Name | inseq JSON |
Version |
0.6.0
JSON |
| download |
home_page | None |
Summary | Interpretability for Sequence Generation Models 🔍 |
upload_time | 2024-04-13 13:37:37 |
maintainer | Gabriele Sarti |
docs_url | None |
author | The Inseq Team |
requires_python | >=3.9 |
license | Apache License Version 2.0, January 2004 http://www.apache.org/licenses/ TERMS AND CONDITIONS FOR USE, REPRODUCTION, AND DISTRIBUTION 1. Definitions. "License" shall mean the terms and conditions for use, reproduction, and distribution as defined by Sections 1 through 9 of this document. "Licensor" shall mean the copyright owner or entity authorized by the copyright owner that is granting the License. "Legal Entity" shall mean the union of the acting entity and all other entities that control, are controlled by, or are under common control with that entity. For the purposes of this definition, "control" means (i) the power, direct or indirect, to cause the direction or management of such entity, whether by contract or otherwise, or (ii) ownership of fifty percent (50%) or more of the outstanding shares, or (iii) beneficial ownership of such entity. "You" (or "Your") shall mean an individual or Legal Entity exercising permissions granted by this License. "Source" form shall mean the preferred form for making modifications, including but not limited to software source code, documentation source, and configuration files. "Object" form shall mean any form resulting from mechanical transformation or translation of a Source form, including but not limited to compiled object code, generated documentation, and conversions to other media types. "Work" shall mean the work of authorship, whether in Source or Object form, made available under the License, as indicated by a copyright notice that is included in or attached to the work (an example is provided in the Appendix below). "Derivative Works" shall mean any work, whether in Source or Object form, that is based on (or derived from) the Work and for which the editorial revisions, annotations, elaborations, or other modifications represent, as a whole, an original work of authorship. For the purposes of this License, Derivative Works shall not include works that remain separable from, or merely link (or bind by name) to the interfaces of, the Work and Derivative Works thereof. "Contribution" shall mean any work of authorship, including the original version of the Work and any modifications or additions to that Work or Derivative Works thereof, that is intentionally submitted to Licensor for inclusion in the Work by the copyright owner or by an individual or Legal Entity authorized to submit on behalf of the copyright owner. For the purposes of this definition, "submitted" means any form of electronic, verbal, or written communication sent to the Licensor or its representatives, including but not limited to communication on electronic mailing lists, source code control systems, and issue tracking systems that are managed by, or on behalf of, the Licensor for the purpose of discussing and improving the Work, but excluding communication that is conspicuously marked or otherwise designated in writing by the copyright owner as "Not a Contribution." "Contributor" shall mean Licensor and any individual or Legal Entity on behalf of whom a Contribution has been received by Licensor and subsequently incorporated within the Work. 2. Grant of Copyright License. Subject to the terms and conditions of this License, each Contributor hereby grants to You a perpetual, worldwide, non-exclusive, no-charge, royalty-free, irrevocable copyright license to reproduce, prepare Derivative Works of, publicly display, publicly perform, sublicense, and distribute the Work and such Derivative Works in Source or Object form. 3. Grant of Patent License. Subject to the terms and conditions of this License, each Contributor hereby grants to You a perpetual, worldwide, non-exclusive, no-charge, royalty-free, irrevocable (except as stated in this section) patent license to make, have made, use, offer to sell, sell, import, and otherwise transfer the Work, where such license applies only to those patent claims licensable by such Contributor that are necessarily infringed by their Contribution(s) alone or by combination of their Contribution(s) with the Work to which such Contribution(s) was submitted. If You institute patent litigation against any entity (including a cross-claim or counterclaim in a lawsuit) alleging that the Work or a Contribution incorporated within the Work constitutes direct or contributory patent infringement, then any patent licenses granted to You under this License for that Work shall terminate as of the date such litigation is filed. 4. Redistribution. You may reproduce and distribute copies of the Work or Derivative Works thereof in any medium, with or without modifications, and in Source or Object form, provided that You meet the following conditions: 1. You must give any other recipients of the Work or Derivative Works a copy of this License; and 2. You must cause any modified files to carry prominent notices stating that You changed the files; and 3. You must retain, in the Source form of any Derivative Works that You distribute, all copyright, patent, trademark, and attribution notices from the Source form of the Work, excluding those notices that do not pertain to any part of the Derivative Works; and 4. If the Work includes a "NOTICE" text file as part of its distribution, then any Derivative Works that You distribute must include a readable copy of the attribution notices contained within such NOTICE file, excluding those notices that do not pertain to any part of the Derivative Works, in at least one of the following places: within a NOTICE text file distributed as part of the Derivative Works; within the Source form or documentation, if provided along with the Derivative Works; or, within a display generated by the Derivative Works, if and wherever such third-party notices normally appear. The contents of the NOTICE file are for informational purposes only and do not modify the License. You may add Your own attribution notices within Derivative Works that You distribute, alongside or as an addendum to the NOTICE text from the Work, provided that such additional attribution notices cannot be construed as modifying the License. You may add Your own copyright statement to Your modifications and may provide additional or different license terms and conditions for use, reproduction, or distribution of Your modifications, or for any such Derivative Works as a whole, provided Your use, reproduction, and distribution of the Work otherwise complies with the conditions stated in this License. 5. Submission of Contributions. Unless You explicitly state otherwise, any Contribution intentionally submitted for inclusion in the Work by You to the Licensor shall be under the terms and conditions of this License, without any additional terms or conditions. Notwithstanding the above, nothing herein shall supersede or modify the terms of any separate license agreement you may have executed with Licensor regarding such Contributions. 6. Trademarks. This License does not grant permission to use the trade names, trademarks, service marks, or product names of the Licensor, except as required for reasonable and customary use in describing the origin of the Work and reproducing the content of the NOTICE file. 7. Disclaimer of Warranty. Unless required by applicable law or agreed to in writing, Licensor provides the Work (and each Contributor provides its Contributions) on an "AS IS" BASIS, WITHOUT WARRANTIES OR CONDITIONS OF ANY KIND, either express or implied, including, without limitation, any warranties or conditions of TITLE, NON-INFRINGEMENT, MERCHANTABILITY, or FITNESS FOR A PARTICULAR PURPOSE. You are solely responsible for determining the appropriateness of using or redistributing the Work and assume any risks associated with Your exercise of permissions under this License. 8. Limitation of Liability. In no event and under no legal theory, whether in tort (including negligence), contract, or otherwise, unless required by applicable law (such as deliberate and grossly negligent acts) or agreed to in writing, shall any Contributor be liable to You for damages, including any direct, indirect, special, incidental, or consequential damages of any character arising as a result of this License or out of the use or inability to use the Work (including but not limited to damages for loss of goodwill, work stoppage, computer failure or malfunction, or any and all other commercial damages or losses), even if such Contributor has been advised of the possibility of such damages. 9. Accepting Warranty or Additional Liability. While redistributing the Work or Derivative Works thereof, You may choose to offer, and charge a fee for, acceptance of support, warranty, indemnity, or other liability obligations and/or rights consistent with this License. However, in accepting such obligations, You may act only on Your own behalf and on Your sole responsibility, not on behalf of any other Contributor, and only if You agree to indemnify, defend, and hold each Contributor harmless for any liability incurred by, or claims asserted against, such Contributor by reason of your accepting any such warranty or additional liability. END OF TERMS AND CONDITIONS APPENDIX: How to apply the Apache License to your work To apply the Apache License to your work, attach the following boilerplate notice, with the fields enclosed by brackets "[]" replaced with your own identifying information. (Don't include the brackets!) The text should be enclosed in the appropriate comment syntax for the file format. We also recommend that a file or class name and description of purpose be included on the same "printed page" as the copyright notice for easier identification within third-party archives. Copyright 2021 The Inseq Team Licensed under the Apache License, Version 2.0 (the "License"); you may not use this file except in compliance with the License. You may obtain a copy of the License at http://www.apache.org/licenses/LICENSE-2.0 Unless required by applicable law or agreed to in writing, software distributed under the License is distributed on an "AS IS" BASIS, WITHOUT WARRANTIES OR CONDITIONS OF ANY KIND, either express or implied. See the License for the specific language governing permissions and limitations under the License. |
keywords |
generative ai
transformers
natural language processing
xai
explainable ai
interpretability
feature attribution
machine translation
|
VCS |
 |
bugtrack_url |
|
requirements |
captum
certifi
charset-normalizer
contourpy
cycler
filelock
fonttools
fsspec
huggingface-hub
idna
jaxtyping
jinja2
kiwisolver
markdown-it-py
markupsafe
matplotlib
mdurl
mpmath
networkx
numpy
packaging
pillow
protobuf
pygments
pyparsing
python-dateutil
pyyaml
regex
requests
rich
safetensors
sentencepiece
six
sympy
tokenizers
torch
tqdm
transformers
typeguard
typing-extensions
urllib3
|
Travis-CI |
No Travis.
|
coveralls test coverage |
No coveralls.
|
<div align="center">
<img src="https://raw.githubusercontent.com/inseq-team/inseq/main/docs/source/images/inseq_logo.png" width="300"/>
<h4>Intepretability for Sequence Generation Models 🔍</h4>
</div>
<br/>
<div align="center">
[](https://github.com/inseq-team/inseq/actions?query=workflow%3Abuild)
[](https://inseq.readthedocs.io)
[](https://pypi.org/project/inseq/)
[](https://pypi.org/project/inseq/)
[](https://pepy.tech/project/inseq)
[](https://github.com/inseq-team/inseq/blob/main/LICENSE)
[](http://arxiv.org/abs/2302.13942)
</div>
<div align="center">
[](https://twitter.com/InseqLib)
[](https://discord.gg/V5VgwwFPbu)
[](https://inseq.org)
[](https://github.com/inseq-team/inseq/blob/main/examples/inseq_tutorial.ipynb)
</div>
Inseq is a Pytorch-based hackable toolkit to democratize the access to common post-hoc **in**terpretability analyses of **seq**uence generation models.
