Name | lit-nlp JSON |
Version |
1.2
JSON |
| download |
home_page | None |
Summary | 🔥LIT: The Learning Interpretability Tool |
upload_time | 2024-06-26 16:32:41 |
maintainer | None |
docs_url | None |
author | None |
requires_python | >=3.9 |
license | Apache License Version 2.0, January 2004 http://www.apache.org/licenses/ TERMS AND CONDITIONS FOR USE, REPRODUCTION, AND DISTRIBUTION 1. Definitions. "License" shall mean the terms and conditions for use, reproduction, and distribution as defined by Sections 1 through 9 of this document. "Licensor" shall mean the copyright owner or entity authorized by the copyright owner that is granting the License. "Legal Entity" shall mean the union of the acting entity and all other entities that control, are controlled by, or are under common control with that entity. For the purposes of this definition, "control" means (i) the power, direct or indirect, to cause the direction or management of such entity, whether by contract or otherwise, or (ii) ownership of fifty percent (50%) or more of the outstanding shares, or (iii) beneficial ownership of such entity. "You" (or "Your") shall mean an individual or Legal Entity exercising permissions granted by this License. "Source" form shall mean the preferred form for making modifications, including but not limited to software source code, documentation source, and configuration files. "Object" form shall mean any form resulting from mechanical transformation or translation of a Source form, including but not limited to compiled object code, generated documentation, and conversions to other media types. "Work" shall mean the work of authorship, whether in Source or Object form, made available under the License, as indicated by a copyright notice that is included in or attached to the work (an example is provided in the Appendix below). "Derivative Works" shall mean any work, whether in Source or Object form, that is based on (or derived from) the Work and for which the editorial revisions, annotations, elaborations, or other modifications represent, as a whole, an original work of authorship. For the purposes of this License, Derivative Works shall not include works that remain separable from, or merely link (or bind by name) to the interfaces of, the Work and Derivative Works thereof. "Contribution" shall mean any work of authorship, including the original version of the Work and any modifications or additions to that Work or Derivative Works thereof, that is intentionally submitted to Licensor for inclusion in the Work by the copyright owner or by an individual or Legal Entity authorized to submit on behalf of the copyright owner. For the purposes of this definition, "submitted" means any form of electronic, verbal, or written communication sent to the Licensor or its representatives, including but not limited to communication on electronic mailing lists, source code control systems, and issue tracking systems that are managed by, or on behalf of, the Licensor for the purpose of discussing and improving the Work, but excluding communication that is conspicuously marked or otherwise designated in writing by the copyright owner as "Not a Contribution." "Contributor" shall mean Licensor and any individual or Legal Entity on behalf of whom a Contribution has been received by Licensor and subsequently incorporated within the Work. 2. Grant of Copyright License. Subject to the terms and conditions of this License, each Contributor hereby grants to You a perpetual, worldwide, non-exclusive, no-charge, royalty-free, irrevocable copyright license to reproduce, prepare Derivative Works of, publicly display, publicly perform, sublicense, and distribute the Work and such Derivative Works in Source or Object form. 3. Grant of Patent License. Subject to the terms and conditions of this License, each Contributor hereby grants to You a perpetual, worldwide, non-exclusive, no-charge, royalty-free, irrevocable (except as stated in this section) patent license to make, have made, use, offer to sell, sell, import, and otherwise transfer the Work, where such license applies only to those patent claims licensable by such Contributor that are necessarily infringed by their Contribution(s) alone or by combination of their Contribution(s) with the Work to which such Contribution(s) was submitted. If You institute patent litigation against any entity (including a cross-claim or counterclaim in a lawsuit) alleging that the Work or a Contribution incorporated within the Work constitutes direct or contributory patent infringement, then any patent licenses granted to You under this License for that Work shall terminate as of the date such litigation is filed. 4. Redistribution. You may reproduce and distribute copies of the Work or Derivative Works thereof in any medium, with or without modifications, and in Source or Object form, provided that You meet the following conditions: (a) You must give any other recipients of the Work or Derivative Works a copy of this License; and (b) You must cause any modified files to carry prominent notices stating that You changed the files; and (c) You must retain, in the Source form of any Derivative Works that You distribute, all copyright, patent, trademark, and attribution notices from the Source form of the Work, excluding those notices that do not pertain to any part of the Derivative Works; and (d) If the Work includes a "NOTICE" text file as part of its distribution, then any Derivative Works that You distribute must include a readable copy of the attribution notices contained within such NOTICE file, excluding those notices that do not pertain to any part of the Derivative Works, in at least one of the following places: within a NOTICE text file distributed as part of the Derivative Works; within the Source form or documentation, if provided along with the Derivative Works; or, within a display generated by the Derivative Works, if and wherever such third-party notices normally appear. The contents of the NOTICE file are for informational purposes only and do not modify the License. You may add Your own attribution notices within Derivative Works that You distribute, alongside or as an addendum to the NOTICE text from the Work, provided that such additional attribution notices cannot be construed as modifying the License. You may add Your own copyright statement to Your modifications and may provide additional or different license terms and conditions for use, reproduction, or distribution of Your modifications, or for any such Derivative Works as a whole, provided Your use, reproduction, and distribution of the Work otherwise complies with the conditions stated in this License. 