<div id="top"></div>
# VIT-VQGAN
This is an unofficial implementation of both [ViT-VQGAN](https://arxiv.org/abs/2110.04627) and [RQ-VAE](https://arxiv.org/abs/2110.04627) in Pytorch. ViT-VQGAN is a simple ViT-based Vector Quantized AutoEncoder while RQ-VAE introduces a new residual quantization scheme. Further details can be viewed in the papers
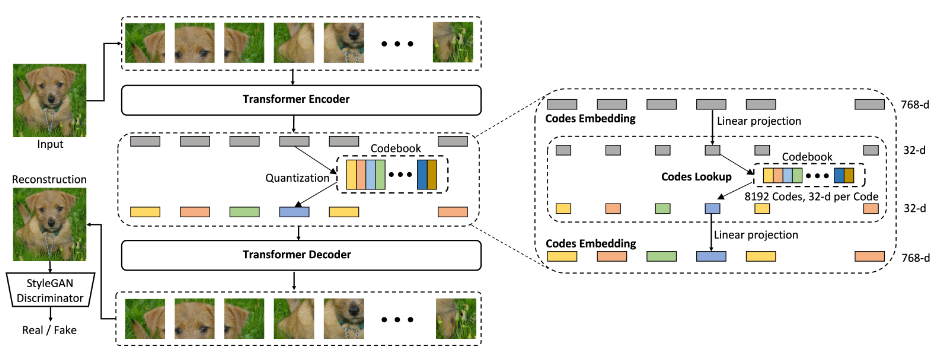
## Installation
```python
pip install vitvqgan
```
## Training
**Train the model:**
```
python -m vitvqgan.train_vim
```
You can add more options too:
```python
python -m vitvqgan.train_vim -c imagenet_vitvq_small -lr 0.00001 -e 10
```
It uses `Imagenette` as the training dataset for demo purpose, to change it, modify [dataloader init file](vitvqgan/dataloader/__init__.py).
**Inference:**
- download checkpoints from above in mbin folder
- Run the following command:
```
python -m vitvqgan.demo_recon
```
## Checkpoints
- [ViT-VQGAN Small](https://drive.google.com/file/d/1jbjD4q0iJpXrRMVSYJRIvM_94AxA1EqJ/view?usp=sharing)
- [ViT-VQGAN Base](https://drive.google.com/file/d/1syv0t3nAJ-bETFgFpztw9cPXghanUaM6/view?usp=sharing)
## Acknowledgements
The repo is modified from [here](https://github.com/thuanz123/enhancing-transformers) with updates to latest dependencies and to be easily run in consumer-grade GPU for learning purpose.
Raw data
{
"_id": null,
"home_page": "https://github.com/henrywoo/vim",
"name": "vitvqgan",
"maintainer": null,
"docs_url": null,
"requires_python": null,
"maintainer_email": null,
"keywords": "vitvqgan",
"author": "Fuheng Wu",
"author_email": null,
"download_url": null,
"platform": null,
"description": "<div id=\"top\"></div>\n\n# VIT-VQGAN\n\nThis is an unofficial implementation of both [ViT-VQGAN](https://arxiv.org/abs/2110.04627) and [RQ-VAE](https://arxiv.org/abs/2110.04627) in Pytorch. ViT-VQGAN is a simple ViT-based Vector Quantized AutoEncoder while RQ-VAE introduces a new residual quantization scheme. Further details can be viewed in the papers\n\n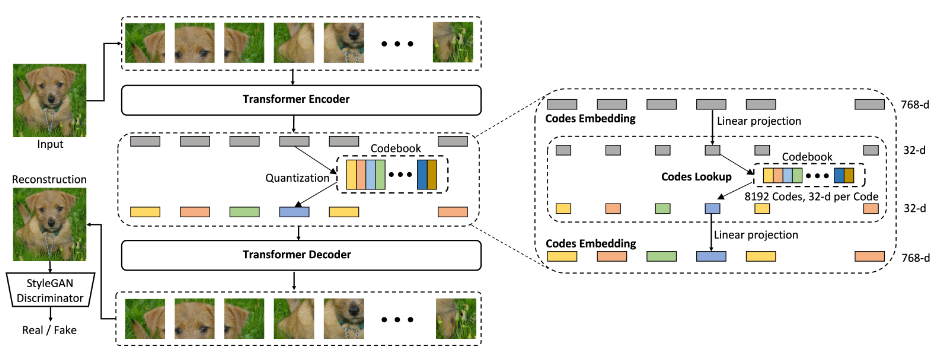\n\n\n## Installation\n\n```python\npip install vitvqgan \n```\n\n\n## Training\n\n**Train the model:**\n```\npython -m vitvqgan.train_vim\n```\n\nYou can add more options too:\n\n```python\npython -m vitvqgan.train_vim -c imagenet_vitvq_small -lr 0.00001 -e 10\n```\n\nIt uses `Imagenette` as the training dataset for demo purpose, to change it, modify [dataloader init file](vitvqgan/dataloader/__init__.py).\n\n**Inference:**\n- download checkpoints from above in mbin folder\n- Run the following command:\n```\npython -m vitvqgan.demo_recon\n```\n\n## Checkpoints\n\n- [ViT-VQGAN Small](https://drive.google.com/file/d/1jbjD4q0iJpXrRMVSYJRIvM_94AxA1EqJ/view?usp=sharing) \n- [ViT-VQGAN Base](https://drive.google.com/file/d/1syv0t3nAJ-bETFgFpztw9cPXghanUaM6/view?usp=sharing)\n\n\n## Acknowledgements\n\nThe repo is modified from [here](https://github.com/thuanz123/enhancing-transformers) with updates to latest dependencies and to be easily run in consumer-grade GPU for learning purpose.\n\n",
"bugtrack_url": null,
"license": null,
"summary": "VITVQGAN - VECTOR-QUANTIZED IMAGE MODELING WITH IMPROVED VQGAN",
"version": "0.0.1.dev1",
"project_urls": {
"Homepage": "https://github.com/henrywoo/vim"
},
"split_keywords": [
"vitvqgan"
],
"urls": [
{
"comment_text": "",
"digests": {
"blake2b_256": "f6564c5b693749692093acc7a391cc9ae8799ecb7e363aac069effc37989a835",
"md5": "3fe5b3f3fd26a72eaa1c1d0a64254931",
"sha256": "86ec8a289cbbc90cefbd6b6c8cc6eab7b8d41831df82f2950ae48dd77341edfc"
},
"downloads": -1,
"filename": "vitvqgan-0.0.1.dev1-py3-none-any.whl",
"has_sig": false,
"md5_digest": "3fe5b3f3fd26a72eaa1c1d0a64254931",
"packagetype": "bdist_wheel",
"python_version": "py3",
"requires_python": null,
"size": 1933350,
"upload_time": "2024-06-29T03:00:51",
"upload_time_iso_8601": "2024-06-29T03:00:51.664152Z",
"url": "https://files.pythonhosted.org/packages/f6/56/4c5b693749692093acc7a391cc9ae8799ecb7e363aac069effc37989a835/vitvqgan-0.0.1.dev1-py3-none-any.whl",
"yanked": false,
"yanked_reason": null
}
],
"upload_time": "2024-06-29 03:00:51",
"github": true,
"gitlab": false,
"bitbucket": false,
"codeberg": false,
"github_user": "henrywoo",
"github_project": "vim",
"travis_ci": false,
"coveralls": false,
"github_actions": false,
"requirements": [],
"lcname": "vitvqgan"
}