## Installation
Inseq is available on PyPI and can be installed with `pip` for Python >= 3.9, <= 3.11:
```bash
# Install latest stable version
pip install inseq
# Alternatively, install latest development version
pip install git+https://github.com/inseq-team/inseq.git
```
Install extras for visualization in Jupyter Notebooks and 🤗 datasets attribution as `pip install inseq[notebook,datasets]`.
<details>
<summary>Dev Installation</summary>
To install the package, clone the repository and run the following commands:
```bash
cd inseq
make uv-download # Download and install the UV package manager
make install # Installs the package and all dependencies
```
For library developers, you can use the `make install-dev` command to install all development dependencies (quality, docs, extras).
After installation, you should be able to run `make fast-test` and `make lint` without errors.
</details>
<details>
<summary>FAQ Installation</summary>
- Installing the `tokenizers` package requires a Rust compiler installation. You can install Rust from [https://rustup.rs](https://rustup.rs) and add `$HOME/.cargo/env` to your PATH.
- Installing `sentencepiece` requires various packages, install with `sudo apt-get install cmake build-essential pkg-config` or `brew install cmake gperftools pkg-config`.
</details>
## Example usage in Python
This example uses the Integrated Gradients attribution method to attribute the English-French translation of a sentence taken from the WinoMT corpus:
```python
import inseq
model = inseq.load_model("Helsinki-NLP/opus-mt-en-fr", "integrated_gradients")
out = model.attribute(
"The developer argued with the designer because her idea cannot be implemented.",
n_steps=100
)
out.show()
```
This produces a visualization of the attribution scores for each token in the input sentence (token-level aggregation is handled automatically). Here is what the visualization looks like inside a Jupyter Notebook:
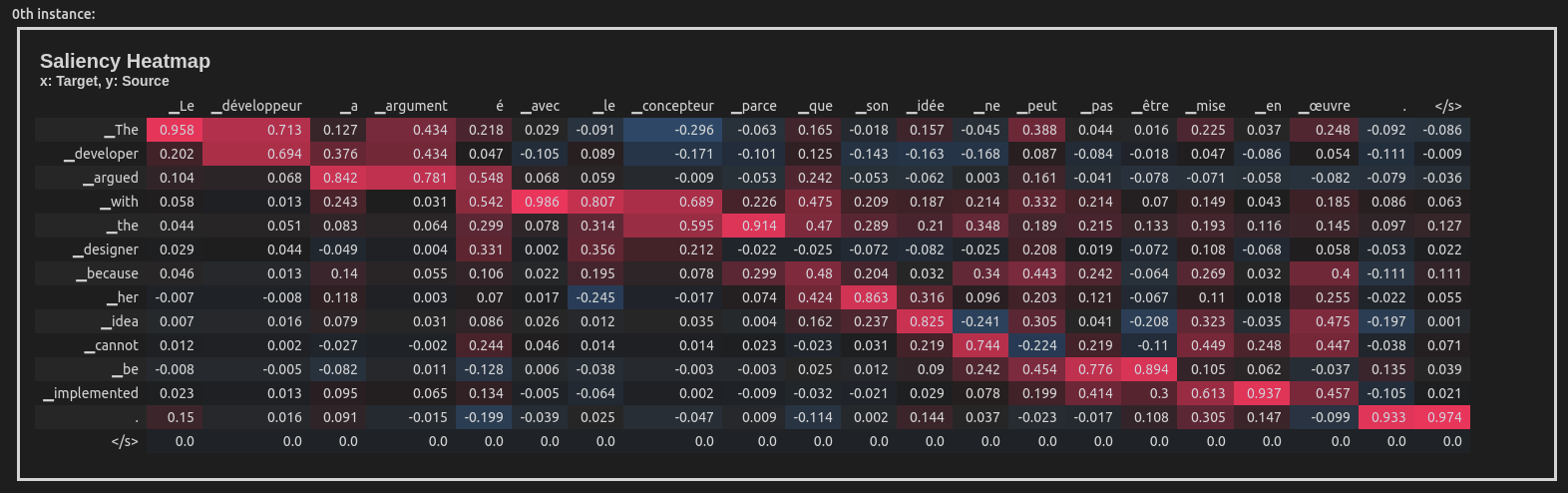
Inseq also supports decoder-only models such as [GPT-2](https://huggingface.co/transformers/model_doc/gpt2.html), enabling usage of a variety of attribution methods and customizable settings directly from the console:
```python
import inseq
model = inseq.load_model("gpt2", "integrated_gradients")
model.attribute(
"Hello ladies and",
generation_args={"max_new_tokens": 9},
n_steps=500,
internal_batch_size=50
).show()
```
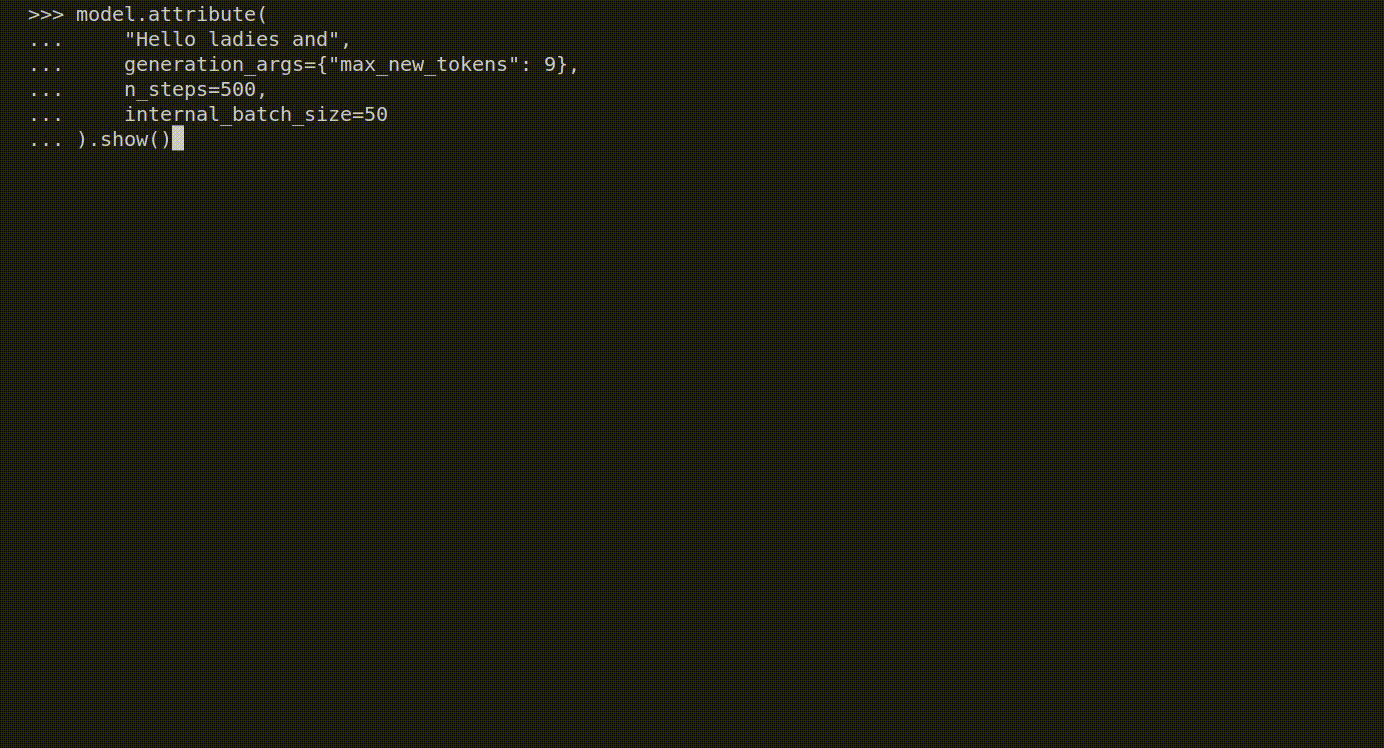
## Features
- 🚀 Feature attribution of sequence generation for most `ForConditionalGeneration` (encoder-decoder) and `ForCausalLM` (decoder-only) models from 🤗 Transformers
- 🚀 Support for multiple feature attribution methods, extending the ones supported by [Captum](https://captum.ai/docs/introduction)
- 🚀 Post-processing, filtering and merging of attribution maps via `Aggregator` classes.