5. Submission of Contributions. Unless You explicitly state otherwise, any Contribution intentionally submitted for inclusion in the Work by You to the Licensor shall be under the terms and conditions of this License, without any additional terms or conditions. Notwithstanding the above, nothing herein shall supersede or modify the terms of any separate license agreement you may have executed with Licensor regarding such Contributions. 6. Trademarks. This License does not grant permission to use the trade names, trademarks, service marks, or product names of the Licensor, except as required for reasonable and customary use in describing the origin of the Work and reproducing the content of the NOTICE file. 7. Disclaimer of Warranty. Unless required by applicable law or agreed to in writing, Licensor provides the Work (and each Contributor provides its Contributions) on an "AS IS" BASIS, WITHOUT WARRANTIES OR CONDITIONS OF ANY KIND, either express or implied, including, without limitation, any warranties or conditions of TITLE, NON-INFRINGEMENT, MERCHANTABILITY, or FITNESS FOR A PARTICULAR PURPOSE. You are solely responsible for determining the appropriateness of using or redistributing the Work and assume any risks associated with Your exercise of permissions under this License. 8. Limitation of Liability. In no event and under no legal theory, whether in tort (including negligence), contract, or otherwise, unless required by applicable law (such as deliberate and grossly negligent acts) or agreed to in writing, shall any Contributor be liable to You for damages, including any direct, indirect, special, incidental, or consequential damages of any character arising as a result of this License or out of the use or inability to use the Work (including but not limited to damages for loss of goodwill, work stoppage, computer failure or malfunction, or any and all other commercial damages or losses), even if such Contributor has been advised of the possibility of such damages. 9. Accepting Warranty or Additional Liability. While redistributing the Work or Derivative Works thereof, You may choose to offer, and charge a fee for, acceptance of support, warranty, indemnity, or other liability obligations and/or rights consistent with this License. However, in accepting such obligations, You may act only on Your own behalf and on Your sole responsibility, not on behalf of any other Contributor, and only if You agree to indemnify, defend, and hold each Contributor harmless for any liability incurred by, or claims asserted against, such Contributor by reason of your accepting any such warranty or additional liability. END OF TERMS AND CONDITIONS APPENDIX: How to apply the Apache License to your work. To apply the Apache License to your work, attach the following boilerplate notice, with the fields enclosed by brackets "[]" replaced with your own identifying information. (Don't include the brackets!) The text should be enclosed in the appropriate comment syntax for the file format. We also recommend that a file or class name and description of purpose be included on the same "printed page" as the copyright notice for easier identification within third-party archives. Copyright [yyyy] [name of copyright owner] Licensed under the Apache License, Version 2.0 (the "License"); you may not use this file except in compliance with the License. You may obtain a copy of the License at http://www.apache.org/licenses/LICENSE-2.0 Unless required by applicable law or agreed to in writing, software distributed under the License is distributed on an "AS IS" BASIS, WITHOUT WARRANTIES OR CONDITIONS OF ANY KIND, either express or implied. See the License for the specific language governing permissions and limitations under the License. |
keywords |
interpretability
interpretable artifical intelligence
explainable
explainable artifical intelligence
xai
computer vision
natural language processing
artifical intelligence
machine learning
deep learning
visualization
visual analytics
tensorflow
tf
torch
|
VCS |
 |
bugtrack_url |
|
requirements |
No requirements were recorded.
|
Travis-CI |
No Travis.
|
coveralls test coverage |
No coveralls.
|
# 🔥 Learning Interpretability Tool (LIT)
<!--* freshness: { owner: 'lit-dev' reviewed: '2024-06-25' } *-->
The Learning Interpretability Tool (🔥LIT, formerly known as the Language
Interpretability Tool) is a visual, interactive ML model-understanding tool that
supports text, image, and tabular data. It can be run as a standalone server, or
inside of notebook environments such as Colab, Jupyter, and Google Cloud Vertex
AI notebooks.
LIT is built to answer questions such as:
* **What kind of examples** does my model perform poorly on?
* **Why did my model make this prediction?** Can this prediction be attributed
to adversarial behavior, or to undesirable priors in the training set?
* **Does my model behave consistently** if I change things like textual style,
verb tense, or pronoun gender?
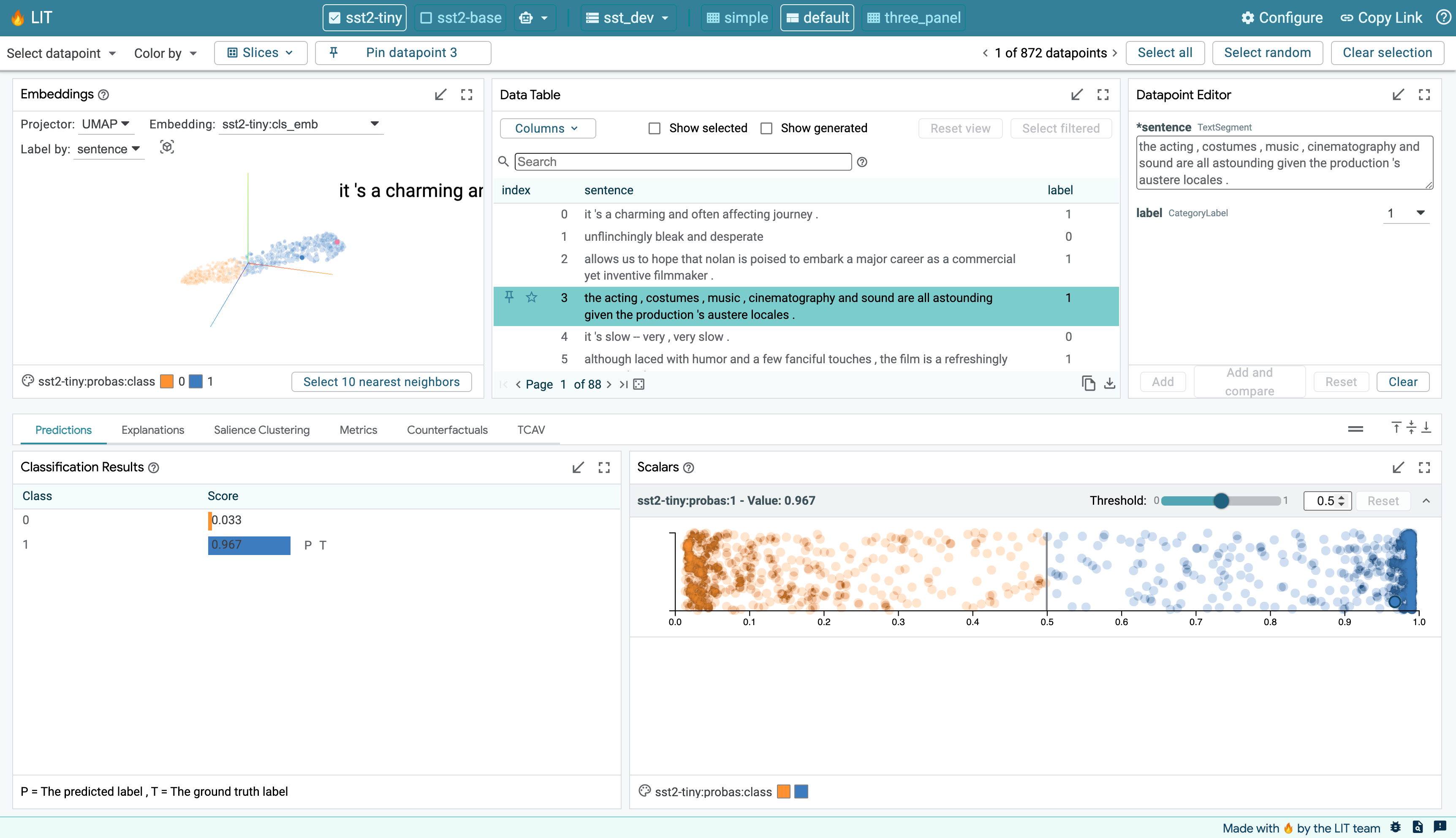
LIT supports a variety of debugging workflows through a browser-based UI.
