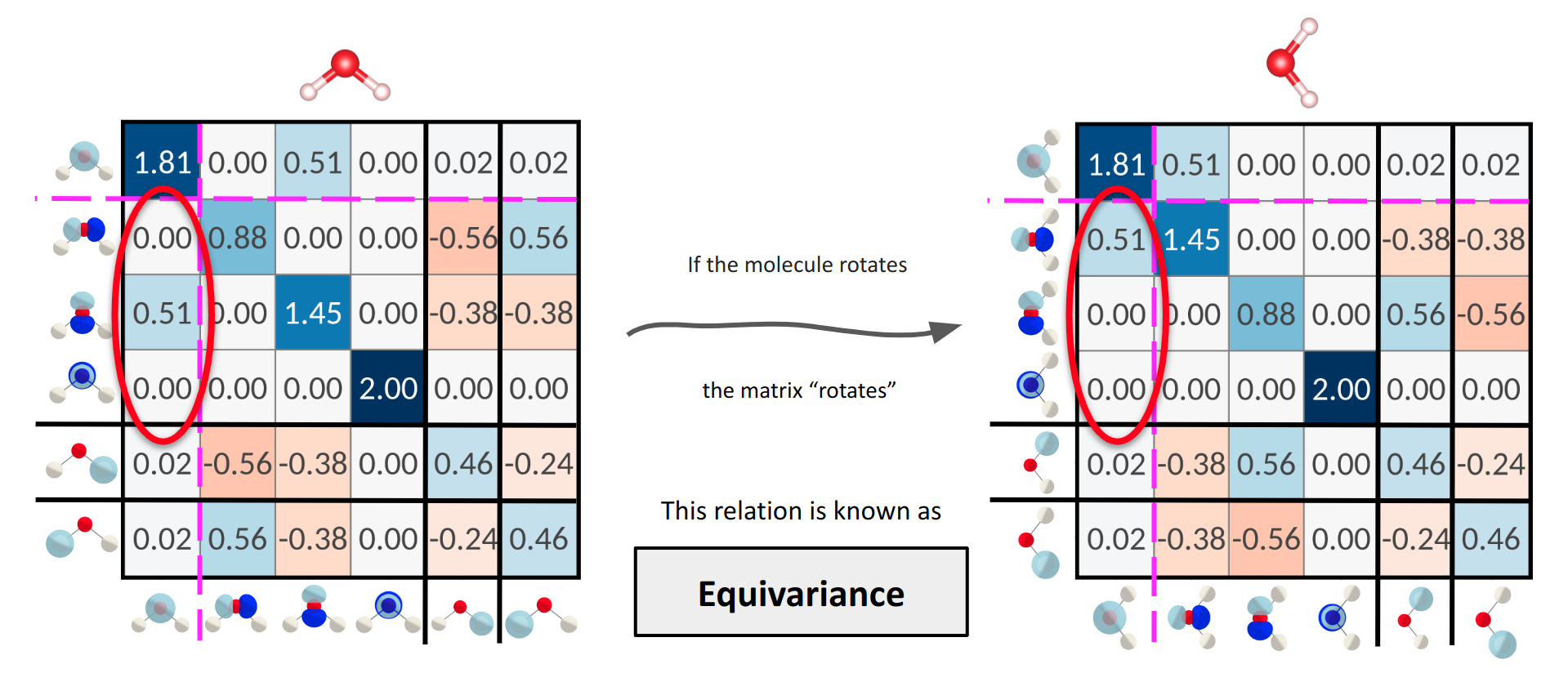\n\n\nContributions\n--------------\n\nWe are very open to suggestions, contributions, discussions...\n\n- If you have questions or want do discuss an idea, please [start a discussion](https://github.com/BIG-MAP/graph2mat/discussions)\n- If you have a feature suggestion or bug report, please [open an issue](https://github.com/BIG-MAP/graph2mat/issues)\n\nWe look forward to your contributions!\n",
"bugtrack_url": null,
"license": "MIT",
"summary": "Utility package to work with equivariant matrices and graphs.",
"version": "0.0.6",
"project_urls": null,
"split_keywords": [
"machine learning",
" equivariance",
" e3nn",
" matrix"
],
"urls": [
{
"comment_text": "",
"digests": {
"blake2b_256": "29d212b5ebab58849bb0af6dcbcdae5e1e70b67e2d3d686ef11953abc3f14d3b",
"md5": "09175b41c838b7991c9521b0541fa8cb",
"sha256": "5d1dc9b257dddbdbf988b0975024f4d700273b91ad4d6c2971f4bcc451763891"
},
"downloads": -1,
"filename": "graph2mat-0.0.6.tar.gz",
"has_sig": false,
"md5_digest": "09175b41c838b7991c9521b0541fa8cb",
"packagetype": "sdist",
"python_version": "source",
"requires_python": ">=3.9",
"size": 1123527,
"upload_time": "2024-08-18T17:47:49",
"upload_time_iso_8601": "2024-08-18T17:47:49.001735Z",
"url": "https://files.pythonhosted.org/packages/29/d2/12b5ebab58849bb0af6dcbcdae5e1e70b67e2d3d686ef11953abc3f14d3b/graph2mat-0.0.6.tar.gz",
"yanked": false,
"yanked_reason": null
}
],
"upload_time": "2024-08-18 17:47:49",
"github": false,
"gitlab": false,
"bitbucket": false,
"codeberg": false,
"lcname": "graph2mat"
}