- 🚀 Attribution visualization in notebooks, browser and command line.
- 🚀 Efficient attribution of single examples or entire 🤗 datasets with the Inseq CLI.
- 🚀 Custom attribution of target functions, supporting advanced methods such as [contrastive feature attributions](https://aclanthology.org/2022.emnlp-main.14/) and [context reliance detection](https://arxiv.org/abs/2310.01188).
- 🚀 Extraction and visualization of custom scores (e.g. probability, entropy) at every generation step alongsides attribution maps.
### Supported methods
Use the `inseq.list_feature_attribution_methods` function to list all available method identifiers and `inseq.list_step_functions` to list all available step functions. The following methods are currently supported:
#### Gradient-based attribution
- `saliency`: [Deep Inside Convolutional Networks: Visualising Image Classification Models and Saliency Maps](https://arxiv.org/abs/1312.6034) (Simonyan et al., 2013)
- `input_x_gradient`: [Deep Inside Convolutional Networks: Visualising Image Classification Models and Saliency Maps](https://arxiv.org/abs/1312.6034) (Simonyan et al., 2013)
- `integrated_gradients`: [Axiomatic Attribution for Deep Networks](https://arxiv.org/abs/1703.01365) (Sundararajan et al., 2017)
- `deeplift`: [Learning Important Features Through Propagating Activation Differences](https://arxiv.org/abs/1704.02685) (Shrikumar et al., 2017)
- `gradient_shap`: [A unified approach to interpreting model predictions](https://dl.acm.org/doi/10.5555/3295222.3295230) (Lundberg and Lee, 2017)
- `discretized_integrated_gradients`: [Discretized Integrated Gradients for Explaining Language Models](https://aclanthology.org/2021.emnlp-main.805/) (Sanyal and Ren, 2021)
- `sequential_integrated_gradients`: [Sequential Integrated Gradients: a simple but effective method for explaining language models](https://aclanthology.org/2023.findings-acl.477/) (Enguehard, 2023)
#### Internals-based attribution
- `attention`: Attention Weight Attribution, from [Neural Machine Translation by Jointly Learning to Align and Translate](https://arxiv.org/abs/1409.0473) (Bahdanau et al., 2014)
#### Perturbation-based attribution
- `occlusion`: [Visualizing and Understanding Convolutional Networks](https://link.springer.com/chapter/10.1007/978-3-319-10590-1_53) (Zeiler and Fergus, 2014)
- `lime`: ["Why Should I Trust You?": Explaining the Predictions of Any Classifier](https://arxiv.org/abs/1602.04938) (Ribeiro et al., 2016)
- `value_zeroing`: [Quantifying Context Mixing in Transformers](https://aclanthology.org/2023.eacl-main.245/) (Mohebbi et al. 2023)
- `reagent`: [ReAGent: A Model-agnostic Feature Attribution Method for Generative Language Models](https://arxiv.org/abs/2402.00794) (Zhao et al., 2024)
#### Step functions
Step functions are used to extract custom scores from the model at each step of the attribution process with the `step_scores` argument in `model.attribute`. They can also be used as targets for attribution methods relying on model outputs (e.g. gradient-based methods) by passing them as the `attributed_fn` argument. The following step functions are currently supported:
- `logits`: Logits of the target token.
- `probability`: Probability of the target token. Can also be used for log-probability by passing `logprob=True`.
- `entropy`: Entropy of the predictive distribution.
- `crossentropy`: Cross-entropy loss between target token and predicted distribution.
- `perplexity`: Perplexity of the target token.
- `contrast_logits`/`contrast_prob`: Logits/probabilities of the target token when different contrastive inputs are provided to the model. Equivalent to `logits`/`probability` when no contrastive inputs are provided.
- `contrast_logits_diff`/`contrast_prob_diff`: Difference in logits/probability between original and foil target tokens pair, can be used for contrastive evaluation as in [contrastive attribution](https://aclanthology.org/2022.emnlp-main.14/) (Yin and Neubig, 2022).
- `pcxmi`: Point-wise Contextual Cross-Mutual Information (P-CXMI) for the target token given original and contrastive contexts [(Yin et al. 2021)](https://arxiv.org/abs/2109.07446).
- `kl_divergence`: KL divergence of the predictive distribution given original and contrastive contexts. Can be restricted to most likely target token options using the `top_k` and `top_p` parameters.
- `in_context_pvi`: In-context Pointwise V-usable Information (PVI) to measure the amount of contextual information used in model predictions [(Lu et al. 2023)](https://arxiv.org/abs/2310.12300).
- `mc_dropout_prob_avg`: Average probability of the target token across multiple samples using [MC Dropout](https://arxiv.org/abs/1506.02142) (Gal and Ghahramani, 2016).
- `top_p_size`: The number of tokens with cumulative probability greater than `top_p` in the predictive distribution of the model.
The following example computes contrastive attributions using the `contrast_prob_diff` step function:
```python
import inseq
attribution_model = inseq.load_model("gpt2", "input_x_gradient")
# Perform the contrastive attribution:
# Regular (forced) target -> "The manager went home because he was sick"
# Contrastive target -> "The manager went home because she was sick"
out = attribution_model.attribute(
"The manager went home because",
"The manager went home because he was sick",
attributed_fn="contrast_prob_diff",
contrast_targets="The manager went home because she was sick",
# We also visualize the corresponding step score
step_scores=["contrast_prob_diff"]
)
out.show()
```
Refer to the [documentation](https://inseq.readthedocs.io/examples/custom_attribute_target.html) for an example including custom function registration.
## Using the Inseq CLI
The Inseq library also provides useful client commands to enable repeated attribution of individual examples and even entire 🤗 datasets directly from the console. See the available options by typing `inseq -h` in the terminal after installing the package.
Three commands are supported:
- `inseq attribute`: Wrapper for enabling `model.attribute` usage in console.
- `inseq attribute-dataset`: Extends `attribute` to full dataset using Hugging Face `datasets.load_dataset` API.
- `inseq attribute-context`: Detects and attribute context dependence for generation tasks using the approach of [Sarti et al. (2023)](https://arxiv.org/abs/2310.01188).
All commands support the full range of parameters available for `attribute`, attribution visualization in the console and saving outputs to disk.
<details>
<summary><code>inseq attribute</code> example</summary>
The following example performs a simple feature attribution of an English sentence translated into Italian using a MarianNMT translation model from <code>transformers</code>. The final result is printed to the console.
```bash
inseq attribute \
--model_name_or_path Helsinki-NLP/opus-mt-en-it \
--attribution_method saliency \
--input_texts "Hello world this is Inseq\! Inseq is a very nice library to perform attribution analysis"
```
</details>
<details>
<summary><code>inseq attribute-dataset</code> example</summary>
The following code can be used to perform attribution (both source and target-side) of Italian translations for a dummy sample of 20 English sentences taken from the FLORES-101 parallel corpus, using a MarianNMT translation model from Hugging Face <code>transformers</code>. We save the visualizations in HTML format in the file <code>attributions.html</code>. See the <code>--help</code> flag for more options.
```bash
inseq attribute-dataset \
--model_name_or_path Helsinki-NLP/opus-mt-en-it \
--attribution_method saliency \
--do_prefix_attribution \
--dataset_name inseq/dummy_enit \
--input_text_field en \
--dataset_split "train[:20]" \
--viz_path attributions.html \
--batch_size 8 \
--hide
```
</details>
<details>
<summary><code>inseq attribute-context</code> example</summary>
The following example uses a GPT-2 model to generate a continuation of <code>input_current_text</code>, and uses the additional context provided by <code>input_context_text</code> to estimate its influence on the the generation. In this case, the output <code>"to the hospital. He said he was fine"</code> is produced, and the generation of token <code>hospital</code> is found to be dependent on context token <code>sick</code> according to the <code>contrast_prob_diff</code> step function.