Features include:
* **Local explanations** via salience maps and rich visualization of model
predictions.
* **Aggregate analysis** including custom metrics, slicing and binning, and
visualization of embedding spaces.
* **Counterfactual generation** via manual edits or generator plug-ins to
dynamically create and evaluate new examples.
* **Side-by-side mode** to compare two or more models, or one model on a pair
of examples.
* **Highly extensible** to new model types, including classification,
regression, span labeling, seq2seq, and language modeling. Supports
multi-head models and multiple input features out of the box.
* **Framework-agnostic** and compatible with TensorFlow, PyTorch, and more.
LIT has a [website](https://pair-code.github.io/lit) with live demos, tutorials,
a setup guide and more.
Stay up to date on LIT by joining the
[lit-announcements mailing list](https://groups.google.com/g/lit-annoucements).
For a broader overview, check out [our paper](https://arxiv.org/abs/2008.05122) and the
[user guide](https://pair-code.github.io/lit/documentation/ui_guide).
## Documentation
* [Documentation index](https://pair-code.github.io/lit/documentation/)
* [FAQ](https://pair-code.github.io/lit/documentation/faq)
* [Release notes](./RELEASE.md)
## Download and Installation
LIT can be run via container image, installed via `pip` or built from source.
Building from source is necessary if you update any of the front-end or core
back-end code.
### Build container image
Build the image using `docker` or `podman`:
```sh
git clone https://github.com/PAIR-code/lit.git && cd lit
docker build --file Dockerfile --tag lit-nlp .
```
See the [advanced guide](https://pair-code.github.io/lit/documentation/docker) for detailed instructions on using the
default LIT Docker image, running LIT as a containerized web app in different
scenarios, and how to creating your own LIT images.
### pip installation
```sh
pip install lit-nlp
```
The `pip` installation will install all necessary prerequisite packages for use
of the core LIT package.
It **does not** install the prerequisites for the provided demos, so you need to
install those yourself. See
[requirements_examples.txt](./requirements_examples.txt) for the list of
packages required to run the demos.
### Install from source
Clone the repo:
```sh
git clone https://github.com/PAIR-code/lit.git && cd lit
```
Note: be sure you are running Python 3.9+. If you have a different version on
your system, use the `conda` instructions below to set up a Python 3.9
environment.
Set up a Python environment with `venv`:
```sh
python -m venv .venv
source .venv/bin/activate
```
Or set up a Python environment using `conda`:
```sh
conda create --name lit-nlp
conda activate lit-nlp
conda install python=3.9
conda install pip
```
Once you have the environment, install LIT's dependencies:
```sh
python -m pip install -r requirements.txt
python -m pip install cudnn cupti # optional, for GPU support
python -m pip install torch # optional, for PyTorch
# Build the frontend
(cd lit_nlp; yarn && yarn build)
```
Note: Use the `-r requirements.txt` option to install every dependency required
for the LIT library, its test suite, and the built-in examples. You can also
install subsets of these using the `-r requirements_core.txt` (core library),
`-r requirements_test.txt` (test suite), `-r requirements_examples.txt`
(examples), and/or any combination thereof.
Note: if you see [an error](https://github.com/yarnpkg/yarn/issues/2821)
running `yarn` on Ubuntu/Debian, be sure you have the
[correct version installed](https://yarnpkg.com/en/docs/install#linux-tab).
## Running LIT
Explore a collection of hosted demos on the
[demos page](https://pair-code.github.io/lit/demos).
### Quick-start: classification and regression
To explore classification and regression models tasks from the popular
[GLUE benchmark](https://gluebenchmark.com/):
```sh
python -m lit_nlp.examples.glue.demo --port=5432 --quickstart
```
Navigate to http://localhost:5432 to access the LIT UI.
Your default view will be a
[small BERT-based model](https://arxiv.org/abs/1908.08962) fine-tuned on the
[Stanford Sentiment Treebank](https://nlp.stanford.edu/sentiment/treebank.html),
but you can switch to
[STS-B](http://ixa2.si.ehu.es/stswiki/index.php/STSbenchmark) or
[MultiNLI](https://cims.nyu.edu/~sbowman/multinli/) using the toolbar or the
gear icon in the upper right.
```
And navigate to http://localhost:5432 for the UI.
### Notebook usage
Colab notebooks showing the use of LIT inside of notebooks can be found at
[lit_nlp/examples/notebooks](./lit_nlp/examples/notebooks).
