<h1 align="center"><b>NoiseGrad and FusionGrad</b></h1>
<h3 align="center"><b>NoiseGrad: enhancing explanations by introducing stochasticity to model weights</b></h3>
<p align="center">
<i>Pytorch implementation</i>
</p>
--------------
Pytorch implementation for **"NoiseGrad: enhancing explanations by introducing stochasticity to model weights"**. The paper introduces two novel methods `NoiseGrad` and `FusionGrad` which both improves attribution-based explanations by introducing stochasticity to the model parameters. See arXiv preprint: https://arxiv.org/abs/2106.10185.
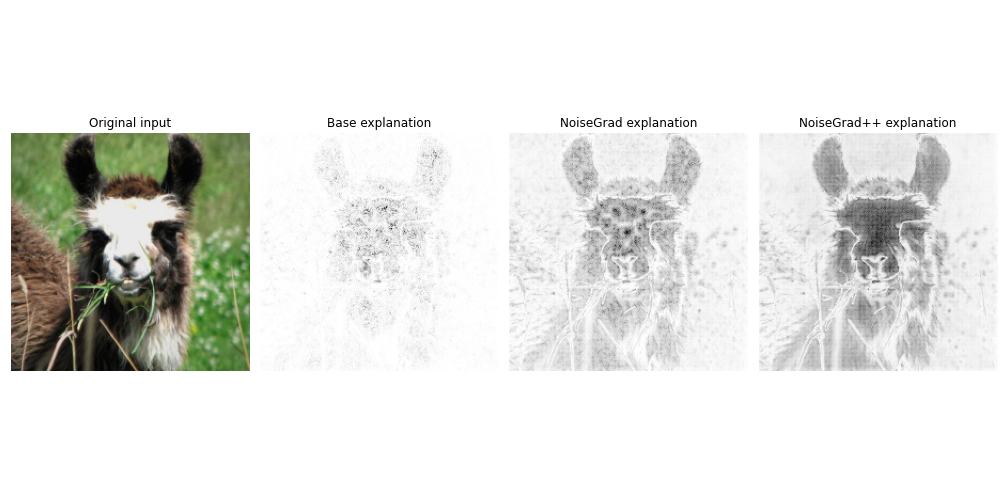
## Cite this paper
To cite this paper use following Bibtex annotation:
@misc{bykov2021noisegrad,
title={NoiseGrad: enhancing explanations by introducing stochasticity to model weights},
author={Kirill Bykov and Anna Hedström and Shinichi Nakajima and Marina M. -C. Höhne},
year={2021},
eprint={2106.10185},
archivePrefix={arXiv},
primaryClass={cs.LG}}
## Installation
```shell
pip install noisegrad
```
All experiments were conducted with Python 3.6.9.
## Minimal Example, for more detailed ones, please refer to `examples/`
```python
from noisegrad import NoiseGrad, NoiseGradConfig, NoiseGradPlusPlus, NoiseGradPlusPlusConfig
from noisegrad.explainers import intgrad_explainer
# Initialize NoiseGrad: enhance any explanation function!
noisegrad = NoiseGrad(NoiseGradConfig(n=5))
# Initialize NoiseGrad++: enhance any explanation function!
noisegradp = NoiseGradPlusPlus(NoiseGradPlusPlusConfig(n=5, m=5))
# Get baseline explanation.
expl_base = intgrad_explainer(model, x, y)
# Get NoiseGrad explanation.
expl_ng = noisegrad.enhance_explanation(model, x, y, intgrad_explainer)
# Get NoiseGrad++ explanation.
expl_ngp = noisegradp.enhance_explanation(model, x, y, intgrad_explainer)
```
Raw data
{
"_id": null,
"home_page": "",
"name": "noisegrad",
"maintainer": "",
"docs_url": null,
"requires_python": ">=3.8",
"maintainer_email": "Kirill Bykov <kirill.bykov@campus.tu-berlin.de>, Anna Hedstr\u00f6m <anna.hedstroem@tu-berlin.de>, Artem Sereda <artem.sereda@campus.tu-berlin.de>",
"keywords": "explainable ai,xai,machine learning,deep learning",
"author": "",
"author_email": "Kirill Bykov <kirill.bykov@campus.tu-berlin.de>, Anna Hedstr\u00f6m <anna.hedstroem@tu-berlin.de>, Shinichi Nakajima <nakajima@tu-berlin.de>, \"Marina M.-C. H\u00f6hne\" <marina.hoehne@tu-berlin.de>, Artem Sereda <artem.sereda@campus.tu-berlin.de>",
"download_url": "https://files.pythonhosted.org/packages/93/70/e7a96233f95b61fad6da04825e19e7b66caf383f258badc71cb1c05a84f4/noisegrad-0.0.3.tar.gz",
"platform": null,
"description": "<h1 align=\"center\"><b>NoiseGrad and FusionGrad</b></h1>\n<h3 align=\"center\"><b>NoiseGrad: enhancing explanations by introducing stochasticity to model weights</b></h3>\n<p align=\"center\">\n <i>Pytorch implementation</i>\n</p>\n\n--------------\n\nPytorch implementation for **\"NoiseGrad: enhancing explanations by introducing stochasticity to model weights\"**. The paper introduces two novel methods `NoiseGrad` and `FusionGrad` which both improves attribution-based explanations by introducing stochasticity to the model parameters. See arXiv preprint: https://arxiv.org/abs/2106.10185.