```bash
inseq attribute-context \
--model_name_or_path gpt2 \
--input_context_text "George was sick yesterday." \
--input_current_text "His colleagues asked him to come" \
--attributed_fn "contrast_prob_diff"
```
**Result:**
```
Context with [contextual cues] (std λ=1.00) followed by output sentence with {context-sensitive target spans} (std λ=1.00)
(CTI = "kl_divergence", CCI = "saliency" w/ "contrast_prob_diff" target)
Input context: George was sick yesterday.
Input current: His colleagues asked him to come
Output current: to the hospital. He said he was fine
#1.
Generated output (CTI > 0.428): to the {hospital}(0.548). He said he was fine
Input context (CCI > 0.460): George was [sick](0.516) yesterday.
```
</details>
## Planned Development
- ⚙️ Support more attention-based and occlusion-based feature attribution methods (documented in [#107](https://github.com/inseq-team/inseq/issues/107) and [#108](https://github.com/inseq-team/inseq/issues/108)).
- ⚙️ Interoperability with [ferret](https://ferret.readthedocs.io/en/latest/) for attribution plausibility and faithfulness evaluation.
- ⚙️ Rich and interactive visualizations in a tabbed interface using [Gradio Blocks](https://gradio.app/docs/#blocks).
## Contributing
Our vision for Inseq is to create a centralized, comprehensive and robust set of tools to enable fair and reproducible comparisons in the study of sequence generation models. To achieve this goal, contributions from researchers and developers interested in these topics are more than welcome. Please see our [contributing guidelines](CONTRIBUTING.md) and our [code of conduct](CODE_OF_CONDUCT.md) for more information.
## Citing Inseq
If you use Inseq in your research we suggest to include a mention to the specific release (e.g. v0.4.0) and we kindly ask you to cite our reference paper as:
```bibtex
@inproceedings{sarti-etal-2023-inseq,
title = "Inseq: An Interpretability Toolkit for Sequence Generation Models",
author = "Sarti, Gabriele and
Feldhus, Nils and
Sickert, Ludwig and
van der Wal, Oskar and
Nissim, Malvina and
Bisazza, Arianna",
booktitle = "Proceedings of the 61st Annual Meeting of the Association for Computational Linguistics (Volume 3: System Demonstrations)",
month = jul,
year = "2023",
address = "Toronto, Canada",
publisher = "Association for Computational Linguistics",
url = "https://aclanthology.org/2023.acl-demo.40",
doi = "10.18653/v1/2023.acl-demo.40",
pages = "421--435",
}
```
## Research using Inseq
Inseq has been used in various research projects. A list of known publications that use Inseq to conduct interpretability analyses of generative models is shown below.
> [!TIP]
> Last update: April 2024. Please open a pull request to add your publication to the list.
<details>
<summary><b>2023</b></summary>
<ol>
<li> <a href="https://aclanthology.org/2023.acl-demo.40/">Inseq: An Interpretability Toolkit for Sequence Generation Models</a> (Sarti et al., 2023) </li>
<li> <a href="https://arxiv.org/abs/2302.14220">Are Character-level Translations Worth the Wait? Comparing ByT5 and mT5 for Machine Translation</a> (Edman et al., 2023) </li>
<li> <a href="https://aclanthology.org/2023.nlp4convai-1.1/">Response Generation in Longitudinal Dialogues: Which Knowledge Representation Helps?</a> (Mousavi et al., 2023) </li>
<li> <a href="https://openreview.net/forum?id=XTHfNGI3zT">Quantifying the Plausibility of Context Reliance in Neural Machine Translation</a> (Sarti et al., 2023)</li>
<li> <a href="https://aclanthology.org/2023.emnlp-main.243/">A Tale of Pronouns: Interpretability Informs Gender Bias Mitigation for Fairer Instruction-Tuned Machine Translation</a> (Attanasio et al., 2023)</li>
<li> <a href="https://arxiv.org/abs/2310.09820">Assessing the Reliability of Large Language Model Knowledge</a> (Wang et al., 2023)</li>
<li> <a href="https://aclanthology.org/2023.conll-1.18/">Attribution and Alignment: Effects of Local Context Repetition on Utterance Production and Comprehension in Dialogue</a> (Molnar et al., 2023)</li>
</ol>
</details>
<details>
<summary><b>2024</b></summary>
<ol>
<li><a href="https://arxiv.org/abs/2401.12576">LLMCheckup: Conversational Examination of Large Language Models via Interpretability Tools</a> (Wang et al., 2024)</li>
<li><a href="https://arxiv.org/abs/2402.00794">ReAGent: A Model-agnostic Feature Attribution Method for Generative Language Models</a> (Zhao et al., 2024)</li>
<li><a href="https://arxiv.org/abs/2404.02421">Revisiting subword tokenization: A case study on affixal negation in large language models</a> (Truong et al., 2024)</li>
</ol>
</details>
Raw data
{
"_id": null,
"home_page": null,
"name": "inseq",
"maintainer": "Gabriele Sarti",
"docs_url": null,
"requires_python": ">=3.9",
"maintainer_email": "gabriele.sarti996@gmail.com",
"keywords": "generative AI, transformers, natural language processing, XAI, explainable ai, interpretability, feature attribution, machine translation",
"author": "The Inseq Team",
"author_email": "info@inseq.org",
"download_url": "https://files.pythonhosted.org/packages/bf/83/499413b48bcf0fab5213d253b1203702c8a8fa64f5ba24f10cc186942ad3/inseq-0.6.0.tar.gz",
"platform": null,
"description": "<div align=\"center\">\n <img src=\"https://raw.githubusercontent.com/inseq-team/inseq/main/docs/source/images/inseq_logo.png\" width=\"300\"/>\n <h4>Intepretability for Sequence Generation Models \ud83d\udd0d</h4>\n</div>\n<br/>\n<div align=\"center\">\n\n\n[](https://github.com/inseq-team/inseq/actions?query=workflow%3Abuild)\n[](https://inseq.readthedocs.io)\n[](https://pypi.org/project/inseq/)\n[](https://pypi.org/project/inseq/)\n[](https://pepy.tech/project/inseq)\n[](https://github.com/inseq-team/inseq/blob/main/LICENSE)\n[](http://arxiv.org/abs/2302.13942)\n\n</div>\n<div align=\"center\">\n\n [](https://twitter.com/InseqLib)\n [](https://discord.gg/V5VgwwFPbu)\n [](https://inseq.org)\n [](https://github.com/inseq-team/inseq/blob/main/examples/inseq_tutorial.ipynb)\n\n\n</div>\n\nInseq is a Pytorch-based hackable toolkit to democratize the access to common post-hoc **in**terpretability analyses of **seq**uence generation models.\n\n## Installation\n\nInseq is available on PyPI and can be installed with `pip` for Python >= 3.9, <= 3.11:\n\n```bash\n# Install latest stable version\npip install inseq\n\n# Alternatively, install latest development version\npip install git+https://github.com/inseq-team/inseq.git\n```\n\nInstall extras for visualization in Jupyter Notebooks and \ud83e\udd17 datasets attribution as `pip install inseq[notebook,datasets]`.\n\n<details>\n <summary>Dev Installation</summary>\nTo install the package, clone the repository and run the following commands:\n\n```bash\ncd inseq\nmake uv-download # Download and install the UV package manager\nmake install # Installs the package and all dependencies\n```\n\nFor library developers, you can use the `make install-dev` command to install all development dependencies (quality, docs, extras).\n\nAfter installation, you should be able to run `make fast-test` and `make lint` without errors.\n</details>\n\n<details>\n <summary>FAQ Installation</summary>\n\n- Installing the `tokenizers` package requires a Rust compiler installation. You can install Rust from [https://rustup.rs](https://rustup.rs) and add `$HOME/.cargo/env` to your PATH.\n\n- Installing `sentencepiece` requires various packages, install with `sudo apt-get install cmake build-essential pkg-config` or `brew install cmake gperftools pkg-config`.