We provide a simple
[Colab demo](https://colab.research.google.com/github/PAIR-code/lit/blob/main/lit_nlp/examples/notebooks/LIT_sentiment_classifier.ipynb).
Run all the cells to see LIT on an example classification model in the notebook.
### More Examples
See [lit_nlp/examples](./lit_nlp/examples). Most are run similarly to the
quickstart example above:
```sh
python -m lit_nlp.examples.<example_name>.demo --port=5432 [optional --args]
```
## User Guide
To learn about LIT's features, check out the [user guide](https://pair-code.github.io/lit/documentation/ui_guide), or
watch this [video](https://www.youtube.com/watch?v=CuRI_VK83dU).
## Adding your own models or data
You can easily run LIT with your own model by creating a custom `demo.py`
launcher, similar to those in [lit_nlp/examples](./lit_nlp/examples). The
basic steps are:
* Write a data loader which follows the [`Dataset` API](https://pair-code.github.io/lit/documentation/api#datasets)
* Write a model wrapper which follows the [`Model` API](https://pair-code.github.io/lit/documentation/api#models)
* Pass models, datasets, and any additional
[components](https://pair-code.github.io/lit/documentation/api#interpretation-components) to the LIT server class
For a full walkthrough, see
[adding models and data](https://pair-code.github.io/lit/documentation/api#adding-models-and-data).
## Extending LIT with new components
LIT is easy to extend with new interpretability components, generators, and
more, both on the frontend or the backend. See our [documentation](https://pair-code.github.io/lit/documentation/) to get
started.
## Pull Request Process
To make code changes to LIT, please work off of the `dev` branch and
[create pull requests](https://docs.github.com/en/pull-requests/collaborating-with-pull-requests/proposing-changes-to-your-work-with-pull-requests/creating-a-pull-request)
(PRs) against that branch. The `main` branch is for stable releases, and it is
expected that the `dev` branch will always be ahead of `main`.
[Draft PRs](https://github.blog/2019-02-14-introducing-draft-pull-requests/) are
encouraged, especially for first-time contributors or contributors working on
complex tasks (e.g., Google Summer of Code contributors). Please use these to
communicate ideas and implementations with the LIT team, in addition to issues.
Prior to sending your PR or marking a Draft PR as "Ready for Review", please run
the Python and TypeScript linters on your code to ensure compliance with
Google's [Python](https://google.github.io/styleguide/pyguide.html) and
[TypeScript](https://google.github.io/styleguide/tsguide.html) Style Guides.
```sh
# Run Pylint on your code using the following command from the root of this repo
pushd lit_nlp & pylint & popd
# Run ESLint on your code using the following command from the root of this repo
pushd lit_nlp & yarn lint & popd
```
## Citing LIT
If you use LIT as part of your work, please cite
[our EMNLP paper](https://arxiv.org/abs/2008.05122):
```
@misc{tenney2020language,
title={The Language Interpretability Tool: Extensible, Interactive Visualizations and Analysis for {NLP} Models},
author={Ian Tenney and James Wexler and Jasmijn Bastings and Tolga Bolukbasi and Andy Coenen and Sebastian Gehrmann and Ellen Jiang and Mahima Pushkarna and Carey Radebaugh and Emily Reif and Ann Yuan},
booktitle = "Proceedings of the 2020 Conference on Empirical Methods in Natural Language Processing: System Demonstrations",
year = "2020",
publisher = "Association for Computational Linguistics",
pages = "107--118",
url = "https://www.aclweb.org/anthology/2020.emnlp-demos.15",
}
```
## Disclaimer
This is not an official Google product.
LIT is a research project and under active development by a small team. There
will be some bugs and rough edges, but we're releasing at an early stage because
we think it's pretty useful already. We want LIT to be an open platform, not a
walled garden, and we would love your suggestions and feedback - drop us a line
in the [issues](https://github.com/pair-code/lit/issues).
Raw data
{
"_id": null,
"home_page": null,
"name": "lit-nlp",
"maintainer": null,
"docs_url": null,
"requires_python": ">=3.9",
"maintainer_email": null,
"keywords": "interpretability, interpretable artifical intelligence, explainable, explainable artifical intelligence, XAI, computer vision, natural language processing, artifical intelligence, machine learning, deep learning, visualization, visual analytics, tensorflow, tf, torch",
"author": null,
"author_email": "\"Google, LLC\" <lit-dev@google.com>",
"download_url": "https://files.pythonhosted.org/packages/d1/1e/ac01a605a49e54298f9cb0c2060a23504efcf238838d6228360a66aafcd1/lit_nlp-1.2.tar.gz",
"platform": null,