\n\n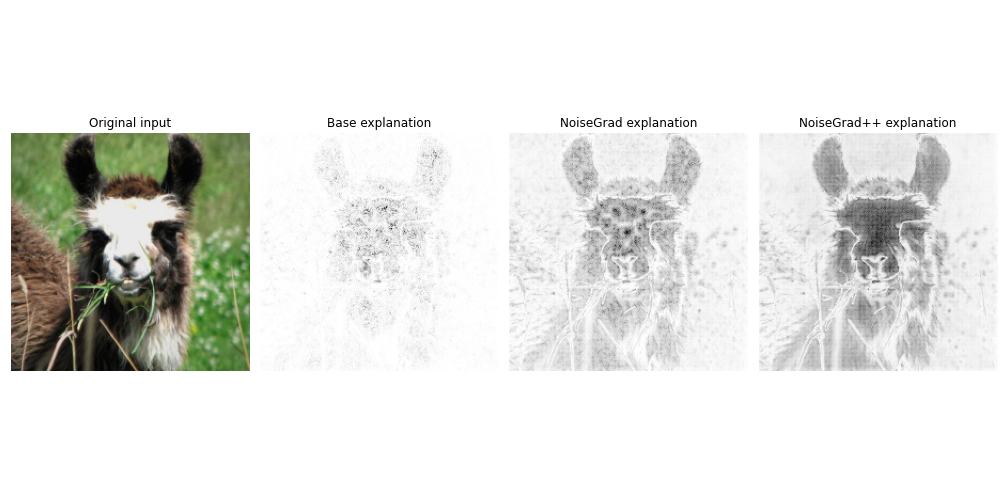\n\n## Cite this paper\n\nTo cite this paper use following Bibtex annotation:\n\n\t@misc{bykov2021noisegrad,\n\t title={NoiseGrad: enhancing explanations by introducing stochasticity to model weights},\n\t author={Kirill Bykov and Anna Hedstr\u00f6m and Shinichi Nakajima and Marina M. -C. H\u00f6hne},\n\t year={2021},\n\t eprint={2106.10185},\n\t archivePrefix={arXiv},\n\t primaryClass={cs.LG}}\n\n## Installation\n\n```shell\npip install noisegrad\n```\n\nAll experiments were conducted with Python 3.6.9.\n\n## Minimal Example, for more detailed ones, please refer to `examples/`\n```python\nfrom noisegrad import NoiseGrad, NoiseGradConfig, NoiseGradPlusPlus, NoiseGradPlusPlusConfig\nfrom noisegrad.explainers import intgrad_explainer\n\n# Initialize NoiseGrad: enhance any explanation function!\nnoisegrad = NoiseGrad(NoiseGradConfig(n=5))\n\n# Initialize NoiseGrad++: enhance any explanation function!\nnoisegradp = NoiseGradPlusPlus(NoiseGradPlusPlusConfig(n=5, m=5))\n\n# Get baseline explanation.\nexpl_base = intgrad_explainer(model, x, y)\n\n# Get NoiseGrad explanation.\nexpl_ng = noisegrad.enhance_explanation(model, x, y, intgrad_explainer)\n\n# Get NoiseGrad++ explanation.\nexpl_ngp = noisegradp.enhance_explanation(model, x, y, intgrad_explainer)\n```\n\n\n\n",
"bugtrack_url": null,
"license": "",
"summary": "A explanation enhancement method.",
"version": "0.0.3",
"split_keywords": [
"explainable ai",
"xai",
"machine learning",
"deep learning"
],
"urls": [
{
"comment_text": "",
"digests": {
"blake2b_256": "efe25b302cf5c574a1a90c8a8fdb47c9c0146768d3d928b1d9c44f4b8159c1c8",
"md5": "69eef3010eed7deee0e5d85b482da87c",
"sha256": "34155b819fac633d8fd35e1f25675043fbaa48bf11a73f5ddf7e2029930edcc9"
},
"downloads": -1,
"filename": "noisegrad-0.0.3-py3-none-any.whl",
"has_sig": false,
"md5_digest": "69eef3010eed7deee0e5d85b482da87c",
"packagetype": "bdist_wheel",
"python_version": "py3",
"requires_python": ">=3.8",
"size": 7173,
"upload_time": "2023-04-09T22:02:53",
"upload_time_iso_8601": "2023-04-09T22:02:53.464680Z",
"url": "https://files.pythonhosted.org/packages/ef/e2/5b302cf5c574a1a90c8a8fdb47c9c0146768d3d928b1d9c44f4b8159c1c8/noisegrad-0.0.3-py3-none-any.whl",
"yanked": false,
"yanked_reason": null
},
{
"comment_text": "",
"digests": {
"blake2b_256": "9370e7a96233f95b61fad6da04825e19e7b66caf383f258badc71cb1c05a84f4",
"md5": "d9402515f66245b141843f13403ec209",
"sha256": "941d6810246f526fae359422700eb0d0e84f85641d0b59389b4db8d7b6869135"
},
"downloads": -1,
"filename": "noisegrad-0.0.3.tar.gz",
"has_sig": false,
"md5_digest": "d9402515f66245b141843f13403ec209",
"packagetype": "sdist",
"python_version": "source",
"requires_python": ">=3.8",
"size": 8252,
"upload_time": "2023-04-09T22:02:54",
"upload_time_iso_8601": "2023-04-09T22:02:54.715821Z",
"url": "https://files.pythonhosted.org/packages/93/70/e7a96233f95b61fad6da04825e19e7b66caf383f258badc71cb1c05a84f4/noisegrad-0.0.3.tar.gz",
"yanked": false,
"yanked_reason": null
}
],
"upload_time": "2023-04-09 22:02:54",
"github": false,
"gitlab": false,
"bitbucket": false,
"lcname": "noisegrad"
}