\n\n</details>\n\n## Example usage in Python\n\nThis example uses the Integrated Gradients attribution method to attribute the English-French translation of a sentence taken from the WinoMT corpus:\n\n```python\nimport inseq\n\nmodel = inseq.load_model(\"Helsinki-NLP/opus-mt-en-fr\", \"integrated_gradients\")\nout = model.attribute(\n \"The developer argued with the designer because her idea cannot be implemented.\",\n n_steps=100\n)\nout.show()\n```\n\nThis produces a visualization of the attribution scores for each token in the input sentence (token-level aggregation is handled automatically). Here is what the visualization looks like inside a Jupyter Notebook:\n\n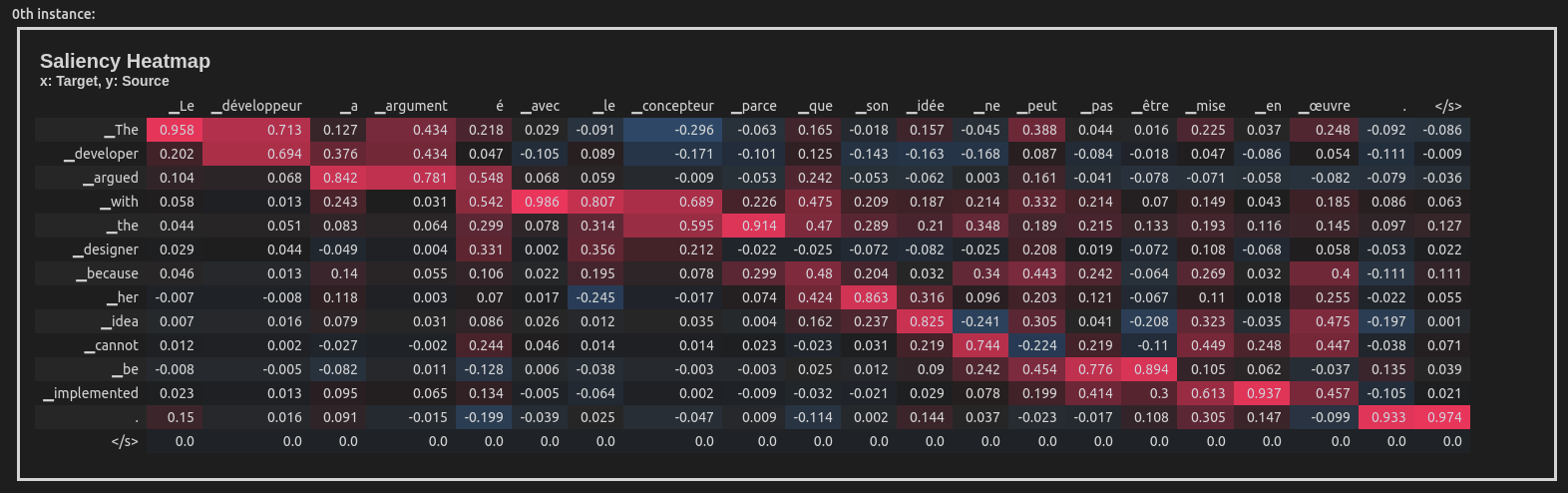\n\nInseq also supports decoder-only models such as [GPT-2](https://huggingface.co/transformers/model_doc/gpt2.html), enabling usage of a variety of attribution methods and customizable settings directly from the console:\n\n```python\nimport inseq\n\nmodel = inseq.load_model(\"gpt2\", \"integrated_gradients\")\nmodel.attribute(\n \"Hello ladies and\",\n generation_args={\"max_new_tokens\": 9},\n n_steps=500,\n internal_batch_size=50\n).show()\n```\n\n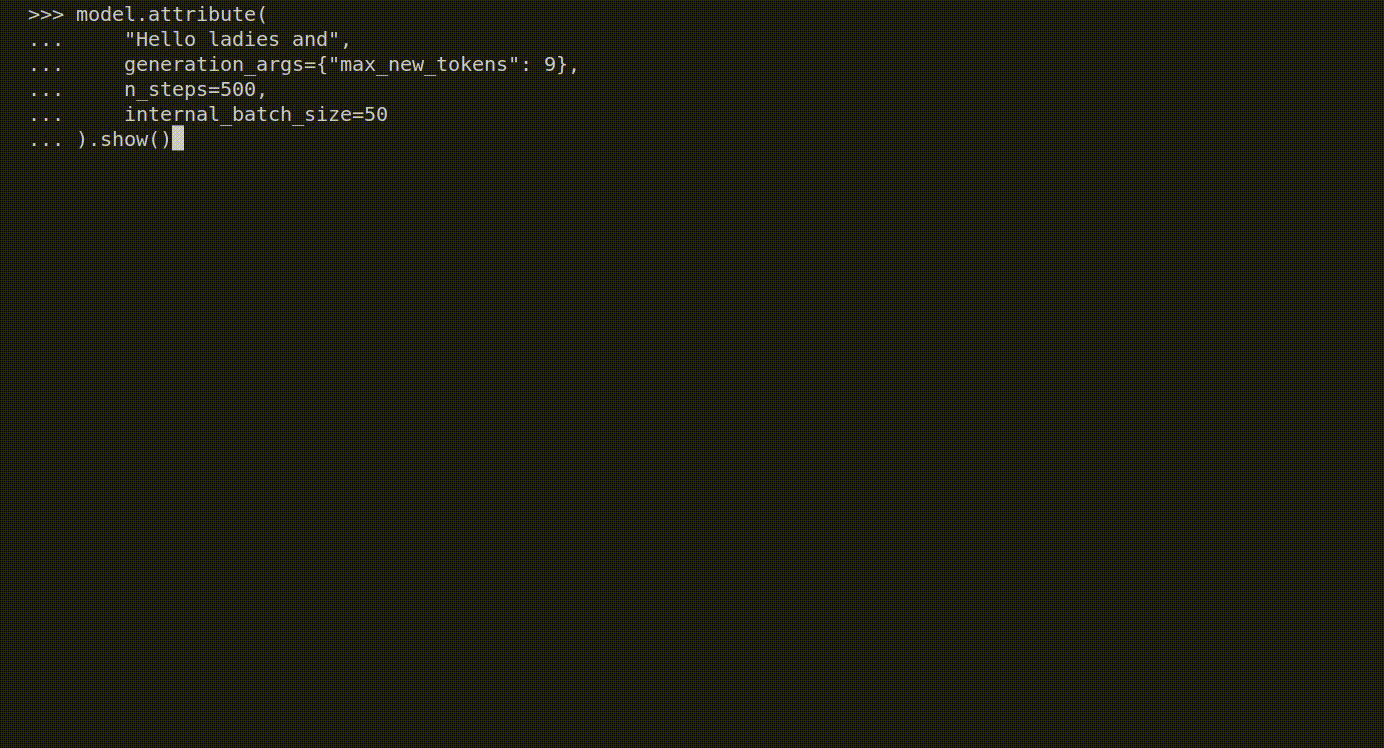\n\n## Features\n\n- \ud83d\ude80 Feature attribution of sequence generation for most `ForConditionalGeneration` (encoder-decoder) and `ForCausalLM` (decoder-only) models from \ud83e\udd17 Transformers\n\n- \ud83d\ude80 Support for multiple feature attribution methods, extending the ones supported by [Captum](https://captum.ai/docs/introduction)\n\n- \ud83d\ude80 Post-processing, filtering and merging of attribution maps via `Aggregator` classes.\n\n- \ud83d\ude80 Attribution visualization in notebooks, browser and command line.\n\n- \ud83d\ude80 Efficient attribution of single examples or entire \ud83e\udd17 datasets with the Inseq CLI.\n\n- \ud83d\ude80 Custom attribution of target functions, supporting advanced methods such as [contrastive feature attributions](https://aclanthology.org/2022.emnlp-main.14/) and [context reliance detection](https://arxiv.org/abs/2310.01188).\n\n- \ud83d\ude80 Extraction and visualization of custom scores (e.g. probability, entropy) at every generation step alongsides attribution maps.\n\n### Supported methods\n\nUse the `inseq.list_feature_attribution_methods` function to list all available method identifiers and `inseq.list_step_functions` to list all available step functions. The following methods are currently supported:\n\n#### Gradient-based attribution\n\n- `saliency`: [Deep Inside Convolutional Networks: Visualising Image Classification Models and Saliency Maps](https://arxiv.org/abs/1312.6034) (Simonyan et al., 2013)\n\n- `input_x_gradient`: [Deep Inside Convolutional Networks: Visualising Image Classification Models and Saliency Maps](https://arxiv.org/abs/1312.6034) (Simonyan et al., 2013)\n\n- `integrated_gradients`: [Axiomatic Attribution for Deep Networks](https://arxiv.org/abs/1703.01365) (Sundararajan et al., 2017)\n\n- `deeplift`: [Learning Important Features Through Propagating Activation Differences](https://arxiv.org/abs/1704.02685) (Shrikumar et al., 2017)\n\n- `gradient_shap`: [A unified approach to interpreting model predictions](https://dl.acm.org/doi/10.5555/3295222.3295230) (Lundberg and Lee, 2017)\n\n- `discretized_integrated_gradients`: [Discretized Integrated Gradients for Explaining Language Models](https://aclanthology.org/2021.emnlp-main.805/) (Sanyal and Ren, 2021)\n\n- `sequential_integrated_gradients`: [Sequential Integrated Gradients: a simple but effective method for explaining language models](https://aclanthology.org/2023.findings-acl.477/) (Enguehard, 2023)\n\n#### Internals-based attribution\n\n- `attention`: Attention Weight Attribution, from [Neural Machine Translation by Jointly Learning to Align and Translate](https://arxiv.org/abs/1409.0473) (Bahdanau et al., 2014)\n\n#### Perturbation-based attribution\n\n- `occlusion`: [Visualizing and Understanding Convolutional Networks](https://link.springer.com/chapter/10.1007/978-3-319-10590-1_53) (Zeiler and Fergus, 2014)\n\n- `lime`: [\"Why Should I Trust You?\": Explaining the Predictions of Any Classifier](https://arxiv.org/abs/1602.04938) (Ribeiro et al., 2016)\n\n- `value_zeroing`: [Quantifying Context Mixing in Transformers](https://aclanthology.org/2023.eacl-main.245/) (Mohebbi et al. 2023)\n\n- `reagent`: [ReAGent: A Model-agnostic Feature Attribution Method for Generative Language Models](https://arxiv.org/abs/2402.00794) (Zhao et al., 2024)\n\n#### Step functions\n\nStep functions are used to extract custom scores from the model at each step of the attribution process with the `step_scores` argument in `model.attribute`. They can also be used as targets for attribution methods relying on model outputs (e.g. gradient-based methods) by passing them as the `attributed_fn` argument. The following step functions are currently supported:\n\n- `logits`: Logits of the target token.\n- `probability`: Probability of the target token. Can also be used for log-probability by passing `logprob=True`.\n- `entropy`: Entropy of the predictive distribution.\n- `crossentropy`: Cross-entropy loss between target token and predicted distribution.\n- `perplexity`: Perplexity of the target token.\n- `contrast_logits`/`contrast_prob`: Logits/probabilities of the target token when different contrastive inputs are provided to the model. Equivalent to `logits`/`probability` when no contrastive inputs are provided.\n- `contrast_logits_diff`/`contrast_prob_diff`: Difference in logits/probability between original and foil target tokens pair, can be used for contrastive evaluation as in [contrastive attribution](https://aclanthology.org/2022.emnlp-main.14/) (Yin and Neubig, 2022).\n- `pcxmi`: Point-wise Contextual Cross-Mutual Information (P-CXMI) for the target token given original and contrastive contexts [(Yin et al. 2021)](https://arxiv.org/abs/2109.07446).\n- `kl_divergence`: KL divergence of the predictive distribution given original and contrastive contexts. Can be restricted to most likely target token options using the `top_k` and `top_p` parameters.\n- `in_context_pvi`: In-context Pointwise V-usable Information (PVI) to measure the amount of contextual information used in model predictions [(Lu et al. 2023)](https://arxiv.org/abs/2310.12300).\n- `mc_dropout_prob_avg`: Average probability of the target token across multiple samples using [MC Dropout](https://arxiv.org/abs/1506.02142) (Gal and Ghahramani, 2016).\n- `top_p_size`: The number of tokens with cumulative probability greater than `top_p` in the predictive distribution of the model.\n\nThe following example computes contrastive attributions using the `contrast_prob_diff` step function:\n\n```python\nimport inseq\n\nattribution_model = inseq.load_model(\"gpt2\", \"input_x_gradient\")\n\n# Perform the contrastive attribution:\n# Regular (forced) target -> \"The manager went home because he was sick\"\n# Contrastive target -> \"The manager went home because she was sick\"\nout = attribution_model.attribute(\n \"The manager went home because\",\n \"The manager went home because he was sick\",\n attributed_fn=\"contrast_prob_diff\",\n contrast_targets=\"The manager went home because she was sick\",\n # We also visualize the corresponding step score\n step_scores=[\"contrast_prob_diff\"]\n)\nout.show()\n```\n\nRefer to the [documentation](https://inseq.readthedocs.io/examples/custom_attribute_target.html) for an example including custom function registration.