"description": "# \ud83d\udd25 Learning Interpretability Tool (LIT)\n\n<!--* freshness: { owner: 'lit-dev' reviewed: '2024-06-25' } *-->\n\nThe Learning Interpretability Tool (\ud83d\udd25LIT, formerly known as the Language\nInterpretability Tool) is a visual, interactive ML model-understanding tool that\nsupports text, image, and tabular data. It can be run as a standalone server, or\ninside of notebook environments such as Colab, Jupyter, and Google Cloud Vertex\nAI notebooks.\n\nLIT is built to answer questions such as:\n\n* **What kind of examples** does my model perform poorly on?\n* **Why did my model make this prediction?** Can this prediction be attributed\n to adversarial behavior, or to undesirable priors in the training set?\n* **Does my model behave consistently** if I change things like textual style,\n verb tense, or pronoun gender?\n\n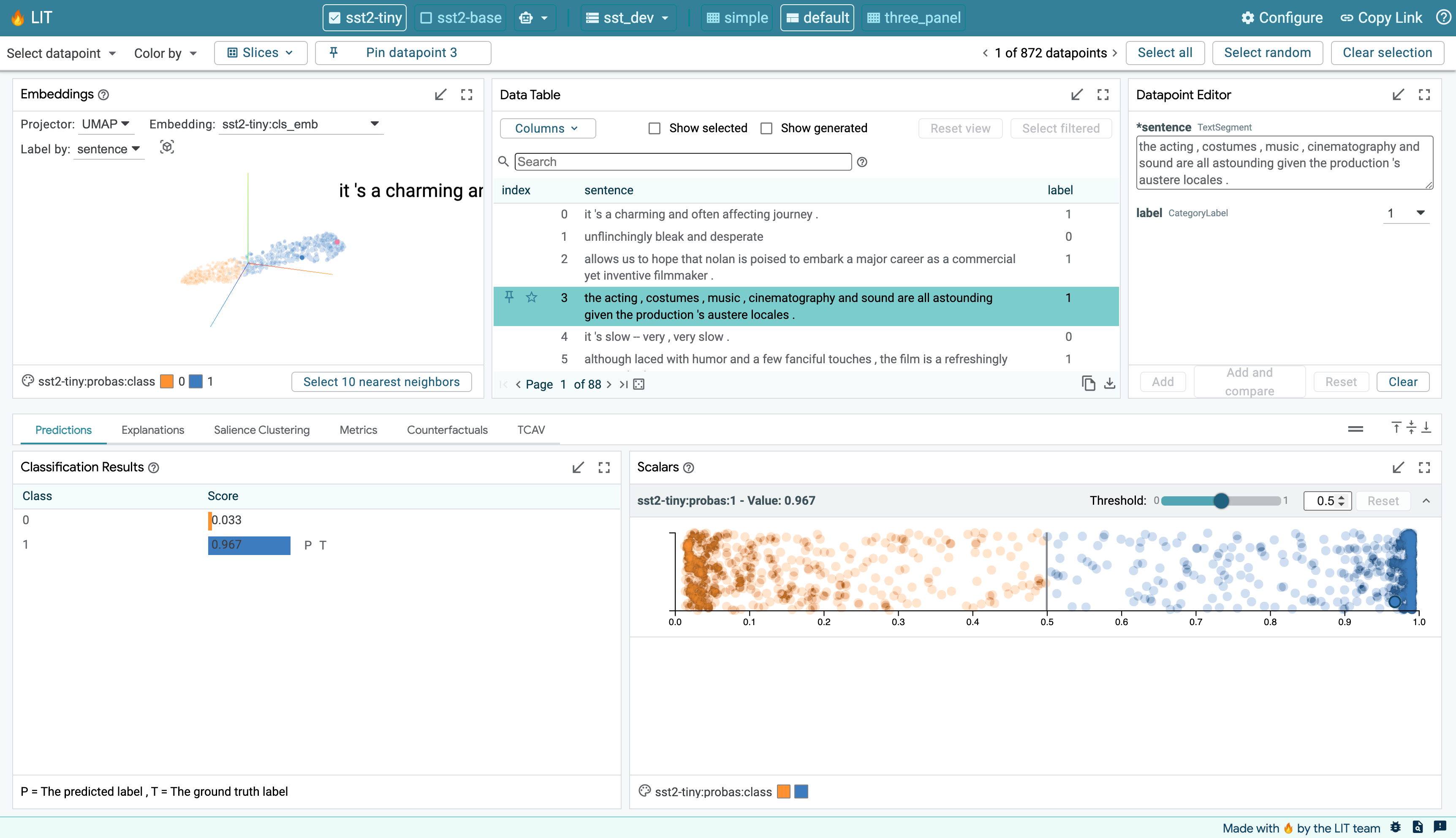\n\nLIT supports a variety of debugging workflows through a browser-based UI.\nFeatures include:\n\n* **Local explanations** via salience maps and rich visualization of model\n predictions.\n* **Aggregate analysis** including custom metrics, slicing and binning, and\n visualization of embedding spaces.\n* **Counterfactual generation** via manual edits or generator plug-ins to\n dynamically create and evaluate new examples.\n* **Side-by-side mode** to compare two or more models, or one model on a pair\n of examples.\n* **Highly extensible** to new model types, including classification,\n regression, span labeling, seq2seq, and language modeling. Supports\n multi-head models and multiple input features out of the box.\n* **Framework-agnostic** and compatible with TensorFlow, PyTorch, and more.\n\nLIT has a [website](https://pair-code.github.io/lit) with live demos, tutorials,\na setup guide and more.\n\nStay up to date on LIT by joining the\n[lit-announcements mailing list](https://groups.google.com/g/lit-annoucements).\n\nFor a broader overview, check out [our paper](https://arxiv.org/abs/2008.05122) and the\n[user guide](https://pair-code.github.io/lit/documentation/ui_guide).\n\n## Documentation\n\n* [Documentation index](https://pair-code.github.io/lit/documentation/)\n* [FAQ](https://pair-code.github.io/lit/documentation/faq)\n* [Release notes](./RELEASE.md)\n\n## Download and Installation\n\nLIT can be run via container image, installed via `pip` or built from source.\nBuilding from source is necessary if you update any of the front-end or core\nback-end code.\n\n### Build container image\n\nBuild the image using `docker` or `podman`:\n```sh\ngit clone https://github.com/PAIR-code/lit.git && cd lit\ndocker build --file Dockerfile --tag lit-nlp .\n```\n\nSee the [advanced guide](https://pair-code.github.io/lit/documentation/docker) for detailed instructions on using the\ndefault LIT Docker image, running LIT as a containerized web app in different\nscenarios, and how to creating your own LIT images.\n\n### pip installation\n\n```sh\npip install lit-nlp\n```\n\nThe `pip` installation will install all necessary prerequisite packages for use\nof the core LIT package.\n\nIt **does not** install the prerequisites for the provided demos, so you need to\ninstall those yourself. See\n[requirements_examples.txt](./requirements_examples.txt) for the list of\npackages required to run the demos.\n\n### Install from source\n\nClone the repo:\n\n```sh\ngit clone https://github.com/PAIR-code/lit.git && cd lit\n```\n\n\nNote: be sure you are running Python 3.9+. If you have a different version on\nyour system, use the `conda` instructions below to set up a Python 3.9\nenvironment.\n\nSet up a Python environment with `venv`:\n\n```sh\npython -m venv .venv\nsource .venv/bin/activate\n```\n\nOr set up a Python environment using `conda`:\n\n```sh\nconda create --name lit-nlp\nconda activate lit-nlp\nconda install python=3.9\nconda install pip\n```\n\nOnce you have the environment, install LIT's dependencies:\n```sh\npython -m pip install -r requirements.txt\npython -m pip install cudnn cupti # optional, for GPU support\npython -m pip install torch # optional, for PyTorch\n\n# Build the frontend\n(cd lit_nlp; yarn && yarn build)\n```\n\nNote: Use the `-r requirements.txt` option to install every dependency required\nfor the LIT library, its test suite, and the built-in examples. You can also\ninstall subsets of these using the `-r requirements_core.txt` (core library),\n`-r requirements_test.txt` (test suite), `-r requirements_examples.txt`\n(examples), and/or any combination thereof.\n\nNote: if you see [an error](https://github.com/yarnpkg/yarn/issues/2821)\nrunning `yarn` on Ubuntu/Debian, be sure you have the\n[correct version installed](https://yarnpkg.com/en/docs/install#linux-tab).\n\n\n## Running LIT\n\nExplore a collection of hosted demos on the\n[demos page](https://pair-code.github.io/lit/demos).\n\n### Quick-start: classification and regression\n\nTo explore classification and regression models tasks from the popular\n[GLUE benchmark](https://gluebenchmark.com/):\n\n```sh\npython -m lit_nlp.examples.glue.demo --port=5432 --quickstart\n```\n\nNavigate to http://localhost:5432 to access the LIT UI.\n\nYour default view will be a\n[small BERT-based model](https://arxiv.org/abs/1908.08962) fine-tuned on the\n[Stanford Sentiment Treebank](https://nlp.stanford.edu/sentiment/treebank.html),\nbut you can switch to\n[STS-B](http://ixa2.si.ehu.es/stswiki/index.php/STSbenchmark) or\n[MultiNLI](https://cims.nyu.edu/~sbowman/multinli/) using the toolbar or the\ngear icon in the upper right.\n```\n\nAnd navigate to http://localhost:5432 for the UI.\n\n### Notebook usage\n\nColab notebooks showing the use of LIT inside of notebooks can be found at\n[lit_nlp/examples/notebooks](./lit_nlp/examples/notebooks).\n\nWe provide a simple\n[Colab demo](https://colab.research.google.com/github/PAIR-code/lit/blob/main/lit_nlp/examples/notebooks/LIT_sentiment_classifier.ipynb).\nRun all the cells to see LIT on an example classification model in the notebook.\n\n### More Examples\n\nSee [lit_nlp/examples](./lit_nlp/examples). Most are run similarly to the\nquickstart example above:\n\n```sh\npython -m lit_nlp.examples.<example_name>.demo --port=5432 [optional --args]\n```\n\n## User Guide\n\nTo learn about LIT's features, check out the [user guide](https://pair-code.github.io/lit/documentation/ui_guide), or\nwatch this [video](https://www.youtube.com/watch?v=CuRI_VK83dU).\n\n## Adding your own models or data\n\nYou can easily run LIT with your own model by creating a custom `demo.py`\nlauncher, similar to those in [lit_nlp/examples](./lit_nlp/examples). The\nbasic steps are:\n\n* Write a data loader which follows the [`Dataset` API](https://pair-code.github.io/lit/documentation/api#datasets)\n* Write a model wrapper which follows the [`Model` API](https://pair-code.github.io/lit/documentation/api#models)\n* Pass models, datasets, and any additional\n [components](https://pair-code.github.io/lit/documentation/api#interpretation-components) to the LIT server class\n\nFor a full walkthrough, see\n[adding models and data](https://pair-code.github.io/lit/documentation/api#adding-models-and-data).\n\n## Extending LIT with new components\n\nLIT is easy to extend with new interpretability components, generators, and\nmore, both on the frontend or the backend. See our [documentation](https://pair-code.github.io/lit/documentation/) to get\nstarted.\n\n## Pull Request Process\n\nTo make code changes to LIT, please work off of the `dev` branch and\n[create pull requests](https://docs.github.com/en/pull-requests/collaborating-with-pull-requests/proposing-changes-to-your-work-with-pull-requests/creating-a-pull-request)\n(PRs) against that branch. The `main` branch is for stable releases, and it is\nexpected that the `dev` branch will always be ahead of `main`.\n\n[Draft PRs](https://github.blog/2019-02-14-introducing-draft-pull-requests/) are\nencouraged, especially for first-time contributors or contributors working on\ncomplex tasks (e.g., Google Summer of Code contributors). Please use these to\ncommunicate ideas and implementations with the LIT team, in addition to issues.\n\nPrior to sending your PR or marking a Draft PR as \"Ready for Review\", please run\nthe Python and TypeScript linters on your code to ensure compliance with\nGoogle's [Python](https://google.github.io/styleguide/pyguide.html) and\n[TypeScript](https://google.github.io/styleguide/tsguide.html) Style Guides.\n\n```sh\n# Run Pylint on your code using the following command from the root of this repo\npushd lit_nlp & pylint & popd\n\n# Run ESLint on your code using the following command from the root of this repo\npushd lit_nlp & yarn lint & popd\n```\n\n## Citing LIT\n\nIf you use LIT as part of your work, please cite\n[our EMNLP paper](https://arxiv.org/abs/2008.05122):\n\n```\n@misc{tenney2020language,\n title={The Language Interpretability Tool: Extensible, Interactive Visualizations and Analysis for {NLP} Models},\n author={Ian Tenney and James Wexler and Jasmijn Bastings and Tolga Bolukbasi and Andy Coenen and Sebastian Gehrmann and Ellen Jiang and Mahima Pushkarna and Carey Radebaugh and Emily Reif and Ann Yuan},\n booktitle = \"Proceedings of the 2020 Conference on Empirical Methods in Natural Language Processing: System Demonstrations\",\n year = \"2020\",\n publisher = \"Association for Computational Linguistics\",\n pages = \"107--118\",\n url = \"https://www.aclweb.org/anthology/2020.emnlp-demos.15\",\n}\n```\n\n## Disclaimer\n\nThis is not an official Google product.\n\nLIT is a research project and under active development by a small team. There\nwill be some bugs and rough edges, but we're releasing at an early stage because\nwe think it's pretty useful already. We want LIT to be an open platform, not a\nwalled garden, and we would love your suggestions and feedback - drop us a line\nin the [issues](https://github.com/pair-code/lit/issues).\n",
"bugtrack_url": null,
"license": " Apache License Version 2.0, January 2004 http://www.apache.org/licenses/ TERMS AND CONDITIONS FOR USE, REPRODUCTION, AND DISTRIBUTION 1. Definitions. \"License\" shall mean the terms and conditions for use, reproduction, and distribution as defined by Sections 1 through 9 of this document. \"Licensor\" shall mean the copyright owner or entity authorized by the copyright owner that is granting the License. \"Legal Entity\" shall mean the union of the acting entity and all other entities that control, are controlled by, or are under common control with that entity. For the purposes of this definition, \"control\" means (i) the power, direct or indirect, to cause the direction or management of such entity, whether by contract or otherwise, or (ii) ownership of fifty percent (50%) or more of the outstanding shares, or (iii) beneficial ownership of such entity. \"You\" (or \"Your\") shall mean an individual or Legal Entity exercising permissions granted by this License. \"Source\" form shall mean the preferred form for making modifications, including but not limited to software source code, documentation source, and configuration files. \"Object\" form shall mean any form resulting from mechanical transformation or translation of a Source form, including but not limited to compiled object code, generated documentation, and conversions to other media types. \"Work\" shall mean the work of authorship, whether in Source or Object form, made available under the License, as indicated by a copyright notice that is included in or attached to the work (an example is provided in the Appendix below). \"Derivative Works\" shall mean any work, whether in Source or Object form, that