\n\n## Using the Inseq CLI\n\nThe Inseq library also provides useful client commands to enable repeated attribution of individual examples and even entire \ud83e\udd17 datasets directly from the console. See the available options by typing `inseq -h` in the terminal after installing the package.\n\nThree commands are supported:\n\n- `inseq attribute`: Wrapper for enabling `model.attribute` usage in console.\n\n- `inseq attribute-dataset`: Extends `attribute` to full dataset using Hugging Face `datasets.load_dataset` API.\n\n- `inseq attribute-context`: Detects and attribute context dependence for generation tasks using the approach of [Sarti et al. (2023)](https://arxiv.org/abs/2310.01188).\n\nAll commands support the full range of parameters available for `attribute`, attribution visualization in the console and saving outputs to disk.\n\n<details>\n <summary><code>inseq attribute</code> example</summary>\n\n The following example performs a simple feature attribution of an English sentence translated into Italian using a MarianNMT translation model from <code>transformers</code>. The final result is printed to the console.\n ```bash\n inseq attribute \\\n --model_name_or_path Helsinki-NLP/opus-mt-en-it \\\n --attribution_method saliency \\\n --input_texts \"Hello world this is Inseq\\! Inseq is a very nice library to perform attribution analysis\"\n ```\n\n</details>\n\n<details>\n <summary><code>inseq attribute-dataset</code> example</summary>\n\n The following code can be used to perform attribution (both source and target-side) of Italian translations for a dummy sample of 20 English sentences taken from the FLORES-101 parallel corpus, using a MarianNMT translation model from Hugging Face <code>transformers</code>. We save the visualizations in HTML format in the file <code>attributions.html</code>. See the <code>--help</code> flag for more options.\n\n ```bash\n inseq attribute-dataset \\\n --model_name_or_path Helsinki-NLP/opus-mt-en-it \\\n --attribution_method saliency \\\n --do_prefix_attribution \\\n --dataset_name inseq/dummy_enit \\\n --input_text_field en \\\n --dataset_split \"train[:20]\" \\\n --viz_path attributions.html \\\n --batch_size 8 \\\n --hide\n ```\n</details>\n\n<details>\n <summary><code>inseq attribute-context</code> example</summary>\n\n The following example uses a GPT-2 model to generate a continuation of <code>input_current_text</code>, and uses the additional context provided by <code>input_context_text</code> to estimate its influence on the the generation. In this case, the output <code>\"to the hospital. He said he was fine\"</code> is produced, and the generation of token <code>hospital</code> is found to be dependent on context token <code>sick</code> according to the <code>contrast_prob_diff</code> step function.\n\n ```bash\n inseq attribute-context \\\n --model_name_or_path gpt2 \\\n --input_context_text \"George was sick yesterday.\" \\\n --input_current_text \"His colleagues asked him to come\" \\\n --attributed_fn \"contrast_prob_diff\"\n ```\n\n **Result:**\n\n ```\n Context with [contextual cues] (std \u03bb=1.00) followed by output sentence with {context-sensitive target spans} (std \u03bb=1.00)\n (CTI = \"kl_divergence\", CCI = \"saliency\" w/ \"contrast_prob_diff\" target)\n\n Input context: George was sick yesterday.\n Input current: His colleagues asked him to come\n Output current: to the hospital. He said he was fine\n\n #1.\n Generated output (CTI > 0.428): to the {hospital}(0.548). He said he was fine\n Input context (CCI > 0.460): George was [sick](0.516) yesterday.\n ```\n</details>\n\n## Planned Development\n\n- \u2699\ufe0f Support more attention-based and occlusion-based feature attribution methods (documented in [#107](https://github.com/inseq-team/inseq/issues/107) and [#108](https://github.com/inseq-team/inseq/issues/108)).\n\n- \u2699\ufe0f Interoperability with [ferret](https://ferret.readthedocs.io/en/latest/) for attribution plausibility and faithfulness evaluation.\n\n- \u2699\ufe0f Rich and interactive visualizations in a tabbed interface using [Gradio Blocks](https://gradio.app/docs/#blocks).\n\n## Contributing\n\nOur vision for Inseq is to create a centralized, comprehensive and robust set of tools to enable fair and reproducible comparisons in the study of sequence generation models. To achieve this goal, contributions from researchers and developers interested in these topics are more than welcome. Please see our [contributing guidelines](CONTRIBUTING.md) and our [code of conduct](CODE_OF_CONDUCT.md) for more information.\n\n## Citing Inseq\n\nIf you use Inseq in your research we suggest to include a mention to the specific release (e.g. v0.4.0) and we kindly ask you to cite our reference paper as:\n\n```bibtex\n@inproceedings{sarti-etal-2023-inseq,\n title = \"Inseq: An Interpretability Toolkit for Sequence Generation Models\",\n author = \"Sarti, Gabriele and\n Feldhus, Nils and\n Sickert, Ludwig and\n van der Wal, Oskar and\n Nissim, Malvina and\n Bisazza, Arianna\",\n booktitle = \"Proceedings of the 61st Annual Meeting of the Association for Computational Linguistics (Volume 3: System Demonstrations)\",\n month = jul,\n year = \"2023\",\n address = \"Toronto, Canada\",\n publisher = \"Association for Computational Linguistics\",\n url = \"https://aclanthology.org/2023.acl-demo.40\",\n doi = \"10.18653/v1/2023.acl-demo.40\",\n pages = \"421--435\",\n}\n\n```\n\n## Research using Inseq\n\nInseq has been used in various research projects. A list of known publications that use Inseq to conduct interpretability analyses of generative models is shown below.\n\n> [!TIP]\n> Last update: April 2024. Please open a pull request to add your publication to the list.\n\n<details>\n <summary><b>2023</b></summary>\n <ol>\n <li> <a href=\"https://aclanthology.org/2023.acl-demo.40/\">Inseq: An Interpretability Toolkit for Sequence Generation Models</a> (Sarti et al., 2023) </li>\n <li> <a href=\"https://arxiv.org/abs/2302.14220\">Are Character-level Translations Worth the Wait? Comparing ByT5 and mT5 for Machine Translation</a> (Edman et al., 2023) </li>\n <li> <a href=\"https://aclanthology.org/2023.nlp4convai-1.1/\">Response Generation in Longitudinal Dialogues: Which Knowledge Representation Helps?</a> (Mousavi et al., 2023) </li>\n <li> <a href=\"https://openreview.net/forum?id=XTHfNGI3zT\">Quantifying the Plausibility of Context Reliance in Neural Machine Translation</a> (Sarti et al., 2023)</li>\n <li> <a href=\"https://aclanthology.org/2023.emnlp-main.243/\">A Tale of Pronouns: Interpretability Informs Gender Bias Mitigation for Fairer Instruction-Tuned Machine Translation</a> (Attanasio et al., 2023)</li>\n <li> <a href=\"https://arxiv.org/abs/2310.09820\">Assessing the Reliability of Large Language Model Knowledge</a> (Wang et al., 2023)</li>\n <li> <a href=\"https://aclanthology.org/2023.conll-1.18/\">Attribution and Alignment: Effects of Local Context Repetition on Utterance Production and Comprehension in Dialogue</a> (Molnar et al., 2023)</li>\n </ol>\n\n</details>\n\n<details>\n <summary><b>2024</b></summary>\n <ol>\n <li><a href=\"https://arxiv.org/abs/2401.12576\">LLMCheckup: Conversational Examination of Large Language Models via Interpretability Tools</a> (Wang et al., 2024)</li>\n <li><a href=\"https://arxiv.org/abs/2402.00794\">ReAGent: A Model-agnostic Feature Attribution Method for Generative Language Models</a> (Zhao et al., 2024)</li>\n <li><a href=\"https://arxiv.org/abs/2404.02421\">Revisiting subword tokenization: A case study on affixal negation in large language models</a> (Truong et al., 2024)</li>\n </ol>\n\n</details>\n",