is based on (or derived from) the Work and for which the editorial revisions, annotations, elaborations, or other modifications represent, as a whole, an original work of authorship. For the purposes of this License, Derivative Works shall not include works that remain separable from, or merely link (or bind by name) to the interfaces of, the Work and Derivative Works thereof. \"Contribution\" shall mean any work of authorship, including the original version of the Work and any modifications or additions to that Work or Derivative Works thereof, that is intentionally submitted to Licensor for inclusion in the Work by the copyright owner or by an individual or Legal Entity authorized to submit on behalf of the copyright owner. For the purposes of this definition, \"submitted\" means any form of electronic, verbal, or written communication sent to the Licensor or its representatives, including but not limited to communication on electronic mailing lists, source code control systems, and issue tracking systems that are managed by, or on behalf of, the Licensor for the purpose of discussing and improving the Work, but excluding communication that is conspicuously marked or otherwise designated in writing by the copyright owner as \"Not a Contribution.\" \"Contributor\" shall mean Licensor and any individual or Legal Entity on behalf of whom a Contribution has been received by Licensor and subsequently incorporated within the Work. 2. Grant of Copyright License. Subject to the terms and conditions of this License, each Contributor hereby grants to You a perpetual, worldwide, non-exclusive, no-charge, royalty-free, irrevocable copyright license to reproduce, prepare Derivative Works of, publicly display, publicly perform, sublicense, and distribute the Work and such Derivative Works in Source or Object form. 3. Grant of Patent License. Subject to the terms and conditions of this License, each Contributor hereby grants to You a perpetual, worldwide, non-exclusive, no-charge, royalty-free, irrevocable (except as stated in this section) patent license to make, have made, use, offer to sell, sell, import, and otherwise transfer the Work, where such license applies only to those patent claims licensable by such Contributor that are necessarily infringed by their Contribution(s) alone or by combination of their Contribution(s) with the Work to which such Contribution(s) was submitted. If You institute patent litigation against any entity (including a cross-claim or counterclaim in a lawsuit) alleging that the Work or a Contribution incorporated within the Work constitutes direct or contributory patent infringement, then any patent licenses granted to You under this License for that Work shall terminate as of the date such litigation is filed. 4. Redistribution. You may reproduce and distribute copies of the Work or Derivative Works thereof in any medium, with or without modifications, and in Source or Object form, provided that You meet the following conditions: (a) You must give any other recipients of the Work or Derivative Works a copy of this License; and (b) You must cause any modified files to carry prominent notices stating that You changed the files; and (c) You must retain, in the Source form of any Derivative Works that You distribute, all copyright, patent, trademark, and attribution notices from the Source form of the Work, excluding those notices that do not pertain to any part of the Derivative Works; and (d) If the Work includes a \"NOTICE\" text file as part of its distribution, then any Derivative Works that You distribute must include a readable copy of the attribution notices contained within such NOTICE file, excluding those notices that do not pertain to any part of the Derivative Works, in at least one of the following places: within a NOTICE text file distributed as part of the Derivative Works; within the Source form or documentation, if provided along with the Derivative Works; or, within a display generated by the Derivative Works, if and wherever such third-party notices normally appear. The contents of the NOTICE file are for informational purposes only and do not modify the License. You may add Your own attribution notices within Derivative Works that You distribute, alongside or as an addendum to the NOTICE text from the Work, provided that such additional attribution notices cannot be construed as modifying the License. You may add Your own copyright statement to Your modifications and may provide additional or different license terms and conditions for use, reproduction, or distribution of Your modifications, or for any such Derivative Works as a whole, provided Your use, reproduction, and distribution of the Work otherwise complies with the conditions stated in this License. 5. Submission of Contributions. Unless You explicitly state otherwise, any Contribution intentionally submitted for inclusion in the Work by You to the Licensor shall be under the terms and conditions of this License, without any additional terms or conditions. Notwithstanding the above, nothing herein shall supersede or modify the terms of any separate license agreement you may have executed with Licensor regarding such Contributions. 