"bugtrack_url": null,
"license": "Apache License Version 2.0, January 2004 http://www.apache.org/licenses/ TERMS AND CONDITIONS FOR USE, REPRODUCTION, AND DISTRIBUTION 1. Definitions. \"License\" shall mean the terms and conditions for use, reproduction, and distribution as defined by Sections 1 through 9 of this document. \"Licensor\" shall mean the copyright owner or entity authorized by the copyright owner that is granting the License. \"Legal Entity\" shall mean the union of the acting entity and all other entities that control, are controlled by, or are under common control with that entity. For the purposes of this definition, \"control\" means (i) the power, direct or indirect, to cause the direction or management of such entity, whether by contract or otherwise, or (ii) ownership of fifty percent (50%) or more of the outstanding shares, or (iii) beneficial ownership of such entity. \"You\" (or \"Your\") shall mean an individual or Legal Entity exercising permissions granted by this License. \"Source\" form shall mean the preferred form for making modifications, including but not limited to software source code, documentation source, and configuration files. \"Object\" form shall mean any form resulting from mechanical transformation or translation of a Source form, including but not limited to compiled object code, generated documentation, and conversions to other media types. \"Work\" shall mean the work of authorship, whether in Source or Object form, made available under the License, as indicated by a copyright notice that is included in or attached to the work (an example is provided in the Appendix below). \"Derivative Works\" shall mean any work, whether in Source or Object form, that is based on (or derived from) the Work and for which the editorial revisions, annotations, elaborations, or other modifications represent, as a whole, an original work of authorship. For the purposes of this License, Derivative Works shall not include works that remain separable from, or merely link (or bind by name) to the interfaces of, the Work and Derivative Works thereof. \"Contribution\" shall mean any work of authorship, including the original version of the Work and any modifications or additions to that Work or Derivative Works thereof, that is intentionally submitted to Licensor for inclusion in the Work by the copyright owner or by an individual or Legal Entity authorized to submit on behalf of the copyright owner. For the purposes of this definition, \"submitted\" means any form of electronic, verbal, or written communication sent to the Licensor or its representatives, including but not limited to communication on electronic mailing lists, source code control systems, and issue tracking systems that are managed by, or on behalf of, the Licensor for the purpose of discussing and improving the Work, but excluding communication that is conspicuously marked or otherwise designated in writing by the copyright owner as \"Not a Contribution.\" \"Contributor\" shall mean Licensor and any individual or Legal Entity on behalf of whom a Contribution has been received by Licensor and subsequently incorporated within the Work. 2. Grant of Copyright License. Subject to the terms and conditions of this License, each Contributor hereby grants to You a perpetual, worldwide, non-exclusive, no-charge, royalty-free, irrevocable copyright license to reproduce, prepare Derivative Works of, publicly display, publicly perform, sublicense, and distribute the Work and such Derivative Works in Source or Object form. 3. Grant of Patent License. Subject to the terms and conditions of this License, each Contributor hereby grants to You a perpetual, worldwide, non-exclusive, no-charge, royalty-free, irrevocable (except as stated in this section) patent license to make, have made, use, offer to sell, sell, import, and otherwise transfer the Work, where such license applies only to those patent claims licensable by such Contributor that are necessarily infringed by their Contribution(s) alone or by combination of their Contribution(s) with the Work to which such Contribution(s) was submitted. If You institute patent litigation against any entity (including a cross-claim or counterclaim in a lawsuit) alleging that the Work or a Contribution incorporated within the Work constitutes direct or contributory patent infringement, then any patent licenses granted to You under this License for that Work shall terminate as of the date such litigation is filed. 4. Redistribution. You may reproduce and distribute copies of the Work or Derivative Works thereof in any medium, with or without modifications, and in Source or Object form, provided that You meet the following conditions: 1. You must give any other recipients of the Work or Derivative Works a copy of this License; and 2. You must cause any modified files to carry prominent notices stating that You changed the files; and 3. You must retain, in the Source form of any Derivative Works that You distribute, all copyright, patent, trademark, and attribution notices from the Source form of the Work, excluding those notices that do not pertain to any part of the Derivative Works; and 4. If the Work includes a \"NOTICE\" text file as part of its distribution, then any Derivative Works that You distribute must include a readable copy of the attribution notices contained within such NOTICE file, excluding those notices that do not pertain to any part of the Derivative Works, in at least one of the following places: within a NOTICE text file distributed as part of the Derivative Works; within the Source form or documentation, if provided along with the Derivative Works; or, within a display generated by the Derivative Works, if and wherever such third-party notices normally appear. The contents of the NOTICE file are for informational purposes only and do not modify the License. You may add Your own attribution notices within Derivative Works that You distribute, alongside or as an addendum to the NOTICE text from the Work, provided that such additional attribution notices cannot be construed as modifying the License. You may add Your own copyright statement to Your modifications and may provide additional or different license terms and conditions for use, reproduction, or distribution of Your modifications, or for any such Derivative Works as a whole, provided Your use, reproduction, and distribution of the Work otherwise complies with the conditions stated in this License. 5. Submission of Contributions. Unless You explicitly state otherwise, any Contribution intentionally submitted for inclusion in the Work by You to the Licensor shall be under the terms and conditions of this License, without any additional terms or conditions. Notwithstanding the above, nothing herein shall supersede or modify the terms of any separate license agreement you may have executed with Licensor regarding such Contributions. 