6. Trademarks. This License does not grant permission to use the trade names, trademarks, service marks, or product names of the Licensor, except as required for reasonable and customary use in describing the origin of the Work and reproducing the content of the NOTICE file. 7. Disclaimer of Warranty. Unless required by applicable law or agreed to in writing, Licensor provides the Work (and each Contributor provides its Contributions) on an \"AS IS\" BASIS, WITHOUT WARRANTIES OR CONDITIONS OF ANY KIND, either express or implied, including, without limitation, any warranties or conditions of TITLE, NON-INFRINGEMENT, MERCHANTABILITY, or FITNESS FOR A PARTICULAR PURPOSE. You are solely responsible for determining the appropriateness of using or redistributing the Work and assume any risks associated with Your exercise of permissions under this License. 8. Limitation of Liability. In no event and under no legal theory, whether in tort (including negligence), contract, or otherwise, unless required by applicable law (such as deliberate and grossly negligent acts) or agreed to in writing, shall any Contributor be liable to You for damages, including any direct, indirect, special, incidental, or consequential damages of any character arising as a result of this License or out of the use or inability to use the Work (including but not limited to damages for loss of goodwill, work stoppage, computer failure or malfunction, or any and all other commercial damages or losses), even if such Contributor has been advised of the possibility of such damages. 9. Accepting Warranty or Additional Liability. While redistributing the Work or Derivative Works thereof, You may choose to offer, and charge a fee for, acceptance of support, warranty, indemnity, or other liability obligations and/or rights consistent with this License. However, in accepting such obligations, You may act only on Your own behalf and on Your sole responsibility, not on behalf of any other Contributor, and only if You agree to indemnify, defend, and hold each Contributor harmless for any liability incurred by, or claims asserted against, such Contributor by reason of your accepting any such warranty or additional liability. END OF TERMS AND CONDITIONS APPENDIX: How to apply the Apache License to your work. To apply the Apache License to your work, attach the following boilerplate notice, with the fields enclosed by brackets \"[]\" replaced with your own identifying information. (Don't include the brackets!) The text should be enclosed in the appropriate comment syntax for the file format. We also recommend that a file or class name and description of purpose be included on the same \"printed page\" as the copyright notice for easier identification within third-party archives. Copyright [yyyy] [name of copyright owner] Licensed under the Apache License, Version 2.0 (the \"License\"); you may not use this file except in compliance with the License. You may obtain a copy of the License at http://www.apache.org/licenses/LICENSE-2.0 Unless required by applicable law or agreed to in writing, software distributed under the License is distributed on an \"AS IS\" BASIS, WITHOUT WARRANTIES OR CONDITIONS OF ANY KIND, either express or implied. See the License for the specific language governing permissions and limitations under the License. ",
"summary": "\ud83d\udd25LIT: The Learning Interpretability Tool",
"version": "1.2",
"project_urls": {
"Bug Tracker": "https://github.com/PAIR-code/lit/issues",
"Homepage": "https://github.com/pair-code/lit",
"Repository": "https://github.com/PAIR-code/lit"
},
"split_keywords": [
"interpretability",
" interpretable artifical intelligence",
" explainable",
" explainable artifical intelligence",
" xai",
" computer vision",
" natural language processing",
" artifical intelligence",
" machine learning",
" deep learning",
" visualization",
" visual analytics",
" tensorflow",
" tf",
" torch"
],
"urls": [
{
"comment_text": "",
"digests": {
"blake2b_256": "43e5e7ee2d8c3d5c987d1b0c6b8967527e194fd08c2a140cf8ca58ddc2094353",
"md5": "c90a8ea5407d38c551a29ca817408a3b",
"sha256": "bd68796c992615765fbc14cd005a2a47a05c8b4bc9e5bcae3df3297321aff8c5"
},
"downloads": -1,
"filename": "lit_nlp-1.2-py3-none-any.whl",
"has_sig": false,
"md5_digest": "c90a8ea5407d38c551a29ca817408a3b",
"packagetype": "bdist_wheel",
"python_version": "py3",
"requires_python": ">=3.9",
"size": 11810457,
"upload_time": "2024-06-26T16:32:34",
"upload_time_iso_8601": "2024-06-26T16:32:34.860272Z",
"url": "https://files.pythonhosted.org/packages/43/e5/e7ee2d8c3d5c987d1b0c6b8967527e194fd08c2a140cf8ca58ddc2094353/lit_nlp-1.2-py3-none-any.whl",
"yanked": false,
"yanked_reason": null
},
{
"comment_text": "",
"digests": {
"blake2b_256": "d11eac01a605a49e54298f9cb0c2060a23504efcf238838d6228360a66aafcd1",
"md5": "ca812330956d03f15dbfff4d916dd3b4",
"sha256": "7a638bcc621a4d840b22f2a5e210fcff5f0c95f0c421afb5a775117effc6c34b"
},
"downloads": -1,
"filename": "lit_nlp-1.2.tar.gz",
"has_sig": false,
"md5_digest": "ca812330956d03f15dbfff4d916dd3b4",
"packagetype": "sdist",
"python_version": "source",
"requires_python": ">=3.9",
"size": 11719801,
"upload_time": "2024-06-26T16:32:41",
"upload_time_iso_8601": "2024-06-26T16:32:41.546626Z",
"url": "https://files.pythonhosted.org/packages/d1/1e/ac01a605a49e54298f9cb0c2060a23504efcf238838d6228360a66aafcd1/lit_nlp-1.2.tar.gz",
"yanked": false,
"yanked_reason": null
}
],
"upload_time": "2024-06-26 16:32:41",
"github": true,
"gitlab": false,
"bitbucket": false,
"codeberg": false,
"github_user": "PAIR-code",
"github_project": "lit",
"travis_ci": false,
"coveralls": false,
"github_actions": true,
"requirements": [],
"lcname": "lit-nlp"
}