6. Trademarks. This License does not grant permission to use the trade names, trademarks, service marks, or product names of the Licensor, except as required for reasonable and customary use in describing the origin of the Work and reproducing the content of the NOTICE file. 7. Disclaimer of Warranty. Unless required by applicable law or agreed to in writing, Licensor provides the Work (and each Contributor provides its Contributions) on an \"AS IS\" BASIS, WITHOUT WARRANTIES OR CONDITIONS OF ANY KIND, either express or implied, including, without limitation, any warranties or conditions of TITLE, NON-INFRINGEMENT, MERCHANTABILITY, or FITNESS FOR A PARTICULAR PURPOSE. You are solely responsible for determining the appropriateness of using or redistributing the Work and assume any risks associated with Your exercise of permissions under this License. 8. Limitation of Liability. In no event and under no legal theory, whether in tort (including negligence), contract, or otherwise, unless required by applicable law (such as deliberate and grossly negligent acts) or agreed to in writing, shall any Contributor be liable to You for damages, including any direct, indirect, special, incidental, or consequential damages of any character arising as a result of this License or out of the use or inability to use the Work (including but not limited to damages for loss of goodwill, work stoppage, computer failure or malfunction, or any and all other commercial damages or losses), even if such Contributor has been advised of the possibility of such damages. 9. Accepting Warranty or Additional Liability. While redistributing the Work or Derivative Works thereof, You may choose to offer, and charge a fee for, acceptance of support, warranty, indemnity, or other liability obligations and/or rights consistent with this License. However, in accepting such obligations, You may act only on Your own behalf and on Your sole responsibility, not on behalf of any other Contributor, and only if You agree to indemnify, defend, and hold each Contributor harmless for any liability incurred by, or claims asserted against, such Contributor by reason of your accepting any such warranty or additional liability. END OF TERMS AND CONDITIONS APPENDIX: How to apply the Apache License to your work To apply the Apache License to your work, attach the following boilerplate notice, with the fields enclosed by brackets \"[]\" replaced with your own identifying information. (Don't include the brackets!) The text should be enclosed in the appropriate comment syntax for the file format. We also recommend that a file or class name and description of purpose be included on the same \"printed page\" as the copyright notice for easier identification within third-party archives. Copyright 2021 The Inseq Team Licensed under the Apache License, Version 2.0 (the \"License\"); you may not use this file except in compliance with the License. You may obtain a copy of the License at http://www.apache.org/licenses/LICENSE-2.0 Unless required by applicable law or agreed to in writing, software distributed under the License is distributed on an \"AS IS\" BASIS, WITHOUT WARRANTIES OR CONDITIONS OF ANY KIND, either express or implied. See the License for the specific language governing permissions and limitations under the License.",
"summary": "Interpretability for Sequence Generation Models \ud83d\udd0d",
"version": "0.6.0",
"project_urls": {
"changelog": "https://github.com/inseq-team/inseq/blob/main/CHANGELOG.md",
"documentation": "https://inseq.org",
"homepage": "https://github.com/inseq-team/inseq",
"repository": "https://github.com/inseq-team/inseq"
},
"split_keywords": [
"generative ai",
" transformers",
" natural language processing",
" xai",
" explainable ai",
" interpretability",
" feature attribution",
" machine translation"
],
"urls": [
{
"comment_text": "",
"digests": {
"blake2b_256": "7b9da326f71920949e92be538ad86aad03a37e41c32a7c1f7bf61706f8d5afbb",
"md5": "1b3abe999e292b7e57bc502dcf073f06",
"sha256": "4c80529ad6b255242ce1257b137c923294c76629bcc588bb24beeb2d752d3e1a"
},
"downloads": -1,
"filename": "inseq-0.6.0-py3-none-any.whl",
"has_sig": false,
"md5_digest": "1b3abe999e292b7e57bc502dcf073f06",
"packagetype": "bdist_wheel",
"python_version": "py3",
"requires_python": ">=3.9",
"size": 186602,
"upload_time": "2024-04-13T13:37:33",
"upload_time_iso_8601": "2024-04-13T13:37:33.927980Z",
"url": "https://files.pythonhosted.org/packages/7b/9d/a326f71920949e92be538ad86aad03a37e41c32a7c1f7bf61706f8d5afbb/inseq-0.6.0-py3-none-any.whl",
"yanked": false,
"yanked_reason": null
},
{
"comment_text": "",
"digests": {
"blake2b_256": "bf83499413b48bcf0fab5213d253b1203702c8a8fa64f5ba24f10cc186942ad3",
"md5": "1f63709bf4ed64f0ab7fb3acf5a26176",
"sha256": "836f2b69cf2528d3a2c8061c7e8ee8c42b81701b4385993a6e912a59d805021d"
},
"downloads": -1,
"filename": "inseq-0.6.0.tar.gz",
"has_sig": false,
"md5_digest": "1f63709bf4ed64f0ab7fb3acf5a26176",
"packagetype": "sdist",
"python_version": "source",
"requires_python": ">=3.9",
"size": 180323,
"upload_time": "2024-04-13T13:37:37",
"upload_time_iso_8601": "2024-04-13T13:37:37.795537Z",
"url": "https://files.pythonhosted.org/packages/bf/83/499413b48bcf0fab5213d253b1203702c8a8fa64f5ba24f10cc186942ad3/inseq-0.6.0.tar.gz",
"yanked": false,
"yanked_reason": null
}
],
"upload_time": "2024-04-13 13:37:37",
"github": true,
"gitlab": false,
"bitbucket": false,
"codeberg": false,
"github_user": "inseq-team",
"github_project": "inseq",
"travis_ci": false,
"coveralls": false,
"github_actions": true,
"requirements": [
{
"name": "captum",
"specs": [
[
"==",
"0.7.0"
]
]
},
{
"name": "certifi",
"specs": [
[
"==",
"2024.2.2"
]
]
},
{
"name": "charset-normalizer",
"specs": [
[
"==",
"3.3.2"
]
]
},
{
"name": "contourpy",
"specs": [
[
"==",
"1.2.0"
]
]
},
{
"name": "cycler",
"specs": [
[
"==",
"0.12.1"
]
]
},
{
"name": "filelock",
"specs": [
[
"==",
"3.13.1"
]
]
},
{
"name": "fonttools",
"specs": [
[
"==",
"4.48.1"
]
]
},
{
"name": "fsspec",
"specs": [
[
"==",
"2023.10.0"
]
]
},
{
"name": "huggingface-hub",
"specs": [
[
"==",
"0.20.3"
]
]
},
{
"name": "idna",
"specs": [
[
"==",
"3.6"
]
]
},
{
"name": "jaxtyping",
"specs": [
[
"==",
"0.2.25"
]
]
},
{
"name": "jinja2",
"specs": [
[
"==",
"3.1.3"
]
]
},
{
"name": "kiwisolver",
"specs": [
[
"==",
"1.4.5"
]
]
},
{
"name": "markdown-it-py",
"specs": [
[
"==",
"3.0.0"
]
]
},
{
"name": "markupsafe",
"specs": [
[
"==",
"2.1.5"
]
]
},
{
"name": "matplotlib",
"specs": [
[
"==",
"3.8.2"
]
]
},
{
"name": "mdurl",
"specs": [
[
"==",
"0.1.2"
]
]
},
{
"name": "mpmath",
"specs": [
[
"==",
"1.3.0"
]
]
},
{
"name": "networkx",
"specs": [
[
"==",
"3.2.1"
]
]
},
{
"name": "numpy",
"specs": [
[
"==",
"1.26.4"
]
]
},
{
"name": "packaging",
"specs": [
[
"==",
"23.2"
]
]
},
{
"name": "pillow",
"specs": [
[
"==",
"10.2.0"
]
]
},
{
"name": "protobuf",
"specs": [
[
"==",
"4.25.2"
]
]
},
{
"name": "pygments",
"specs": [
[
"==",
"2.17.2"
]
]
},
{
"name": "pyparsing",
"specs": [
[
"==",
"3.1.1"
]
]
},
{
"name": "python-dateutil",
"specs": [
[
"==",
"2.8.2"
]
]
},
{
"name": "pyyaml",
"specs": [
[
"==",
"6.0.1"
]
]
},
{
"name": "regex",
"specs": [
[
"==",
"2023.12.25"
]
]
},
{
"name": "requests",
"specs": [
[
"==",
"2.31.0"
]
]
},
{
"name": "rich",
"specs": [
[
"==",
"13.7.0"
]
]
},
{
"name": "safetensors",
"specs": [
[
"==",
"0.4.2"
]
]
},
{
"name": "sentencepiece",
"specs": [
[
"==",
"0.1.99"
]
]
},
{
"name": "six",
"specs": [
[
"==",
"1.16.0"
]
]
},
{
"name": "sympy",
"specs": [
[
"==",
"1.12"
]
]
},
{
"name": "tokenizers",
"specs": [
[
"==",
"0.15.2"
]
]
},
{
"name": "torch",
"specs": [
[
"==",
"2.2.0"
]
]
},
{
"name": "tqdm",
"specs": [
[
"==",
"4.66.2"
]
]
},
{
"name": "transformers",
"specs": [
[
"==",
"4.38.1"
]
]
},
{
"name": "typeguard",
"specs": [
[
"==",
"2.13.3"
]
]
},
{
"name": "typing-extensions",
"specs": [
[
"==",
"4.9.0"
]
]
},
{
"name": "urllib3",
"specs": [
[
"==",
"2.2.0"
]
]
}
],
"lcname": "inseq"
}